Histogram lies about distribution shape and Pearson's coefficient of variation lies about relative variability
QUANTITATIVE METHODS FOR PSYCHOLOGY(2022)
摘要
Histograms and Pearson's coefficient of variation are among the most popular summary statistics. Researchers use histograms to judge the shape of quantitative data distribution by visual inspection. The coefficient of variation is taken as an estimator of relative variability of these data. We explore properties of histograms and coefficient of variation by examples in R, thus offering better alternatives: density plots and Eisenhauer's relative dispersion coefficient. Hypothetical examples developed in R are applied to create histograms and density plots, and to compute coefficient of variation and relative dispersion coefficient. These hypothetical examples clearly show that these two traditional approaches are flawed. Histograms do not necessarily reflect the distribution of probabilities and the Pearson's coefficient of variation is not invariant with linear transformations and is not a measure of relative variability, for it is a ratio between a measure of absolute variability (standard deviation) and a measure of central position (mean). Potential alternatives are explained and applied for contrast. With the use of modern computers and R language it is easy to apply density plots, which are able to approximate the theoretical probability distribution. In addition, Eisenhauer's relative dispersion coefficient is suggested as a suitable estimator of relative variability, including sample size correction for lower and upper bounds.
更多查看译文
关键词
Histogram, density plot, relative variability, coefficient of relative dispersion, Pearson's coefficient of variation, standardized range
AI 理解论文
溯源树
样例
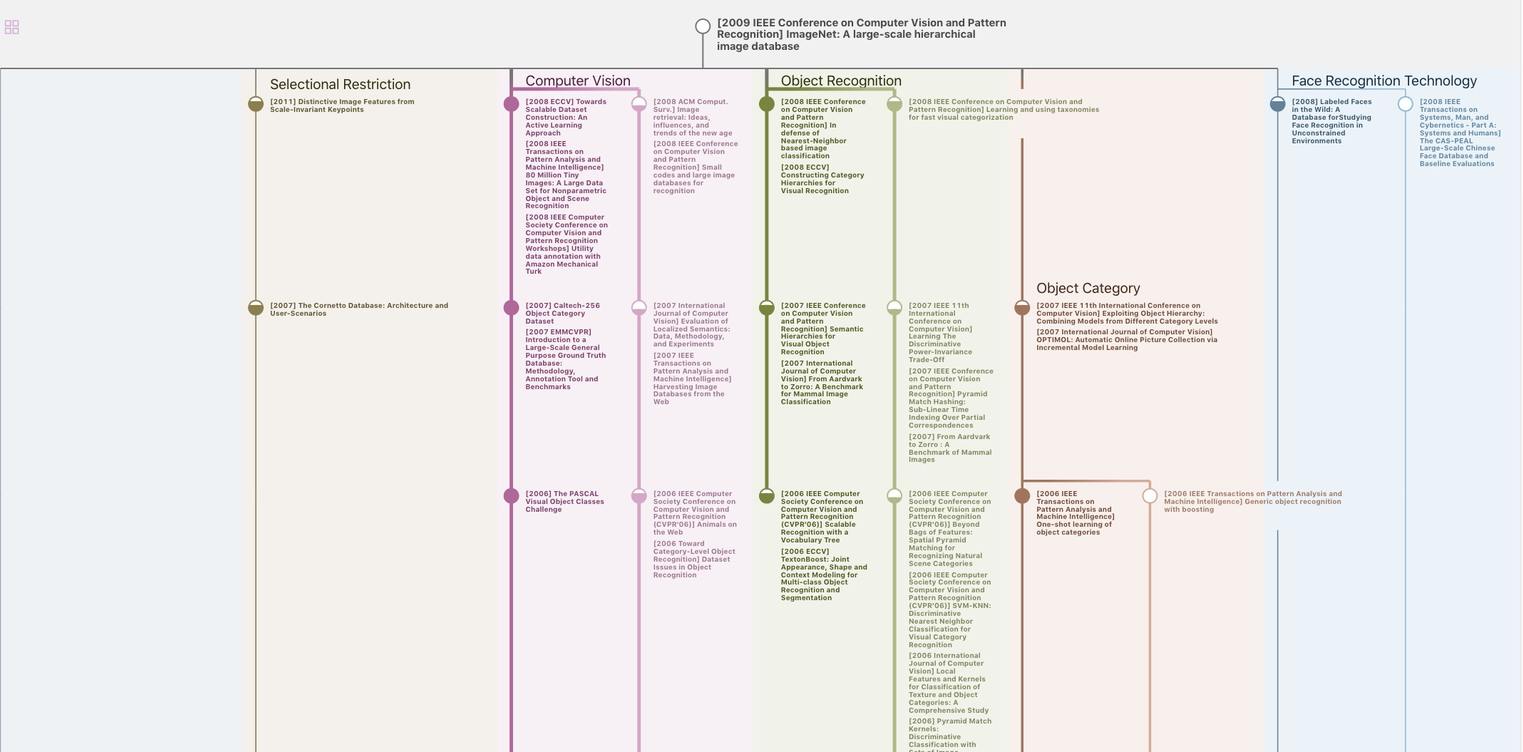
生成溯源树,研究论文发展脉络
Chat Paper
正在生成论文摘要