The Prediction of Essential Medicines Demand: A Machine Learning Approach Using Consumption Data in Rwanda
PROCESSES(2022)
摘要
Today's global business trends are causing a significant and complex data revolution in the healthcare industry, culminating in the use of artificial intelligence and predictive modeling to improve health outcomes and performance. The dataset, which was referred to is based on consumption data from 2015 to 2019, included approximately 500 goods. Based on a series of data pre-processing activities, the top ten (10) essential medicines most used were chosen, namely cotrimoxazole 480 mg, amoxicillin 250 mg, paracetamol 500 mg, oral rehydration salts (O.R.S) sachet 20.5 g, chlorpheniramine 4 mg, nevirapine 200 mg, aminophylline 100 mg, artemether 20 mg + lumefantrine (AL) 120 mg, Cromoglycate ophthalmic. Our study concentrated on the application of machine learning (ML) to forecast future trends in the demand for essential drugs in Rwanda. The following models were created and applied: linear regression, artificial neural network, and random forest. The random forest was able to predict 10 selected medicines with an accuracy of 88 percent with the train set and 76 percent with the test set, and it can thus be used to forecast future demand based on past consumption data by inputting a month, year, district, and medicine name. According to our findings, the random Forest model performed well as a forecasting model for the demand for essential medicines. Finally, data-driven predictive modeling with machine learning (ML) could become the cornerstone of health supply chain planning and operational management.
更多查看译文
关键词
forecasting models, essential medicines, consumption data, health supply chain, machine learning, Rwanda
AI 理解论文
溯源树
样例
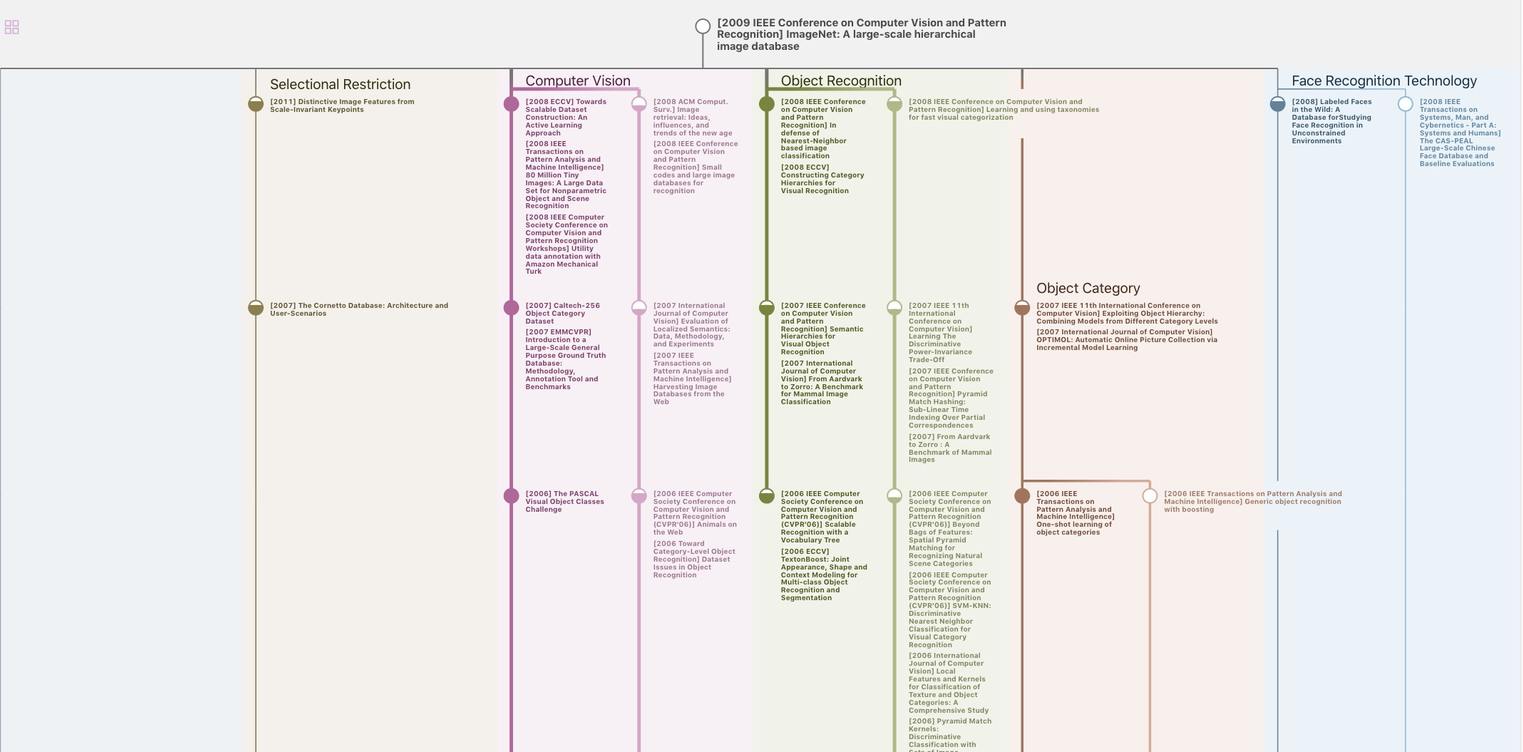
生成溯源树,研究论文发展脉络
Chat Paper
正在生成论文摘要