Benchmarked linear shrinkage prediction in the Fay-Herriot small area model
SCANDINAVIAN JOURNAL OF STATISTICS(2023)
摘要
The empirical best linear unbiased predictor (EBLUP) is a linear shrinkage of the direct estimate toward the regression estimate and useful for the small area estimation in the sense of increasing precision of estimation of small area means. However, one potential difficulty of EBLUP is that the overall estimate for a larger geographical area based on a sum of EBLUP is not necessarily identical to the corresponding direct estimate like the overall sample mean. To fix this problem, the paper suggests a new method for benchmarking EBLUP in the Fay-Herriot model without assuming normality of random effects and sampling errors. The resulting benchmarked empirical linear shrinkage (BELS) predictor has novelty in the sense that coefficients for benchmarking are adjusted based on the data from each area. To measure the uncertainty of BELS, the second-order unbiased estimator of the mean squared error is derived.
更多查看译文
关键词
benchmark,best linear unbiased predictor,Fay-Herriot model,linear shrinkage,mean squared error,non normal distribution,small area estimation
AI 理解论文
溯源树
样例
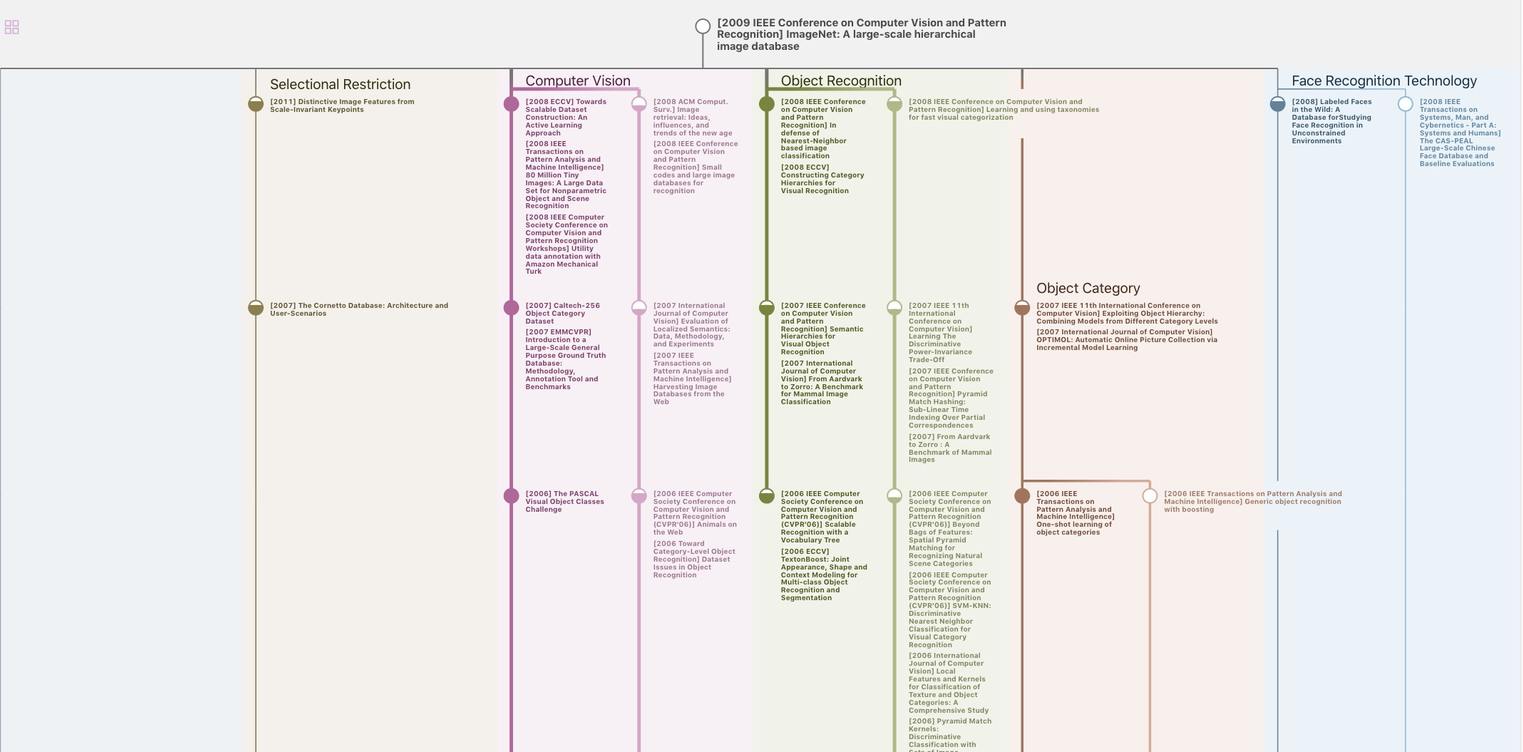
生成溯源树,研究论文发展脉络
Chat Paper
正在生成论文摘要