Using Accelerometers to Detect Activity Type in a Sport Setting: Challenges with Using Multiple Types of Conventional Machine Learning Approaches
MEASUREMENT IN PHYSICAL EDUCATION AND EXERCISE SCIENCE(2023)
摘要
A universal approach to characterizing sport-related physical activity (PA) types in sport settings does not yet exist. Young adults (n = 30), 19-33 years, engaged in a 15-min activity session, performing warm-ups, 3-on-3 soccer, and 3-on-3 basketball. Videos were recorded and manually coded as criterion PA types (walking, running, jumping, rapid lateral movements). Participants wore an accelerometer on their right hip. Multiple machine learning models were developed and compared for predicting PA type. Most models underestimated time spent completing the activities performed least commonly. Point estimates for percent agreement, sensitivity, specificity, F-scores, and kappa were similar across models, with Hidden Markov Models (HMMs) being best at classifying rare events. Models detected activity type during sport-related movements with modest accuracy (kappas <= .40). Given the better performance of HMMs, incorporating the temporal nature of sport-related activities is important for improving sport-related PA classification.
更多查看译文
关键词
Activity monitor, device, young adult, random forest, hidden Markov models
AI 理解论文
溯源树
样例
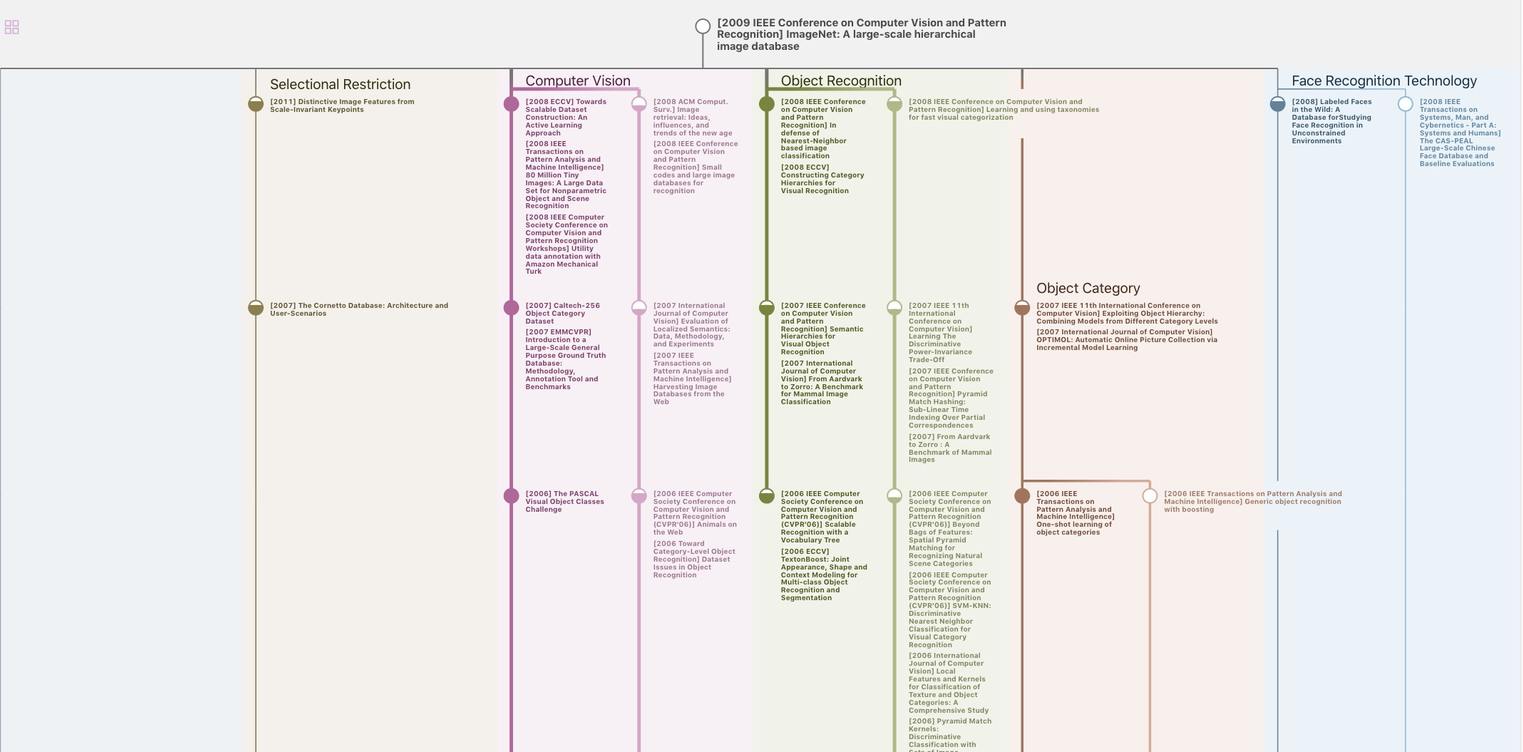
生成溯源树,研究论文发展脉络
Chat Paper
正在生成论文摘要