MULTISCALE BSBL COMPRESSED SENSING-BASED ECG SIGNAL COMPRESSION WITH ENCODING FOR TELEMEDICINE
BIOMEDICAL ENGINEERING-APPLICATIONS BASIS COMMUNICATIONS(2021)
摘要
An electrocardiogram (ECG) signal is an important diagnostic tool for cardiologists to detect the abnormality. In continuous monitoring, an ambulatory huge amount of ECG data is involved. This leads to high storage requirements and transmission costs. Hence, to reduce the storage and transmission cost, there is a requirement for an efficient compression or coding technique. One of the most promising compression techniques is Compressive Sensing (CS) which makes efficient compression of signals. By this methodology, a signal can easily be reconstructed if it has a sparse representation. This paper presents the Block Sparse Bayesian Learning (BSBL)-based multiscale compressed sensing (MCS) method for the compression of ECG signals. The main focus of the proposed technique is to achieve a reconstructed signal with less error and more energy efficiency. The ECG signal is sparsely represented by wavelet transform. MIT-BIH Arrhythmia database is used for testing purposes. The Huffman technique is used for encoding and decoding. The signal recovery is appropriate up to 75% of compression. The quality of the signal is ascertained using the standard performance measures such as signal-to-noise ratio (SNR) and Percent root mean square difference (PRD). The quality of the reconstructed ECG signal is also validated through the visual method. This method is most suitable for telemedicine applications.
更多查看译文
关键词
Compressed sensing, ECG, BSBL, compression, PRD, telemedicine
AI 理解论文
溯源树
样例
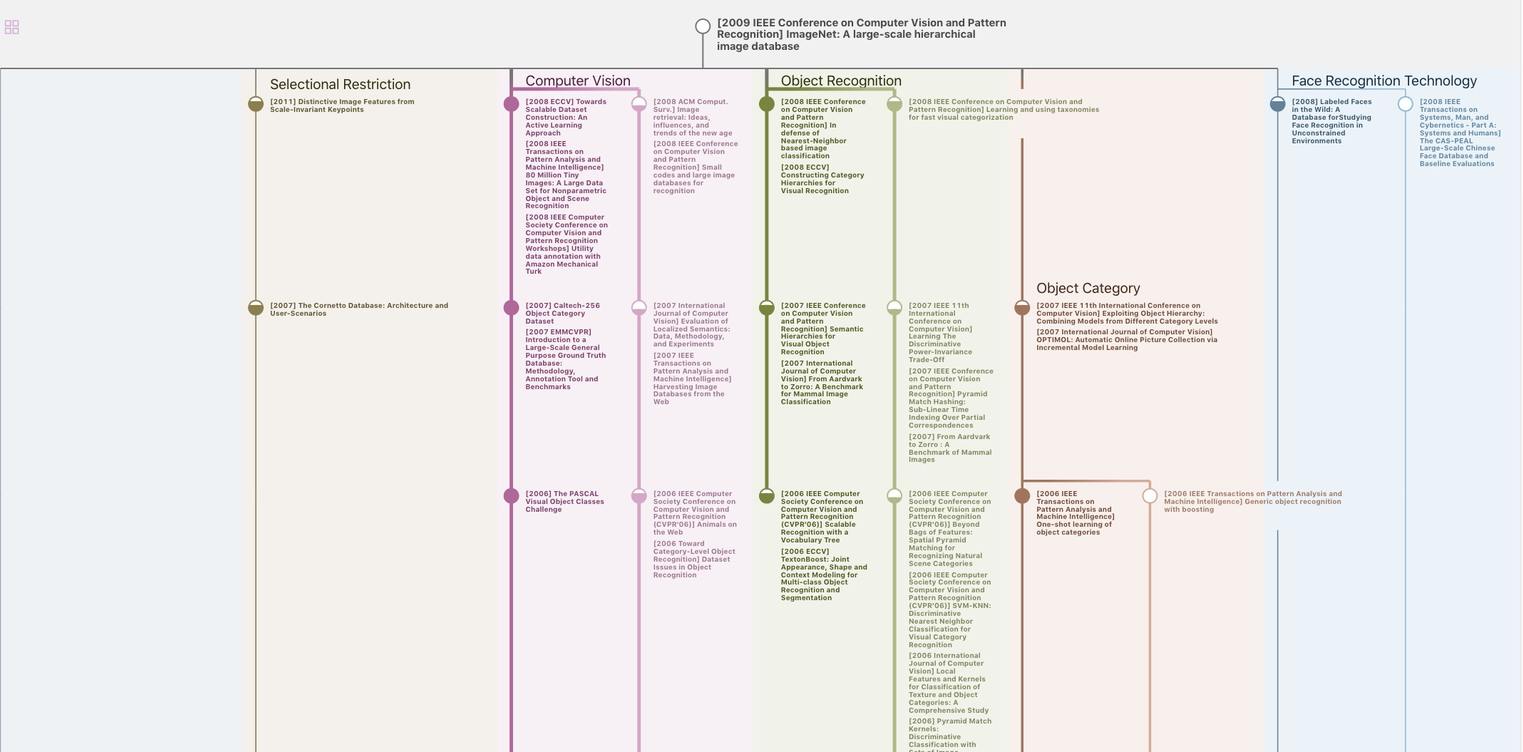
生成溯源树,研究论文发展脉络
Chat Paper
正在生成论文摘要