PREDAIP: Computational Prediction and Analysis for Anti-inflammatory Peptide via a Hybrid Feature Selection Technique
CURRENT BIOINFORMATICS(2021)
摘要
Background: Anti-Inflammatory Peptides (AIPs) are potent therapeutic agents for in-flammatory and autoimmune disorders due to their high specificity and minimal toxicity under normal conditions. Therefore, it is greatly significant and beneficial to identify AIPs for further discovering novel and efficient AIPs-based therapeutics. Recently, three computational approach -es, which can effectively identify potential AIPs, have been developed based on machine learning algorithms. However, there are several challenges with the existing three predictors. Objective: A novel machine learning algorithm needs to be proposed to improve the AIPs predic-tion accuracy. Methods: This study attempts to improve the recognition of AIPs by employing multiple primary sequence-based feature descriptors and an efficient feature selection strategy. By sorting features through four enhanced minimal redundancy maximal relevance (emRMR) methods, and then at-taching seven different classifiers wrapper methods based on the sequential forward selection algo-rithm (SFS), we proposed a hybrid feature selection technique emRMR-SFS to optimize feature vectors. Furthermore, by evaluating seven classifiers trained with the optimal feature subset, we developed the Extremely Randomized Tree (ERT) based predictor named PREDAIP for identify-ing AIPs. Results: We systematically compared the performance of PREDAIP with the existing tools on in-dependent test dataset. It demonstrates the effectiveness and power of the PREDAIP. Conclusion: The correlation criteria used in emRMR would affect the selection results of the op-timal feature subset at the SFS-wrapper stage, which justifies the necessity for considering differ-ent correlation criteria in emRMR.
更多查看译文
关键词
Machine learning, featre selection, enhanced minimal redundancy maximal relevance, sequential forward selection algorithm, prediction, extremely randomized tree
AI 理解论文
溯源树
样例
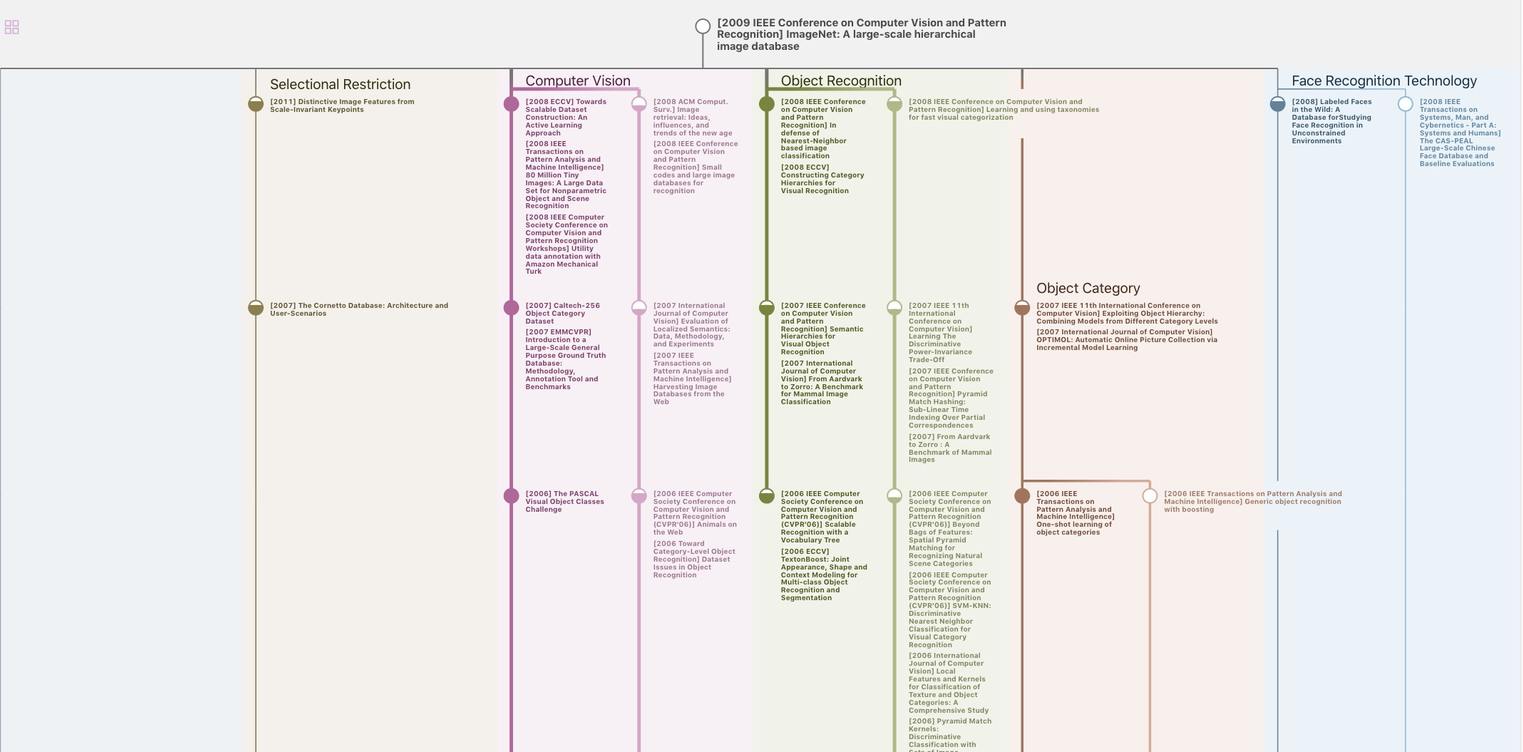
生成溯源树,研究论文发展脉络
Chat Paper
正在生成论文摘要