A Diagnosis Framework for High-reliability Equipment with Small Sample Based on Transfer Learning
COMPLEXITY(2022)
Abstract
Conventional methods for fault diagnosis typically require a substantial amount of training data. However, for equipment with high reliability, it is arduous to form a large-scale well-annotated dataset due to the expense of data acquisition and costly annotation. Besides, the generated data have a large number of redundant features which degraded the performance of models. To overcome this, we proposed a feature transfer scenario that transfers knowledge from similar fields to enhance the accuracy of fault diagnosis with small sample. To reduces the redundant information, data were filtered according to manifold consistency. Then, features were extracted based on CNN and feature transfer was conducted. For adequate fitness, the joint adaptation of conditional distribution and marginal distribution was used between the two domains. Minimum structural risk and MMD of adaptation were two indicators weighted for training the model. To test the efficiency of the model, we built an airborne fuel pump testbed, and contributed a new dataset that contained 15 categories of fault data, which serves as the small sample dataset in this research. Then the proposed model was applied in our experimental data. As a result, the fault diagnosis rate increases by 28.6% through our proposed model, which is more precise than other classical methods. The results of feature visualization further demonstrate that the features are more distinguished through the proposed method. All code and data are accessible on my GitHub.
MoreTranslated text
AI Read Science
Must-Reading Tree
Example
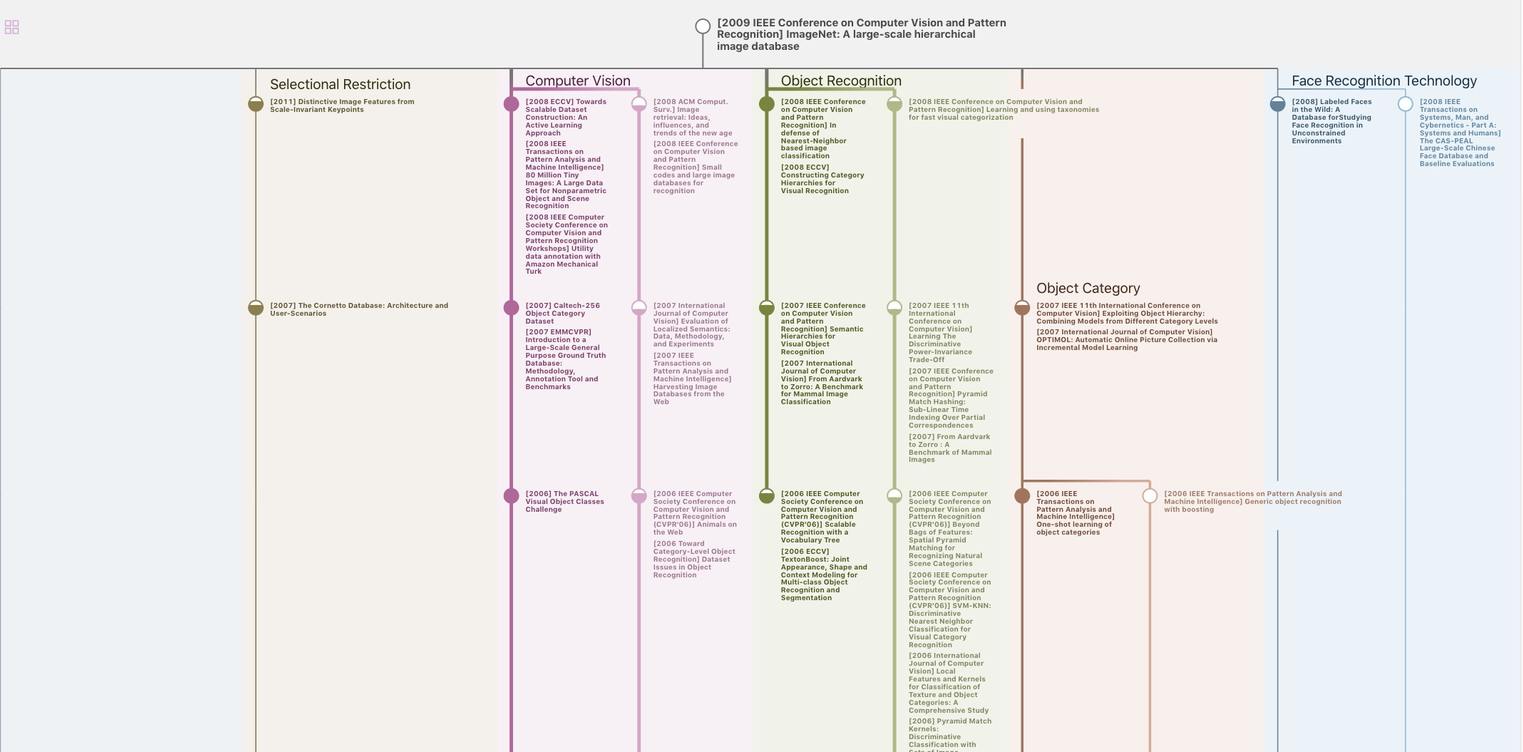
Generate MRT to find the research sequence of this paper
Chat Paper
Summary is being generated by the instructions you defined