Probabilistic Gas Leak Rate Estimation Using Submodular Function Maximization With Routing Constraints
IEEE ROBOTICS AND AUTOMATION LETTERS(2022)
摘要
Harmful greenhouse gases such as methane are prone to leak during extraction, transportation, and storage in oil fields. Therefore we must monitor gas leak rates to keep such fugitive emissions in check. However, most currently available approaches incur significant computational costs to generate informative paths for mobile sensors and estimate leak rates from the collected data. As such, they do not scale to large oil fields and are infeasible for real-time applications. We address these problems by deriving an efficient analytical approach to compute the leak rate distribution and Expected Entropy Reduction (EER) metric used for path generation. Moreover, a faster variant of a submodular function maximization algorithm is introduced, along with a generalization of the algorithm to find informative data collection walks with arc routing constraints. Our simulation experiments demonstrate the approach's validity and substantial computational gains.
更多查看译文
关键词
Environment monitoring and management, fugitive emissions, integrated planning and learning, informative path planning, probabilistic inference
AI 理解论文
溯源树
样例
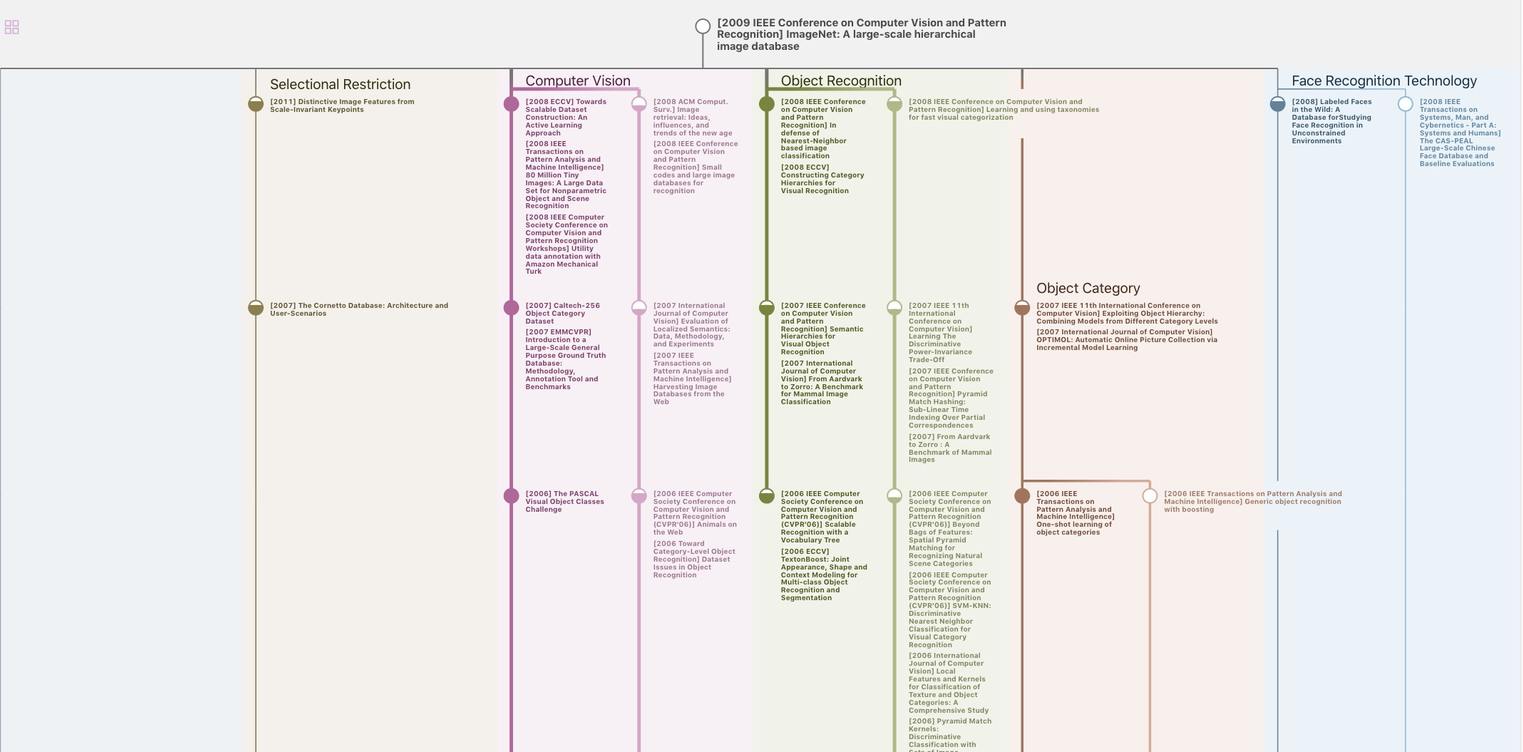
生成溯源树,研究论文发展脉络
Chat Paper
正在生成论文摘要