A Model-free Deep Reinforcement Learning Approach for Robotic Manipulators Path Planning
2021 21ST INTERNATIONAL CONFERENCE ON CONTROL, AUTOMATION AND SYSTEMS (ICCAS 2021)(2021)
摘要
Path planning problems have attracted much attention in robotic fields such as manipulators. In this paper, a model-free off-policy actor critic based deep reinforcement learning method is proposed to solve the classical path planning problem of a UR5 robot arm. Unlike standard path planning methods, the reward design of the proposed method contains smoothness reward, which assures smooth trajectory of the UR5 robot arm when accomplishing path planning tasks. Additionally, the proposed method does not rely on any model while the standard path planning method is modelbased. The proposed method not only guarantees that the joint angle of the UR5 robotic arm lies within the allowable range each time when it reaches the random target point, but also ensures that the joint angle of the UR5 robotic arm is always within the allowable range during the entire episode of training. A standard path planning method was implemented in Robot Operating System (ROS) and the proposed method was applied in CoppeliaSim to validate the feasibility. It can be inferred from the experiment that the training with the proposed method is successful.
更多查看译文
关键词
Model-free, Deep Reinforcement Learning, Manipulators, Path Planning
AI 理解论文
溯源树
样例
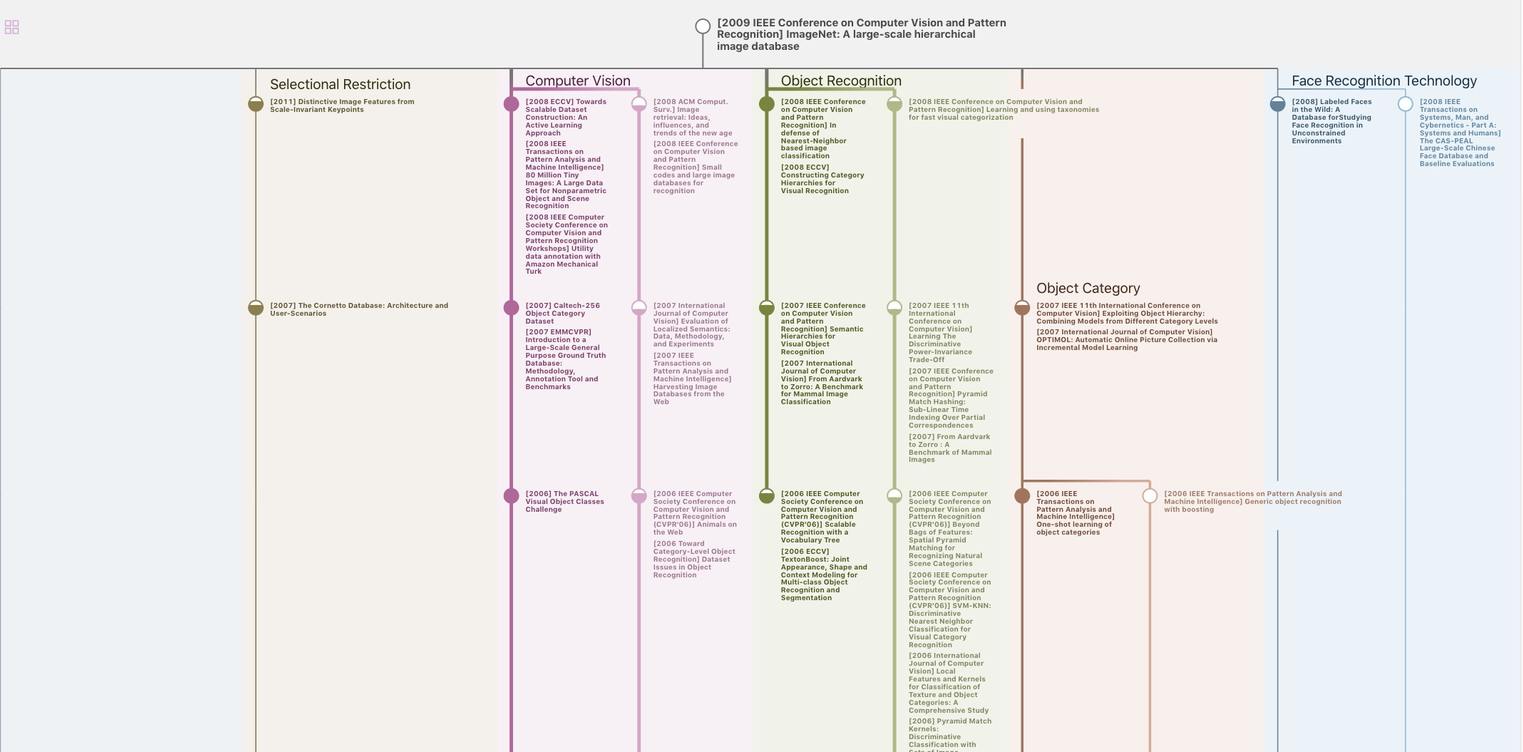
生成溯源树,研究论文发展脉络
Chat Paper
正在生成论文摘要