Evaluations of agri-environmental schemes based on observational farm data: The importance of covariate selection
Land Use Policy(2022)
摘要
Evaluations of agri-environmental schemes (AES) based on observational farm data generally use a matching algorithm for comparing participating and non-participating farms. To mitigate the potential post-matching covariate imbalances between groups resulting from the use of large covariate sets, this paper proposes a method mix that reduces the covariate set and maximises the utilised number of observations. We test the approach on an evaluation of the European Union’s AES in the programming period of 2000–2006, estimating the impacts of AES participation on typical measures of land management, i.e. fertiliser and plant protection expenditures and grassland share. We use Mahalanobis distance matching with exact matching on the entry year of the participating farms and kernel matching with automated bandwidth selection to maximise the utilised sample and increase the estimator’s efficiency. Combining cause-and-effect path analysis with statistical covariate selection algorithms reduces the covariate set and improves balance on the characteristics that describe the production environment, farming intensity, productivity, and farmers’ preferences. We find that AES generate moderate decreases in plant protection expenditure and moderate increases in grassland shares. We conclude that our proposed method mix ensures an efficient use of information and improves the reliability of AES impact evaluation.
更多查看译文
关键词
Q18,Q24,Q58,D04
AI 理解论文
溯源树
样例
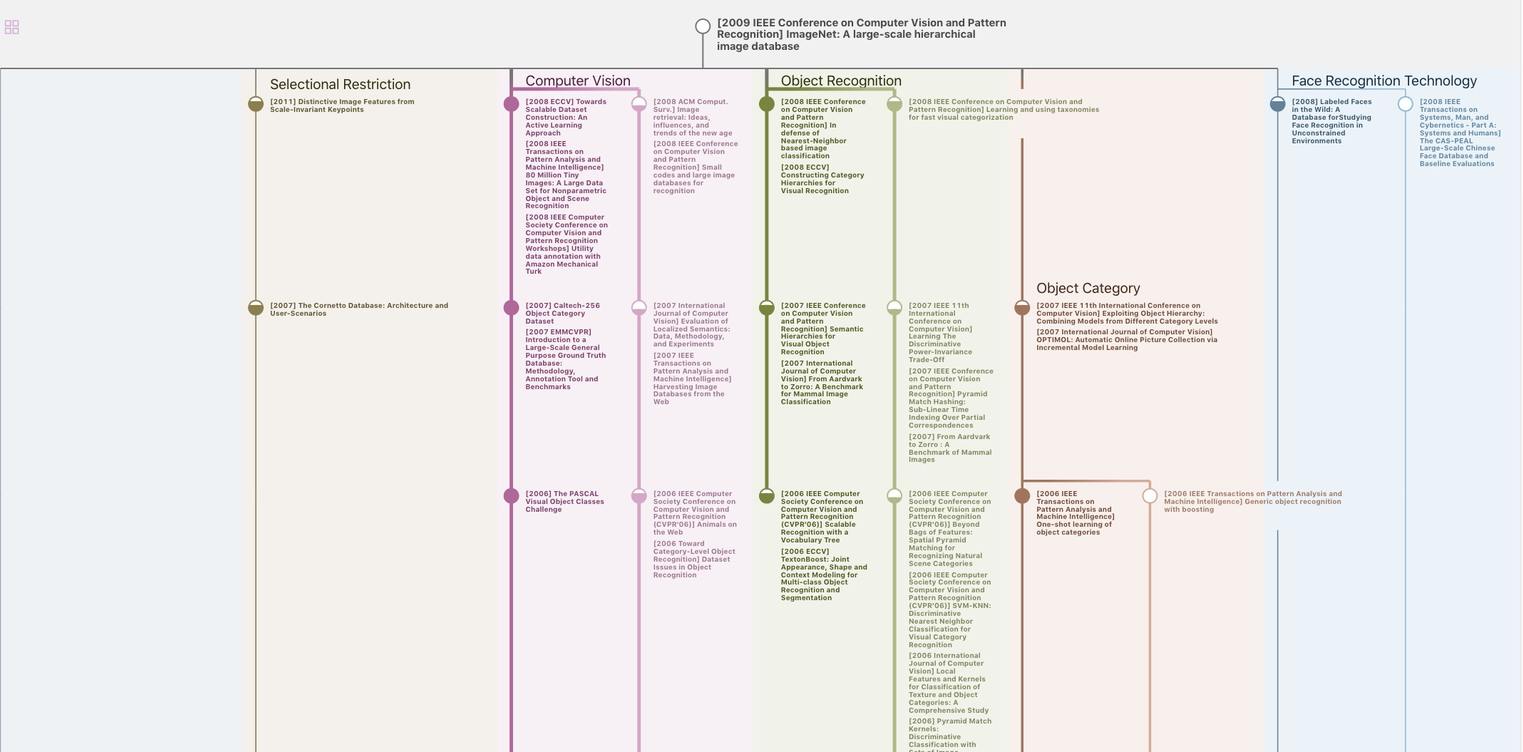
生成溯源树,研究论文发展脉络
Chat Paper
正在生成论文摘要