A Data-Driven Bilevel Model for Estimating Operational Information of a Neighboring Rival's Reservoir in a Competitive Context
IEEE ACCESS(2021)
Abstract
Uncertainties from neighboring rival's reservoirs challenge hydropower companies in participating in competitive markets. Cooperative behaviors are generally impractical due to stakeholders' self-interest and regulatory requirements. Considering this obstacle, this paper proposes a data-driven bilevel model, in a competitive context, to estimate the operational information of the neighboring rival's reservoir, including its historical operating states and operational functions. The proposed bilevel model is an inverse problem of the conventional hydropower scheduling model. The upper-level model is designed to find the most appropriate operational parameters of the estimated reservoir that fit its historical generation volumes. The lower-level model simulates the profit-maxing operation of the estimated reservoir. Since the lower simulating model is nonconvex, an Enhanced Parallel Genetic Algorithm (EPGA) is proposed. It avoids infeasible situations through several strategies and uses multiple CPU threads simultaneously in solving. A case study in China's market demonstrates that the proposed model and solving method can efficiently obtain accurate state series and (near-)optimal operational parameters. More experiments are also taken to validate the parallel design.
MoreTranslated text
Key words
Reservoirs,Hydroelectric power generation,Data models,Stakeholders,Solid modeling,Predictive models,Inverse problems,Bilevel problem,data-driven model,hydropower operation,inverse optimization,parallel genetic algorithm
AI Read Science
Must-Reading Tree
Example
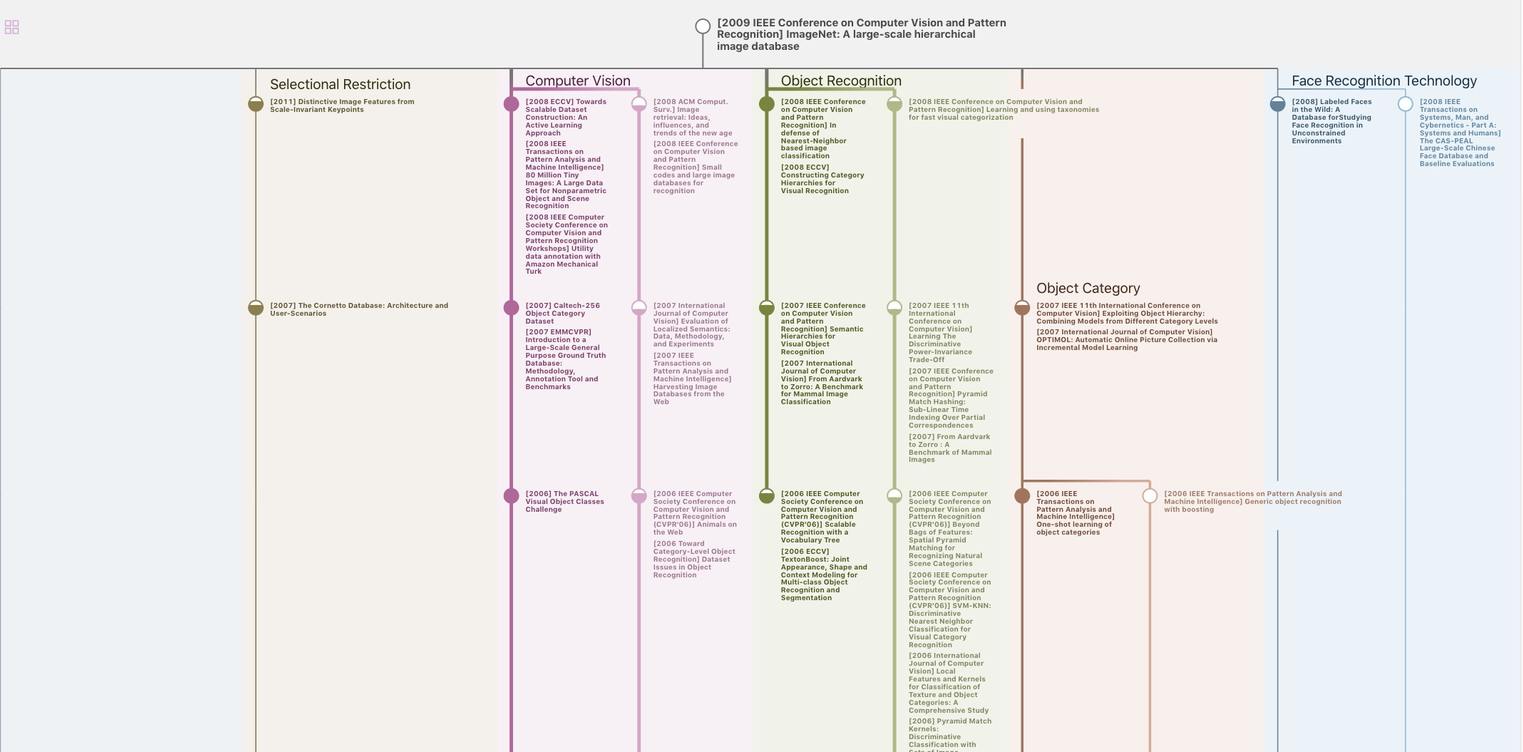
Generate MRT to find the research sequence of this paper
Chat Paper
Summary is being generated by the instructions you defined