The Hybrid Trip Destination Prediction Model of Vehicles Based on Autoencoder and High-Order Interaction Features
IEEE TRANSACTIONS ON INTELLIGENT TRANSPORTATION SYSTEMS(2023)
Abstract
Through the intelligent vehicles trip data collection, processing and analysis, so as to predict the vehicles trip destination, this technology can improve the user's driving experience and the city traffic conditions. The vehicles dispatching system can also judge the real-time road conditions according to the prediction results and plan a more reasonable and efficient driving route, which is of great significance to urban traffic planning and urban construction planning. However, the amount of information in the vehicles data is less, which cannot meet the training needs of some artificial intelligence models. In addition, due to communication technology and other issues, there is a certain degree of deviation between the GPS data of the vehicles and the real data. The previous vehicles destination prediction model did not well eliminate the negative impact of this deviation. In addition, the previous model linearly adds the characteristics of each vehicles's trip data, which cannot reflect the complex rules of the vehicles's trip destination. Therefore, to address the above-mentioned drawbacks, we propose a novel vehicle trip destination prediction method named Hybrid Trip Destination Prediction Model of Vehicle Based on Autoencoder and High-Order Interaction Features (HAHIF). The HAHIF model extracts robust hidden features using the autoencoder model and considers the second-order association between them using a factorization machine to improve its superiority and effectiveness. Compared with mainstream benchmarks, the HAHIF model has an MSE value of 0.096, an RMSE value of 0.427, and a MAE value of 0.203 on the public dataset, both of which are the first place, which verifies that the HAHIF model has good predictive ability.
MoreTranslated text
Key words
Trip destination prediction, vehicles trajectory mining, neural networks, autoencoder
AI Read Science
Must-Reading Tree
Example
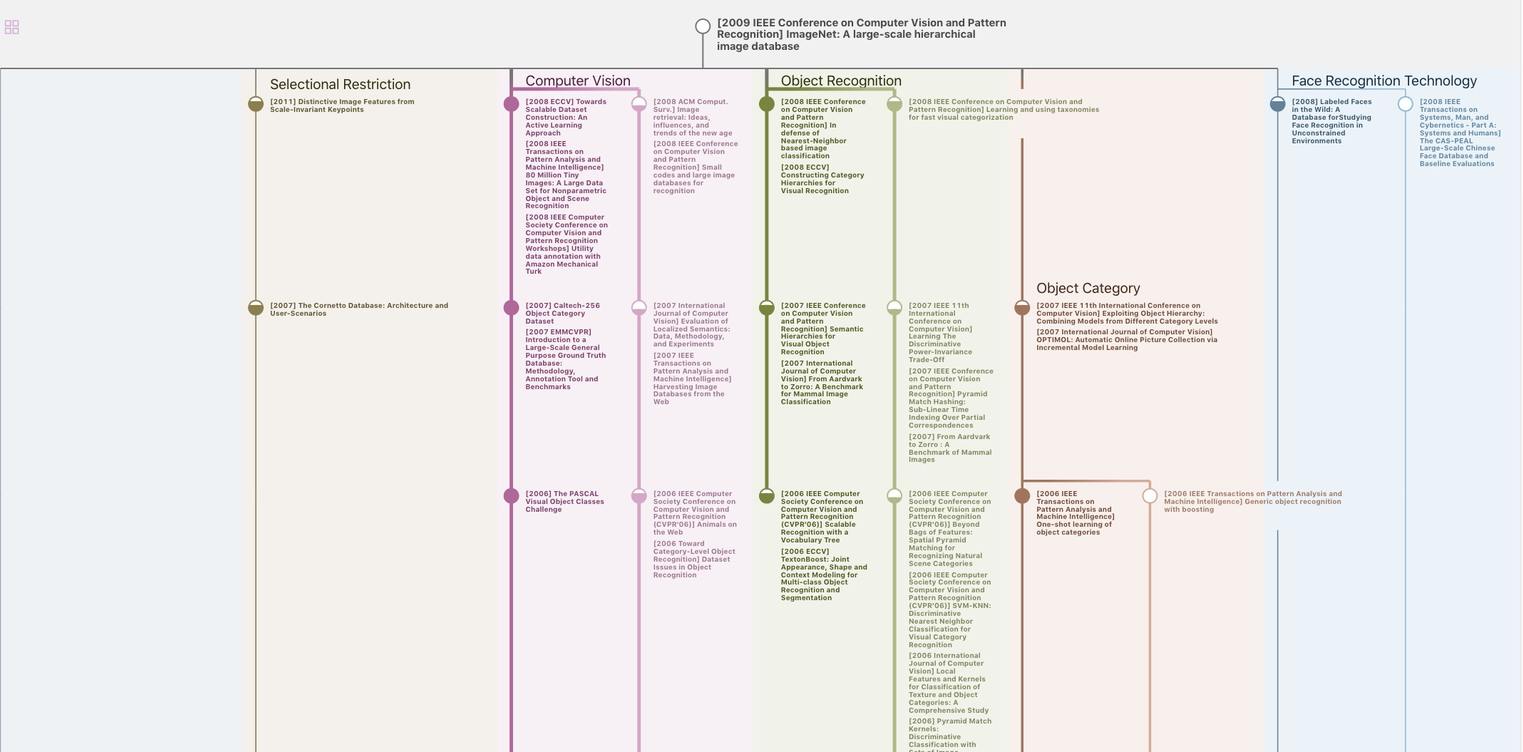
Generate MRT to find the research sequence of this paper
Chat Paper
Summary is being generated by the instructions you defined