BEKT: Deep Knowledge Tracing with Bidirectional Encoder Representations from Transformers
29TH INTERNATIONAL CONFERENCE ON COMPUTERS IN EDUCATION (ICCE 2021), VOL II(2021)
摘要
Knowledge tracing is the task of modelling each student's mastery of knowledge components by analysing a student's learning activities trajectories. Each student's knowledge state is modelled based on his or her past learning performance and is an important research area in improving personalized education. In recent years, many researches have focused on deep learning models that aim to solve the knowledge tracing problem. These methods have shown improved performance when compared to traditional knowledge tracing methods such as Bayesian Knowledge Tracing. However, as the input information into the model is a simple representation of the distinction of each student learning logs, the performance of past models are limited and it is hard to measure the relationship between each interaction. To address these problems, we propose the use of a state-of-the-art Bidirectional Encoder Representations from Transformers based model to predict student knowledge state by combining side information such as student historical learning performance. The bidirectional representation can analyse student learning logs in detail and help to understand student learning behaviours. An ablation study is performed to understand the important components of the proposed model and the impact of different input information on model performance. The results of the proposed model evaluation show that it outperforms existing KT methods on a range of datasets.
更多查看译文
关键词
Knowledge tracing, self-attention, BEKT, DKT
AI 理解论文
溯源树
样例
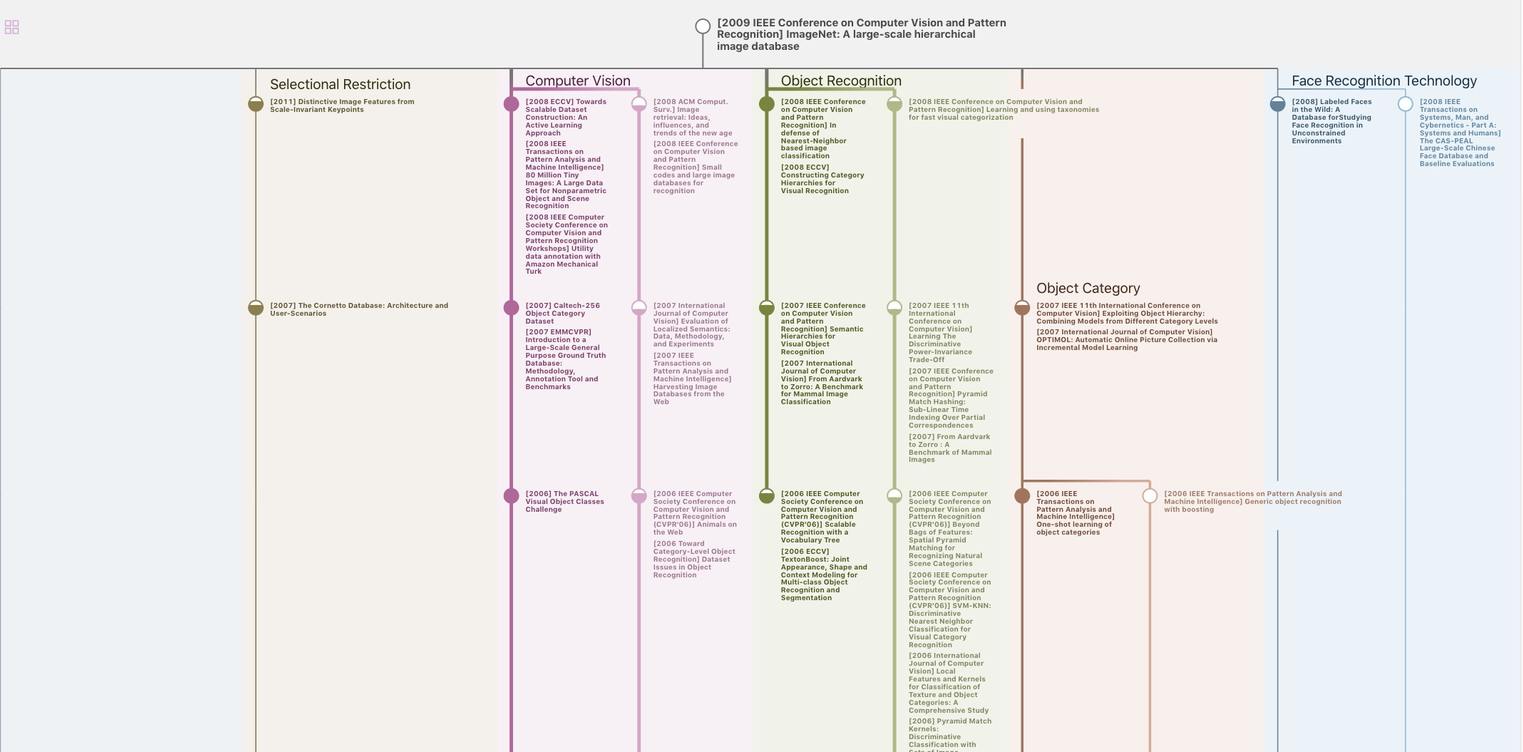
生成溯源树,研究论文发展脉络
Chat Paper
正在生成论文摘要