Cascaded Cross-Layer Fusion Network for Pedestrian Detection
MATHEMATICS(2022)
摘要
The detection method based on anchor-free not only reduces the training cost of object detection, but also avoids the imbalance problem caused by an excessive number of anchors. However, these methods only pay attention to the impact of the detection head on the detection performance, thus ignoring the impact of feature fusion on the detection performance. In this article, we take pedestrian detection as an example and propose a one-stage network Cascaded Cross-layer Fusion Network (CCFNet) based on anchor-free. It consists of Cascaded Cross-layer Fusion module (CCF) and novel detection head. Among them, CCF fully considers the distribution of high-level information and low-level information of feature maps under different stages in the network. First, the deep network is used to remove a large amount of noise in the shallow features, and finally, the high-level features are reused to obtain a more complete feature representation. Secondly, for the pedestrian detection task, a novel detection head is designed, which uses the global smooth map (GSMap) to provide global information for the center map to obtain a more accurate center map. Finally, we verified the feasibility of CCFNet on the Caltech and CityPersons datasets.
更多查看译文
关键词
pedestrian detection, machine learning, end-to-end, anchor-free, feature reuse
AI 理解论文
溯源树
样例
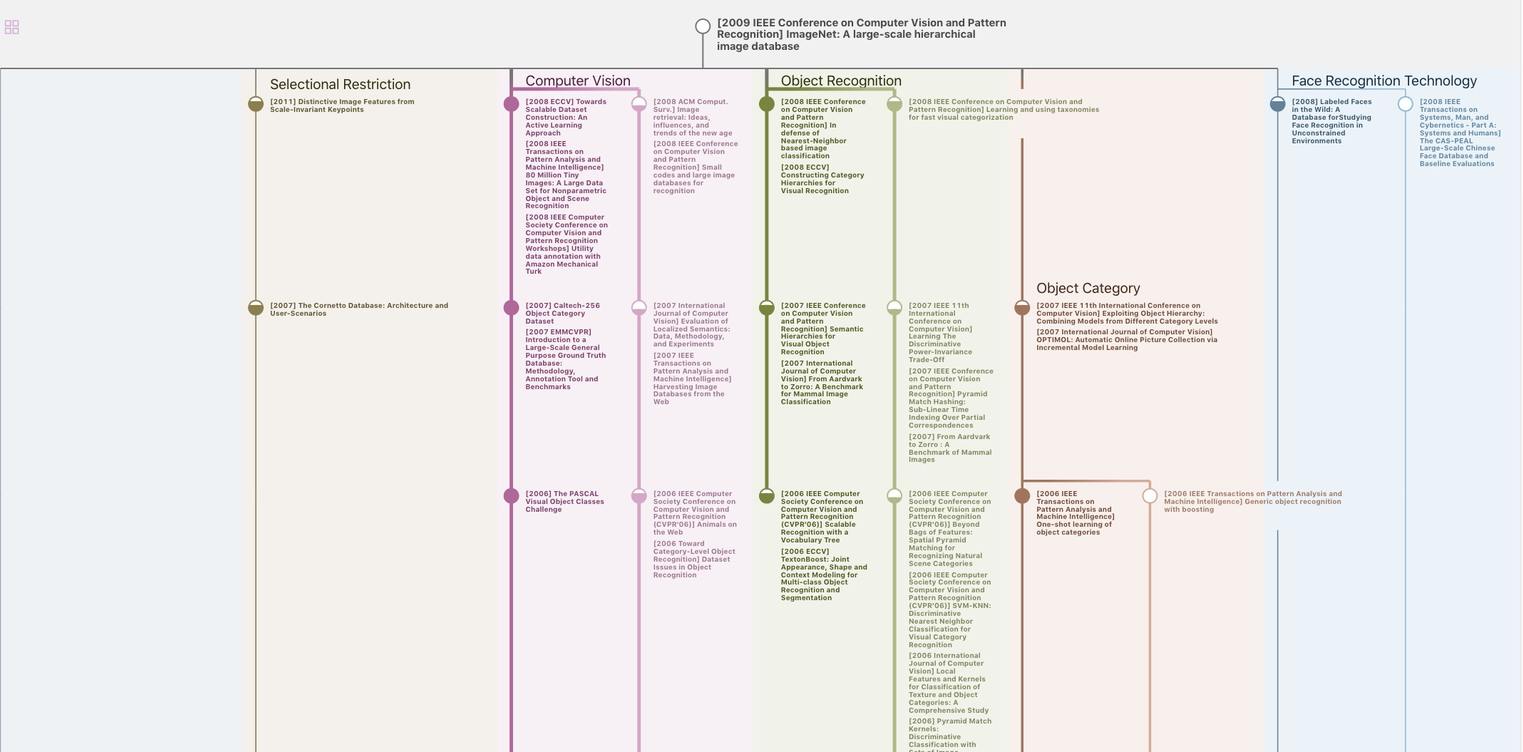
生成溯源树,研究论文发展脉络
Chat Paper
正在生成论文摘要