Computing quantities of interest and their uncertainty using Bayesian simulation
POLITICAL SCIENCE RESEARCH AND METHODS(2023)
摘要
When analyzing data, researchers are often less interested in the parameters of statistical models than in functions of these parameters such as predicted values. Here we show that Bayesian simulation with Markov-Chain Monte Carlo tools makes it easy to compute these quantities of interest with their uncertainty. We illustrate how to produce customary and relatively new quantities of interest such as variable importance ranking, posterior predictive data, difficult marginal effects, and model comparison statistics to allow researchers to report more informative results.
更多查看译文
关键词
Bayesian,categorical data analysis,discrete choice models,linear regression
AI 理解论文
溯源树
样例
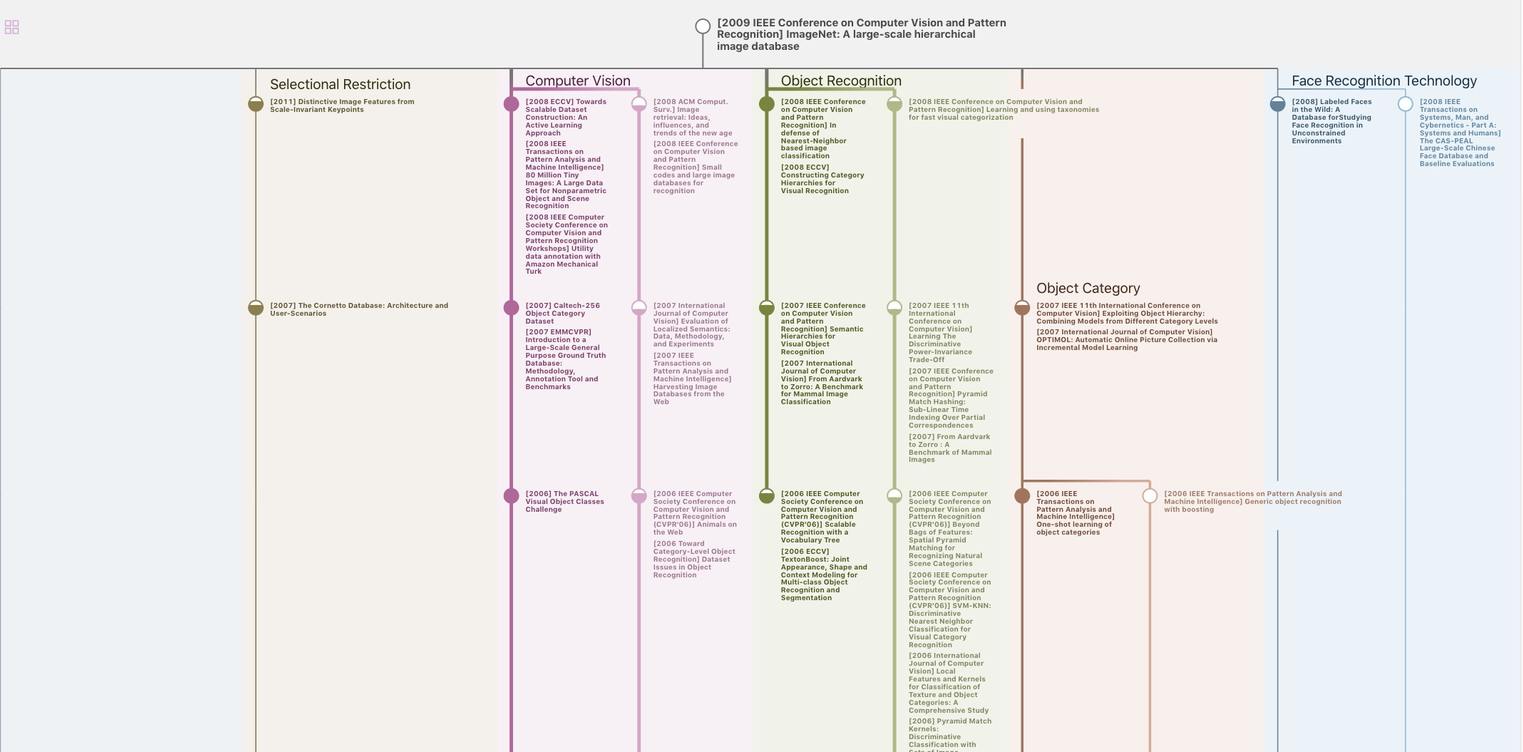
生成溯源树,研究论文发展脉络
Chat Paper
正在生成论文摘要