Bayesian nonparametric quantile mixed-effects models via regularization using Gaussian process priors
Japanese Journal of Statistics and Data Science(2022)
摘要
In this study, we proposed using Bayesian nonparametric quantile mixed-effects models (BNQMs) to estimate the nonlinear structure of quantiles in hierarchical data. Assuming that a nonlinear function representing a phenomenon of interest cannot be specified in advance, a BNQM can estimate the nonlinear function of quantile features using the basis expansion method. Furthermore, BNQMs adjust the smoothness to prevent overfitting by regularization. We also proposed a Bayesian regularization method using Gaussian process priors for the coefficient parameters of the basis functions, and showed that the problem of overfitting can be reduced when the number of basis functions is excessive for the complexity of the nonlinear structure. Although computational cost is often a problem in quantile regression modeling, BNQMs ensure the computational cost is not too high using a fully Bayesian method. Using numerical experiments, we showed that the proposed model can estimate nonlinear structures of quantiles from hierarchical data more accurately than the comparison models in terms of mean squared error. Finally, to determine the cortisol circadian rhythm in infants, we applied a BNQM to longitudinal data of urinary cortisol concentration collected at Kurume University. The result suggested that infants have a bimodal cortisol circadian rhythm before their biological rhythms are established.
更多查看译文
关键词
Basis expansion, Circadian rhythm, Longitudinal data, Mixed-effects models, Infants, Quantile regression, 62G08, 62J99
AI 理解论文
溯源树
样例
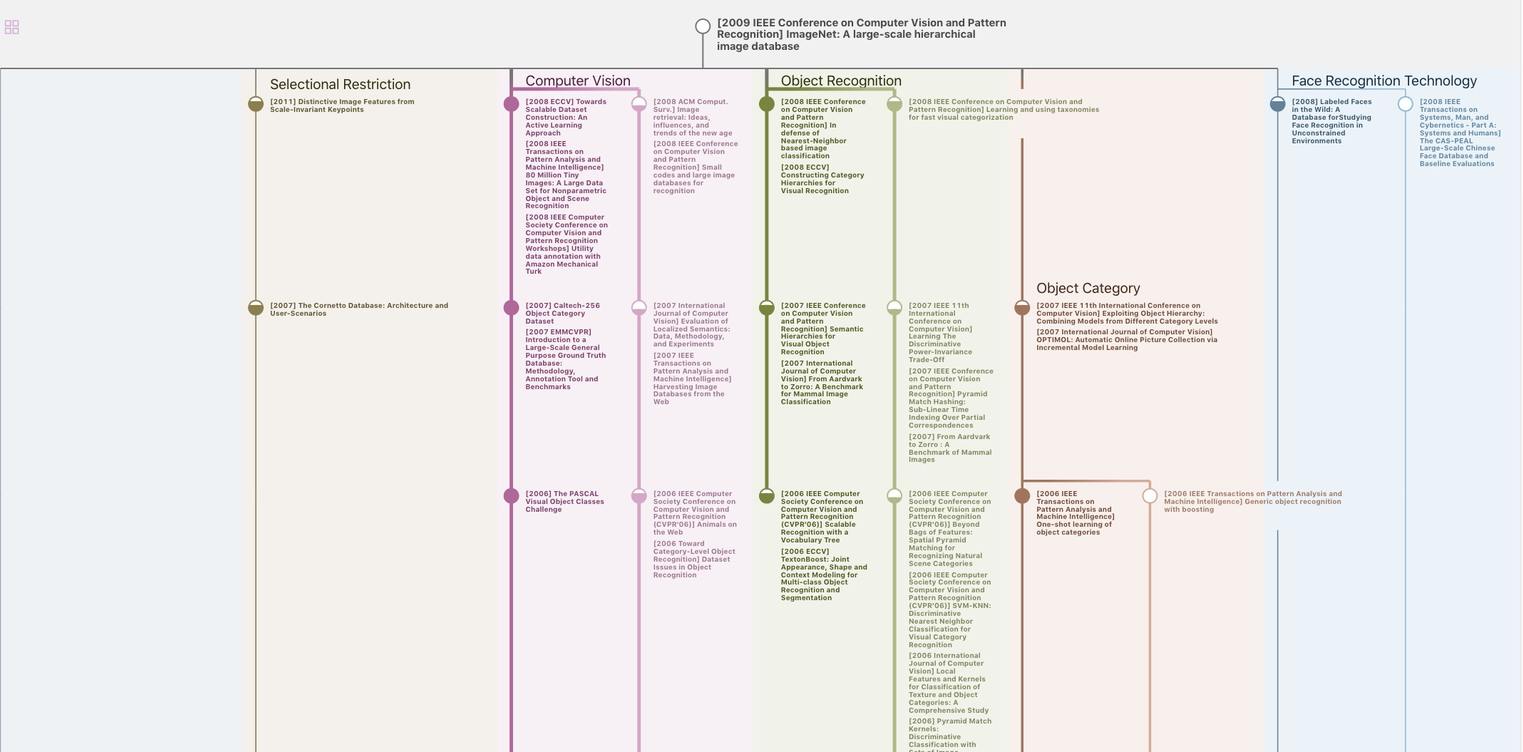
生成溯源树,研究论文发展脉络
Chat Paper
正在生成论文摘要