Evolving CNN with Paddy Field Algorithm for Geographical Landmark Recognition
ELECTRONICS(2022)
摘要
Convolutional Neural Networks (CNNs) operate within a wide variety of hyperparameters, the optimization of which can greatly improve the performance of CNNs when performing the task at hand. However, these hyperparameters can be very difficult to optimize, either manually or by brute force. Neural architecture search or NAS methods have been developed to address this problem and are used to find the best architectures for the deep learning paradigm. In this article, a CNN has been evolved with a well-known nature-inspired metaheuristic paddy field algorithm (PFA). It can be seen that PFA can evolve the neural architecture using the Google Landmarks Dataset V2, which is one of the toughest datasets available in the literature. The CNN's performance, when evaluated based on the accuracy benchmark, increases from an accuracy of 0.53 to 0.76, which is an improvement of more than 40%. The evolved architecture also shows some major improvements in hyperparameters that are normally considered to be the best suited for the task.
更多查看译文
关键词
convolutional neural network, paddy field algorithm, neural architecture search, evolution, geographical landmark recognition
AI 理解论文
溯源树
样例
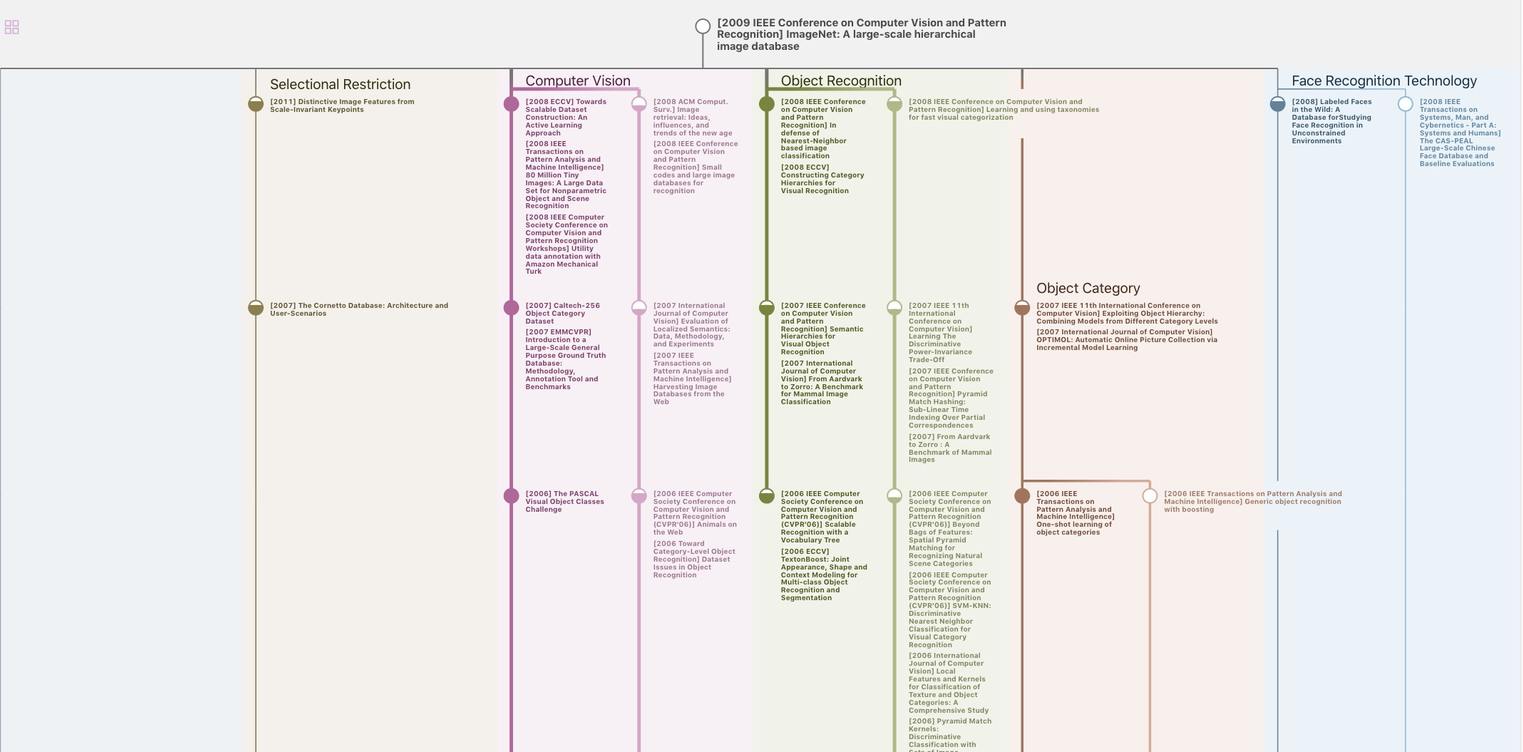
生成溯源树,研究论文发展脉络
Chat Paper
正在生成论文摘要