RETRACTED: AFLPC: An Asynchronous Federated Learning Privacy-Preserving Computing Model Applied to 5G-V2X (Retracted Article)
SECURITY AND COMMUNICATION NETWORKS(2022)
摘要
Federated learning can effectively protect local data privacy in 5G-V2X environment and ensure data protection in Internet of vehicles environment. The advantages of low delay of 5G network should be better utilized in the vehicle-road cooperative system. But the existing asynchronous federated learning obtains a local model through different node training and completes the update of the global model through the central server. There are problems such as single point of failure, privacy leakage, and deviation of aggregation parameters. In response to the above problems, we proposed a 5G-V2X-oriented asynchronous federated learning privacy-preserving computing model (AFLPC). We used an adaptive differential privacy mechanism to reduce noise while protecting data privacy. A weight-based asynchronous federated learning aggregation update method is proposed to reasonably control the proportion of parameters submitted by users with different training speeds in the aggregation parameters and actively update the aggregation parameters of lagging users, so as to effectively reduce the negative impact on the model caused by the different speed of finding you. Experiments show that the proposed method can effectively ensure the credibility and privacy of asynchronous federated learning in 5G-V2X scenarios and at the same time improve the utility of the model.
更多查看译文
AI 理解论文
溯源树
样例
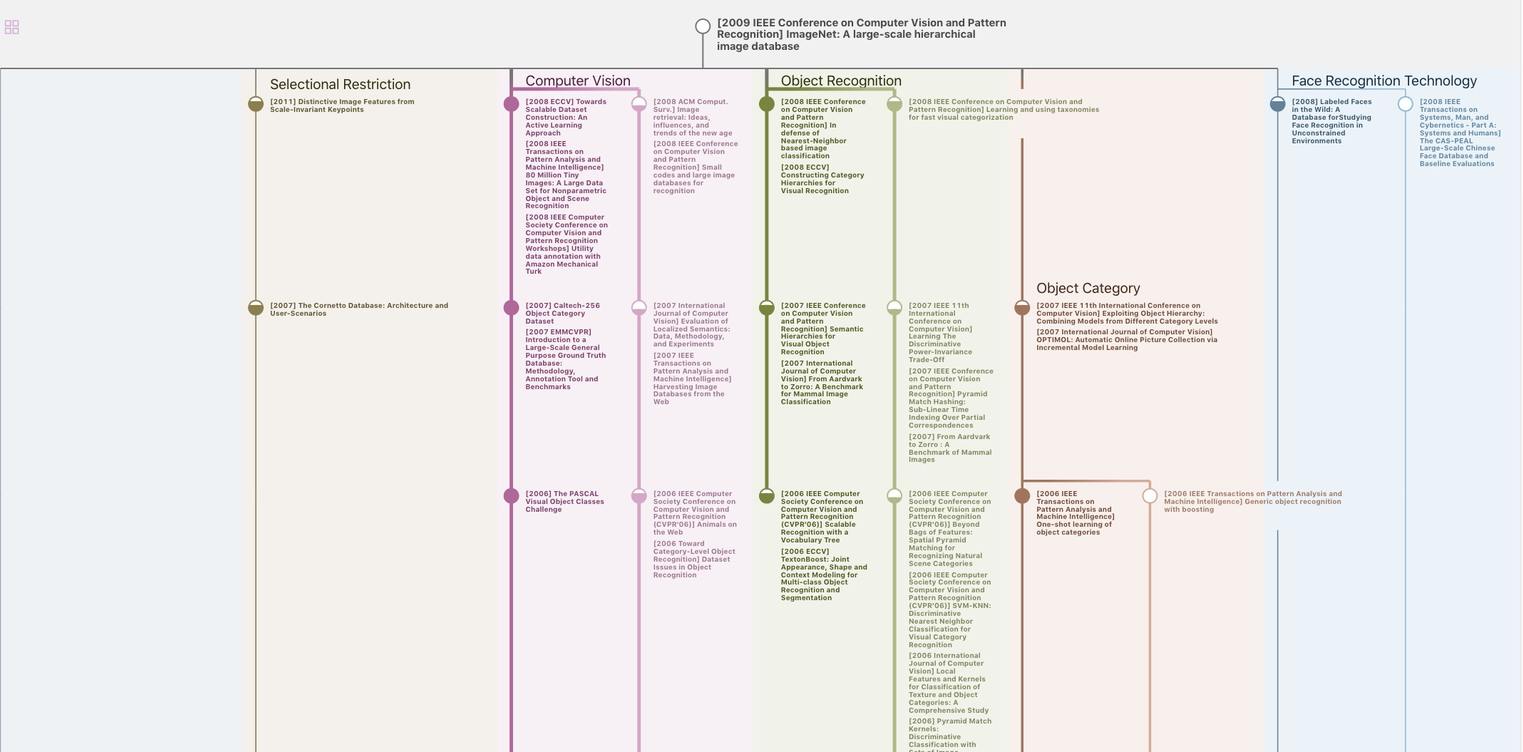
生成溯源树,研究论文发展脉络
Chat Paper
正在生成论文摘要