Missing Precipitation Data Estimation Using Long Short-Term Memory Deep Neural Networks
JOURNAL OF ECOLOGICAL ENGINEERING(2022)
摘要
Due to the spatiotemporal variability of precipitation and the complexity of physical processes involved, missing precipitation data estimation remains as a significant problem. Algeria, like other countries in the world, is affected by this problem. In the present paper, Long Short-Term Memory (LSTM) deep neural Networks model was tested to estimate missing monthly precipitation data. The application was presented for the K'sob basin, Algeria. In the present paper, the optimal architecture of LSTM model was adjusted by trial-and-error-procedure. The LSTM model was compared with the most widely used classical methods including inverse distance weighting method (IDWM) and the coefficient of correlation weighting method (CCWM). Finally, it was concluded that the LSTM model performed better than the other methods.
更多查看译文
关键词
hodna, K'sob basin, missing precipitation data, long short-term memory, CCWM, IDWM
AI 理解论文
溯源树
样例
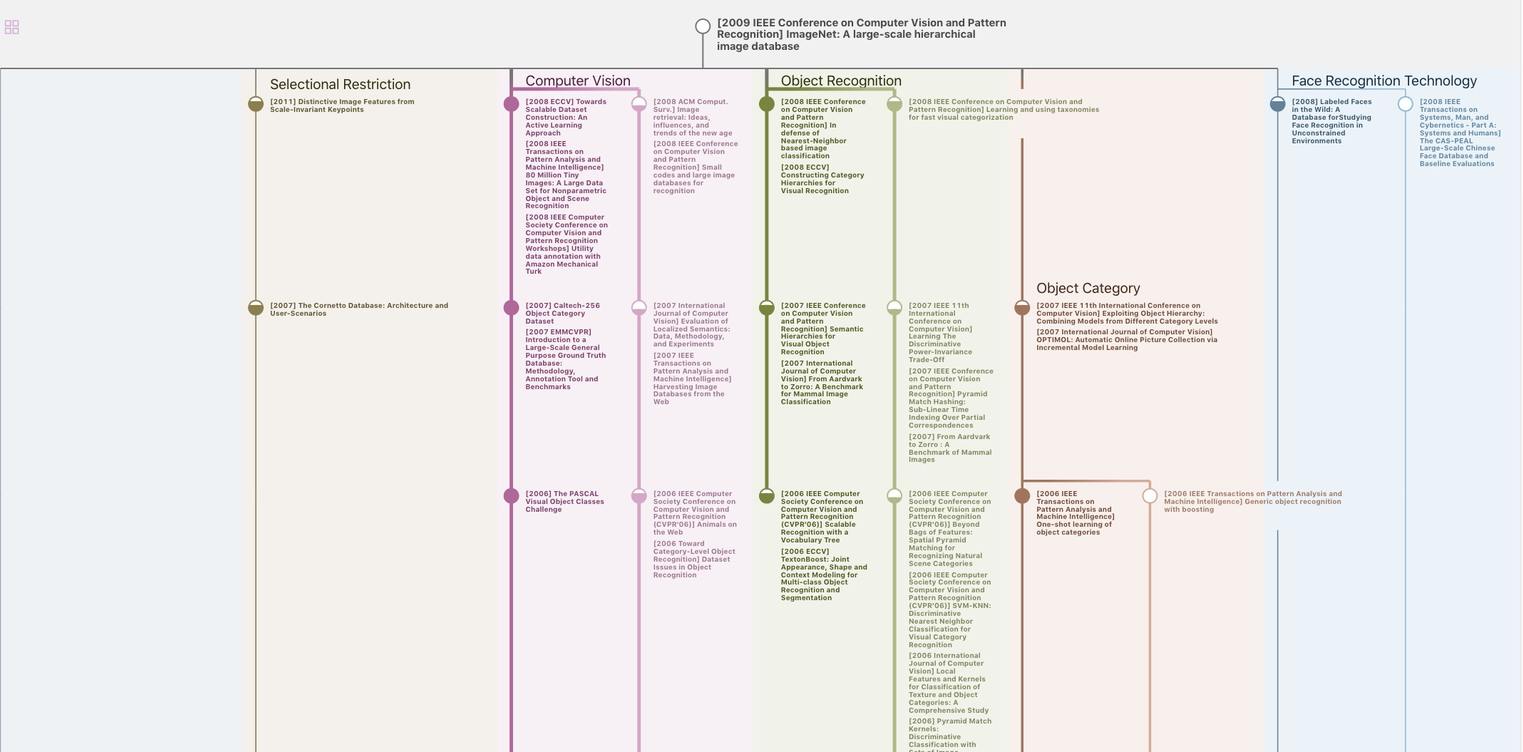
生成溯源树,研究论文发展脉络
Chat Paper
正在生成论文摘要