Spatiotemporal upscaling errors of building stock clustering for energy demand simulation
ENERGY AND BUILDINGS(2022)
摘要
Energy demand of buildings forms a key component of energy system analyses. To integrate building energy demand into energy system models, detailed simulation of every single building is impractical and often computationally infeasible. Simulations are therefore typically performed on a sample of buildings for bottom-up analysis. For a simplified representation of the building stock, grouping and clustering are widely applied. However, a spatiotemporal quantification of the resulting upscaling errors is however lacking. Here, a grouping and clustering approach is performed for all Swiss buildings for bottom-up energy demand analysis. The spatiotemporal simulation error is quantified at the national, regional and neighbourhood (300x300 m) scales. Whereas national energy demands are well represented, the error induced by the grouping and clustering is significant at the neighbourhood scale: simulated demand is easily over or underestimated by double-digit percentages. Obtained regional full load hours and daily load factor values by a grouping and clustering approach are comparable to a full simulation of all buildings. However, careful consideration must be given to the used climate data if relying on clustering approaches. Our analysis reveals the challenge of representing peak demands well by aggregation of building demand profiles, which is exacerbated by grouping and clustering. Bottom-up studies thus need to consider errors arising from the grouping and clustering approach and distinguish between errors resulting from the upscaling process and other sources of uncertainty such as data inputs or modelling concepts. Finally, calculated energy signatures are shown to perform well as a fast and viable alternative to grouping and clustering. (c) 2022 The Author(s). Published by Elsevier B.V. This is an open access article under the CC BY license (http://creativecommons.org/licenses/by/4.0/).
更多查看译文
关键词
Clustering analysis, Energy demand, Statistical upscaling, Building simulation, Geospatial analysis, Energy signatures
AI 理解论文
溯源树
样例
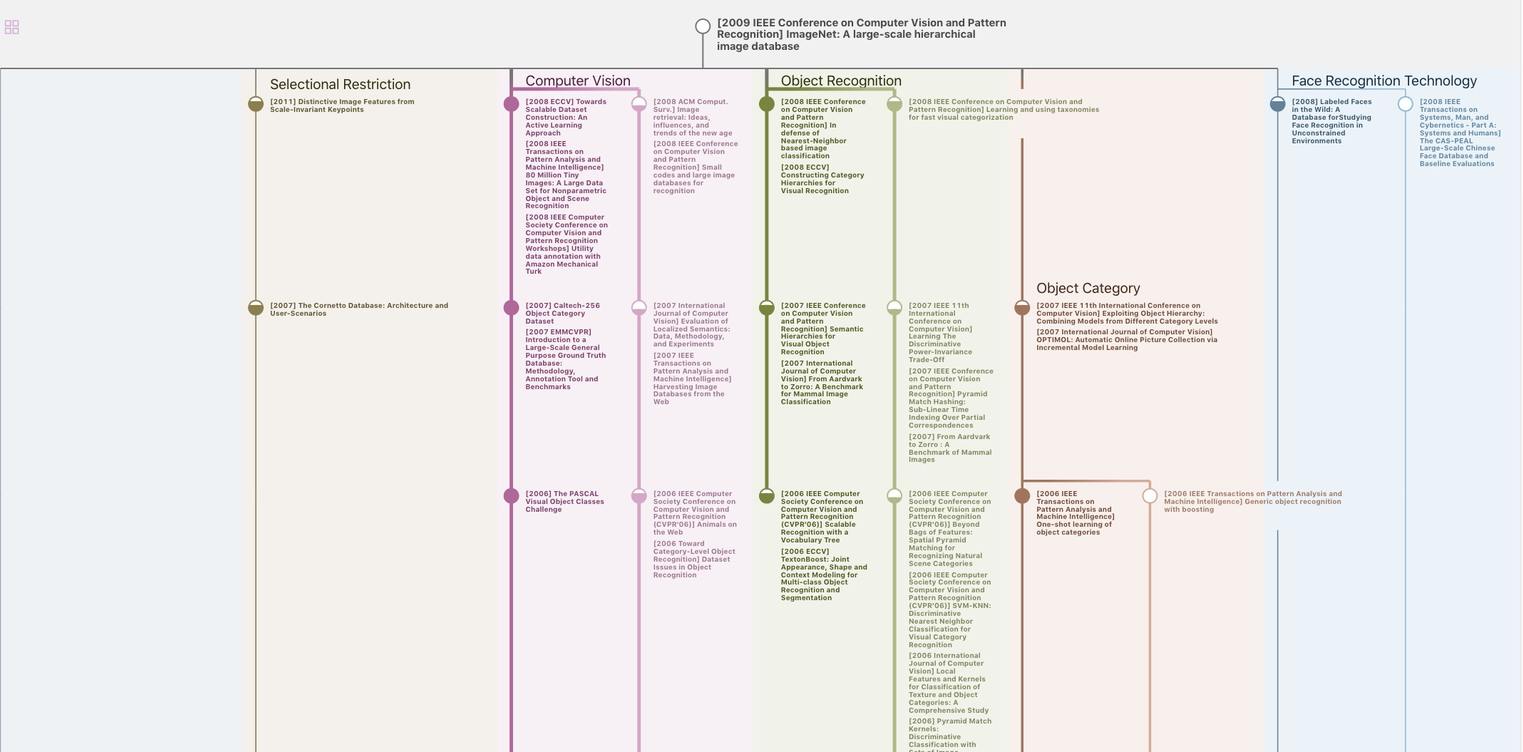
生成溯源树,研究论文发展脉络
Chat Paper
正在生成论文摘要