Modelling short-term variations of differential code bias aiding in extraction of ionospheric observables with sparse kernel learning
ADVANCES IN SPACE RESEARCH(2022)
摘要
As short-term variations existing in differential code bias of receivers (RDCB) will decrease the accuracies of extracting ionospheric observables by carrier-to-code leveling (CCL) method, a modified carrier-to code leveling (MCCL) method had been proposed to estimate RDCB offsets epoch by epoch in retrieval of ionospheric information. However, MCCL method is hampered by efficiency and precision at higher sampling intervals. To address RDCB offsets in higher sampling rate domain, a kernel model in the form of radial basis function (RBF) was chosen to model RDCB offsets produced by MCCL using lower sampling rate data in this paper. Owing to ill-posed problem induced by setting to many kernels, L1 norm regulation was employed to promote the sparsity of the problem and Fast iterative shrinkage thresholding algorithm (FISTA) was adopted to find the sparse solution. Data sets of 350 IGS stations evenly distributed in high-solar and low-solar activity years were chosen to illustrate the general existences of RDCB offsets and their dependence on solar activity. To validate the reliability and efficiency of the proposed method, two stations of them were selected out to construct the spares kernel model. As shown in experimental results, kernel model is of good performance in training data with over 90% sparsity rate and all standard deviations of the errors between original and fitting data are around 0.4 ns. It is a striking finding in terms of efficiency that the proposed method and CCL are much more efficient than MCCL in extracting ionospheric observables by means of fixed equipment. For further validations, generalized triangular series function (GTSF) was constructed using ionospheric observables derived from precise point positioning (PPP), CCL, MCCL and the proposed method. With Center for Orbit Determination in Europe (CODE) Global Ionospheric Map (GIM) regarded as reference, compared with VTEC estimates derived from CCL, those derived from MCCL and the proposed method have improved accuracies ranging from 10% to 20%. Overall, these results indicate that the proposed method has a promising application in retrieval of ionospheric observables in terms of accuracy and efficiency.& nbsp;(c) 2022 Published by Elsevier B.V. on behalf of COSPAR.
更多查看译文
关键词
RDCB offset, Ionospheric observable, Sparse kernel learning, Fast iterative shrinkage thresholding algorithm
AI 理解论文
溯源树
样例
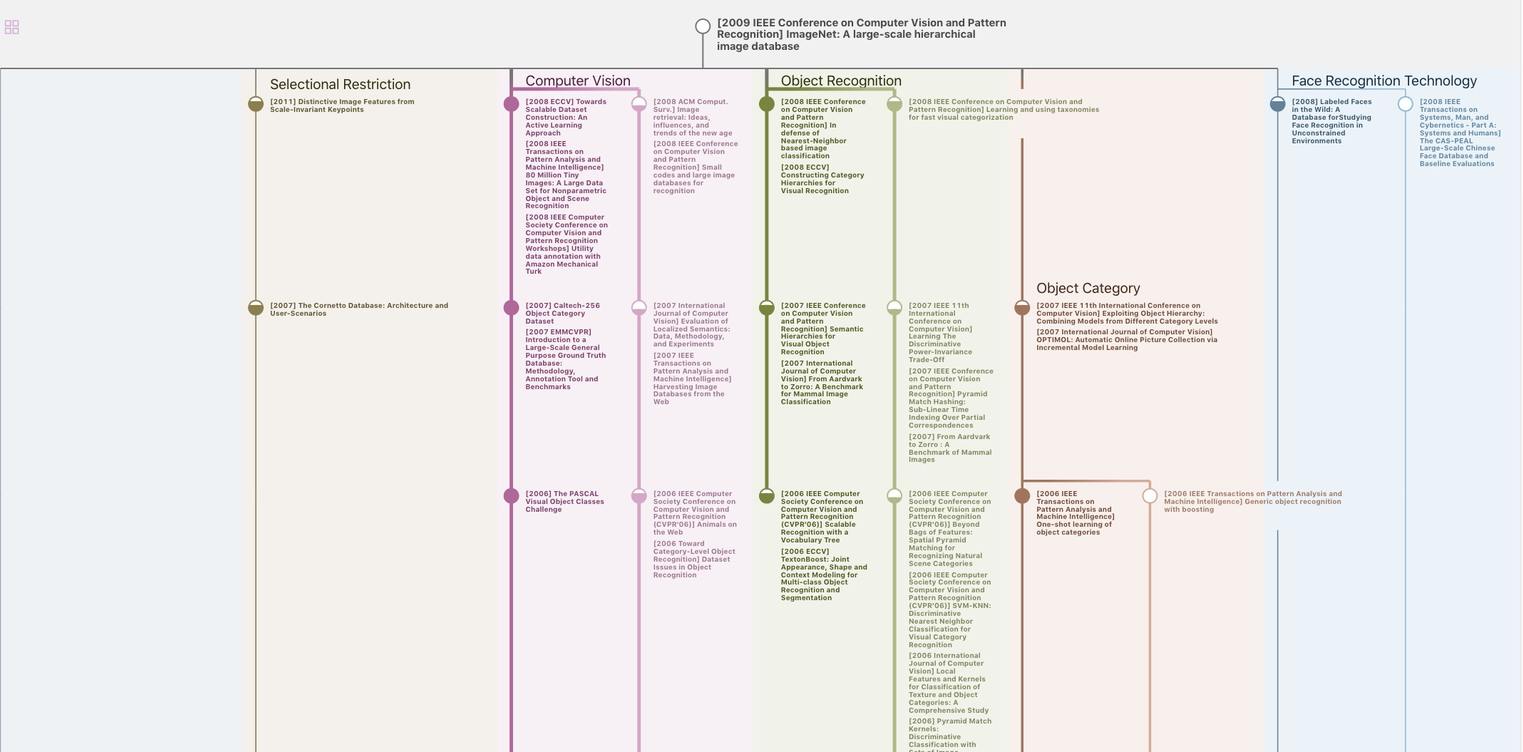
生成溯源树,研究论文发展脉络
Chat Paper
正在生成论文摘要