Application of Long Short-Term Memory Recurrent Neural Networks for Localisation of Leak Source Using 3D Computational Fluid Dynamics
Process safety and environmental protection/Transactions of the Institution of Chemical Engineers Part B, Process safety and environmental protection/Chemical engineering research and design/Chemical engineering research & design(2022)
Abstract
Gas leaks represent a major concern in industrial sites due to potential human and economical losses. Prompt identification of leak scenarios favours corrective maintenance avoiding the domino effect. In this paper, long short-term memory recurrent neural networks were trained and tested to CH4 leakage source in a chemical process module. We exploit the benefits of varying the temporal length of input variables, and the datasets were obtained employing 3D-CFD simulations. We consider four leak locations, four wind speeds, and eight wind directions, besides the non-leakage scenario for the same wind speeds and directions. The models were trained using different values of timesteps to evaluate the prediction accuracy for unseen data. Results showed progressive improvement of the performance of the models with greater values of timesteps, and good generalisation with test accuracy over 95.3%, indicating the ability of the model to correctly predict the leakage source using easily monitored variables.
MoreTranslated text
Key words
Methane release,Source localization,Process safety,Recurrent neural networks,Long short-term memory
AI Read Science
Must-Reading Tree
Example
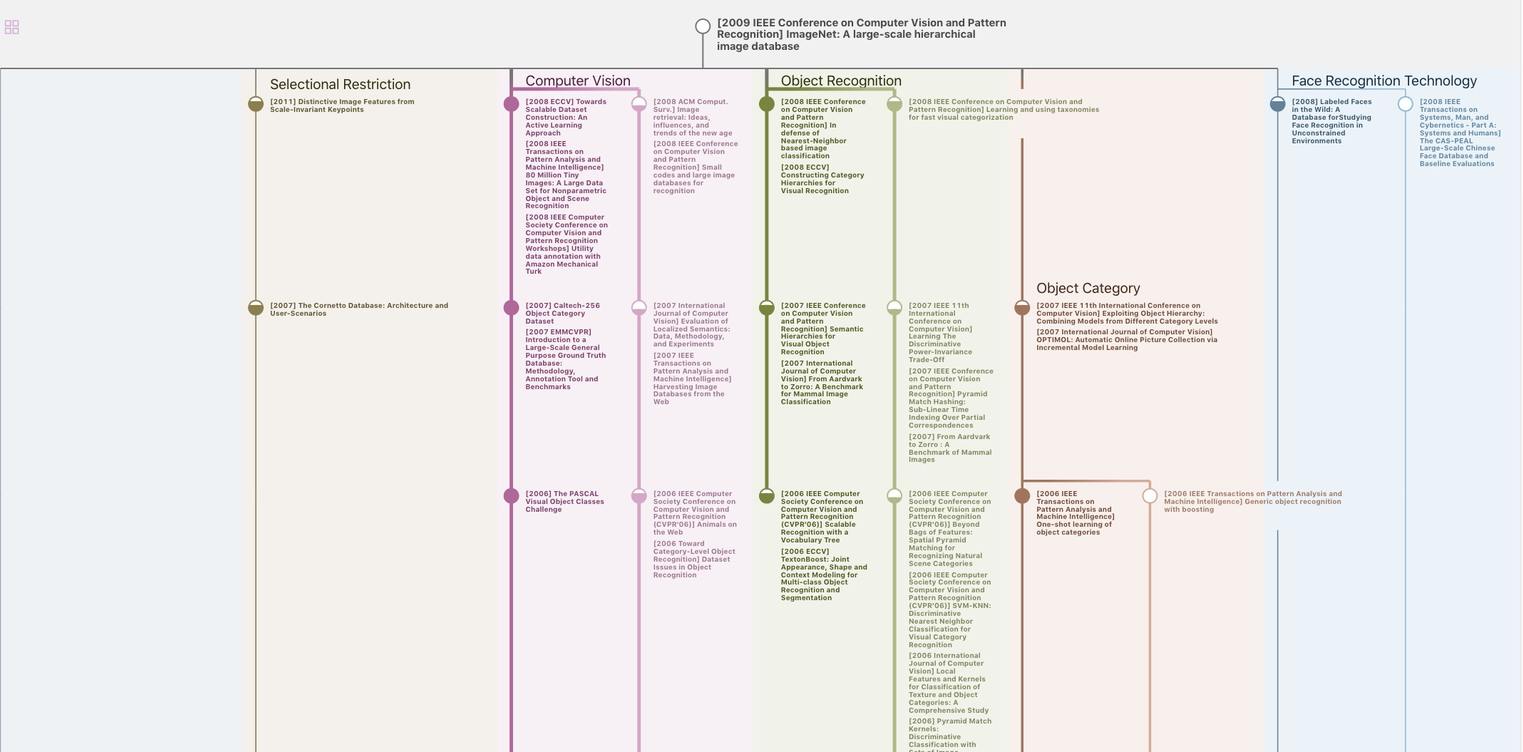
Generate MRT to find the research sequence of this paper
Chat Paper
Summary is being generated by the instructions you defined