Individual-level distance-independent-based growth and yield prediction models for long-term Japanese cedar (Cryptomeria japonica)
CANADIAN JOURNAL OF FOREST RESEARCH(2022)
摘要
Yield prediction has been determined to be vital in sustainable forest management. Recently, research trends have shifted from stand-level to individual-level yield prediction. In this study, we examined the effectiveness of yield prediction models based on a distance-independent approach for Japanese cedar (Cryptomeria japonica (L.f.) D.Don) trees in western Japan. We further examined the accuracy of the models with reference to existing data collected over the long-term. First, we constructed distance-independent height, diameter growth, and survival models. Then, we simulated for approximately 50 years individual tree height, diameter at breast height (DBH), and volume growth using the test data. We then compared the predicted and observed values and calculated root-mean-square error (RMSE) and bias to evaluate the model accuracy. The models were noted to perform well when predicting mean height, DBH, and volume for Japanese cedar trees; in fact, they adequately predicted the diameter distribution. Our results suggest that distance independent models could adequately predict long-term mean values and diameter distribution. However, RMSE and bias indicated that error propagation occurred over longer time spans. Thus, it is effective to conduct actual measurements at some point in the forest development phase and use the measurements as initial values for short-or medium term predictions.
更多查看译文
关键词
yield prediction, distance-independent competition index, simulation, generalized linear mixed model, Japanese cedar
AI 理解论文
溯源树
样例
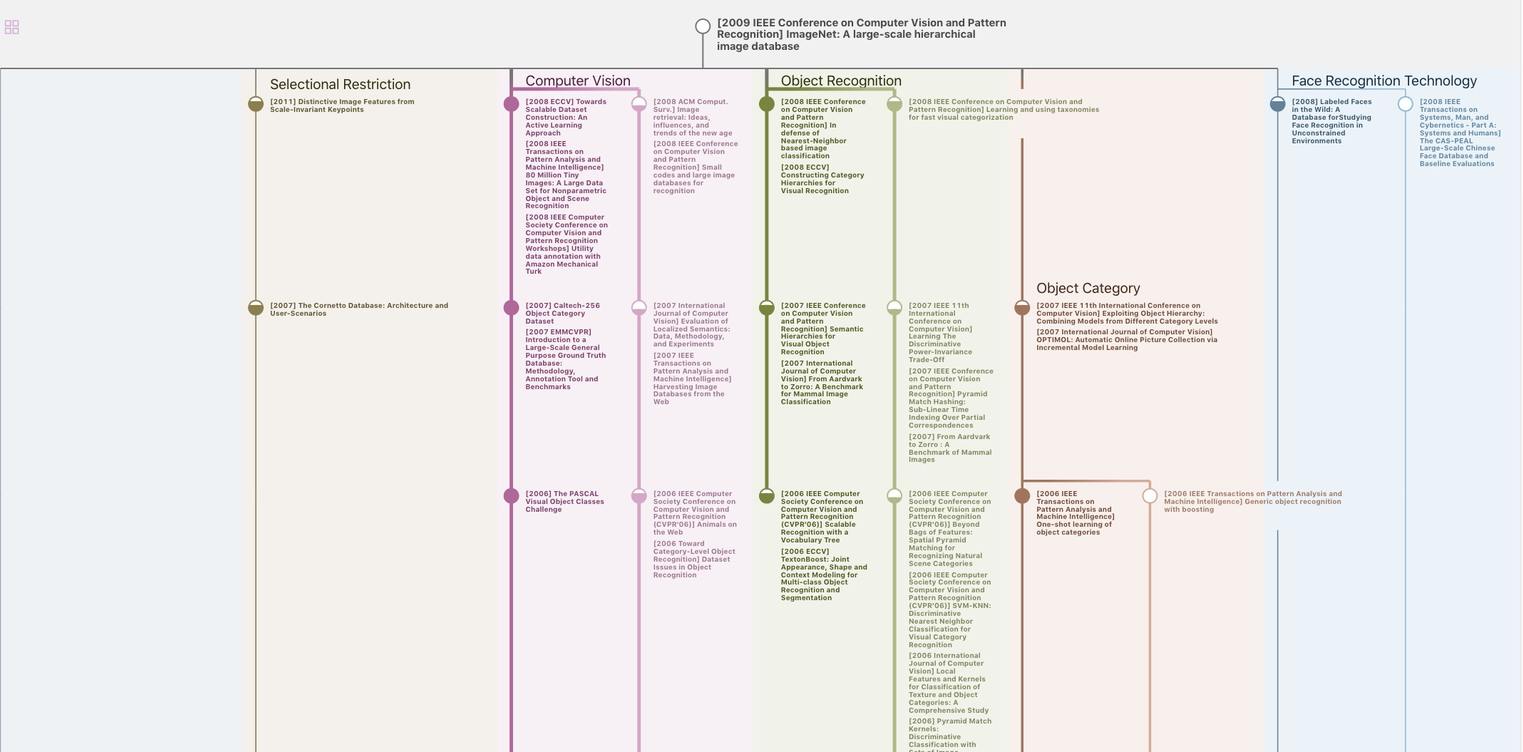
生成溯源树,研究论文发展脉络
Chat Paper
正在生成论文摘要