SMPL-Based 3D Pedestrian Pose Prediction
2021 16TH IEEE INTERNATIONAL CONFERENCE ON AUTOMATIC FACE AND GESTURE RECOGNITION (FG 2021)(2021)
摘要
Modeling human motion is a long-standing problem in computer vision. The rapid development of deep learning technologies for computer vision problems resulted in increased attention in the area of pose prediction due to its vital role in a multitude of applications, for example, behavior analysis, autonomous vehicles, and visual surveillance. In 3D pedestrian pose prediction, joint-rotation-based pose representation is extensively used due to the unconstrained degree of freedom for each joint and its ability to regress the 3D statistical wireframe. However, all the existing joint-rotation-based pose prediction approaches ignore the centrality of the distinct pose parameter components and are consequently prone to suffer from error accumulation along the kinematic chain, which results in unnatural human poses. In joint-rotation-based pose prediction, Skinned Multi-Person Linear (SMPL) parameters are widely used to represent pedestrian pose. In this work, a novel SMPL-based pose prediction network is proposed to address the centrality of each SMPL component by distributing the network weights among them. Furthermore, to constrain the network to generate only plausible human poses, an adversarial training approach is employed. The effectiveness of the proposed network is evaluated using the PedX and BEHAVE datasets. The proposed approach significantly outperforms state-of-the-art methods with improved prediction accuracy and generates plausible human pose predictions.
更多查看译文
关键词
SMPL-based 3D pedestrian pose prediction,modeling human motion,long-standing problem,deep learning technologies,computer vision problems,joint-rotation-based pose representation,3D statistical wireframe,existing joint-rotation-based,prediction approaches,unnatural human poses,novel SMPL-based,prediction network,SMPL component,plausible human poses,improved prediction accuracy
AI 理解论文
溯源树
样例
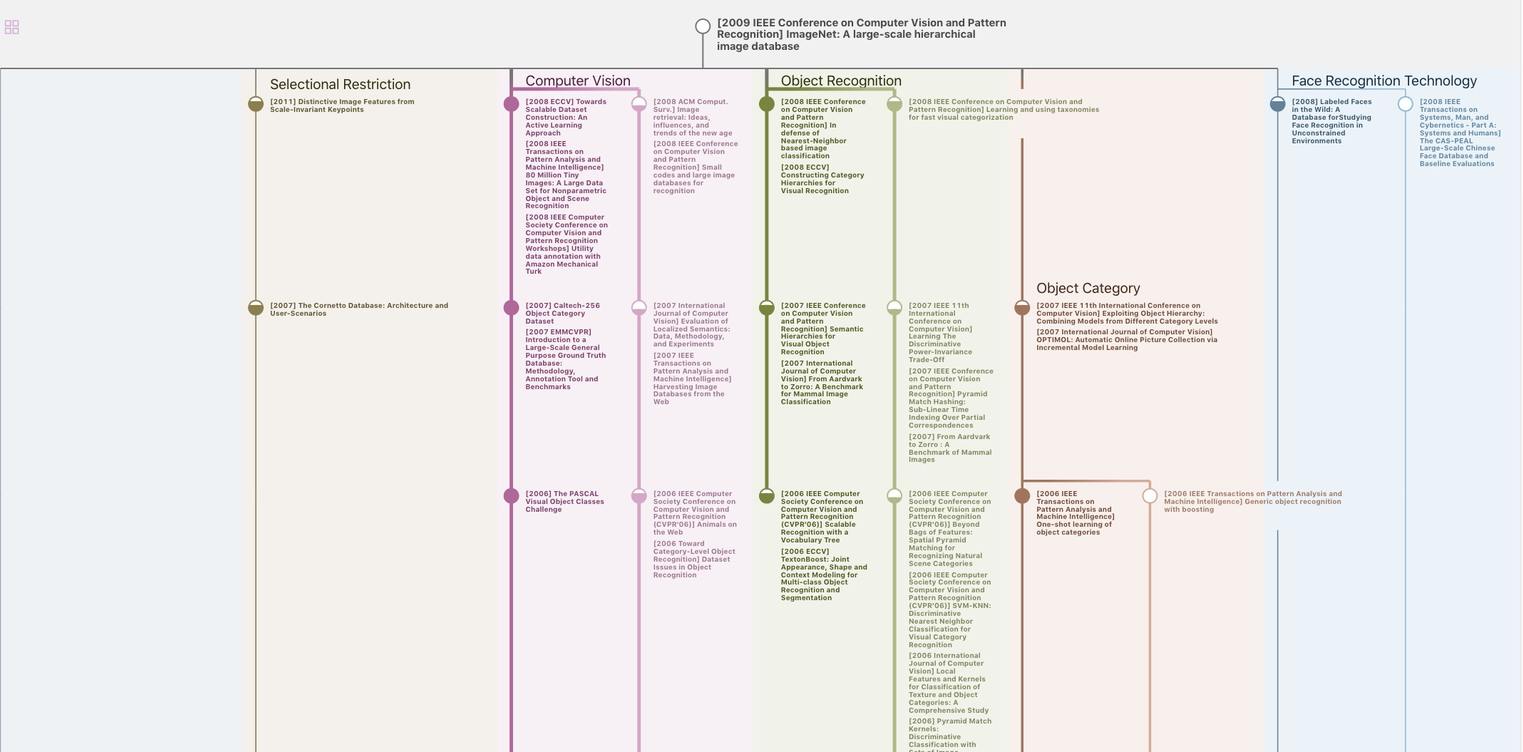
生成溯源树,研究论文发展脉络
Chat Paper
正在生成论文摘要