Machine learning-aided pilot and power allocation in multi-cellular massive MIMO networks
Physical Communication(2022)
摘要
This paper addresses the problem of power allocation (PA) and pilot assignment (PS) in the uplink (UL) of multi-user (MU) massive multiple-input multiple-output (M-MIMO) under pilot contamination (PC) regime. We analyzed the aforementioned problem with different constraints configurations representing practical and adverse conditions such as minimum quality of service (QoS) requirements. A machine learning (ML) approach based on the reward Q-Learning is deployed for implementing the resource allocation (RA) policy. The RA solution obtained with Q-Learning is compared with classical methods available in literature. We have considered different PC scenarios, generated by pilot reuse patterns. Several figures of merit, including spectral and energy efficiencies (SE, EE), bit error rate (BER) and convergence are analyzed considering different system and channel configurations. Extensive numerical simulation results demonstrate the effectiveness and efficiency in terms of performance-complexity trade-off of the proposed sequentially combined PS-PA method in multi-cellular massive MIMO systems.
更多查看译文
关键词
Resource allocation,Massive MIMO,Machine learning,Uplink,Power allocation
AI 理解论文
溯源树
样例
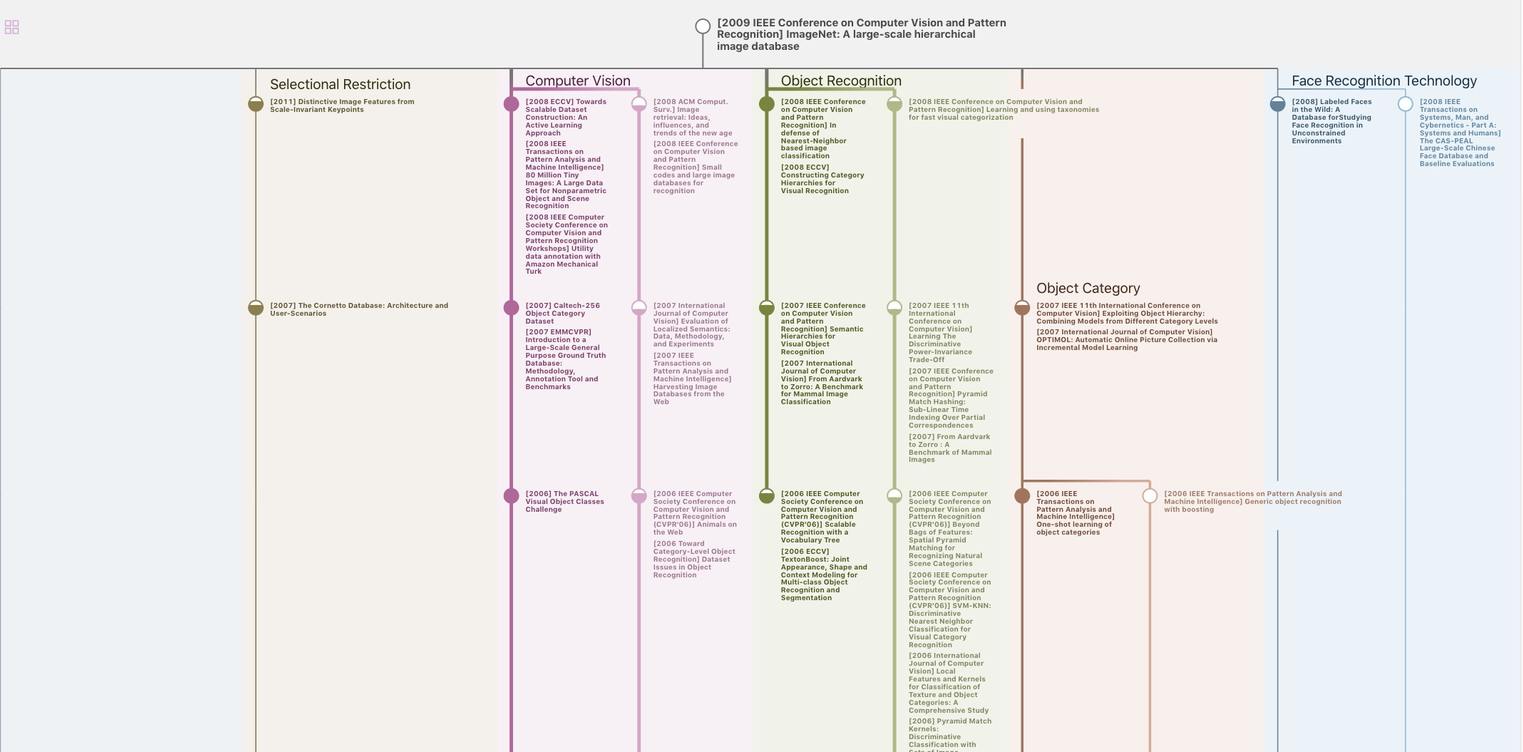
生成溯源树,研究论文发展脉络
Chat Paper
正在生成论文摘要