Double Granularity Relation Network with Self-criticism for Occluded Person Re-identification
MULTIMEDIA MODELING (MMM 2022), PT I(2022)
Abstract
Occluded person Re-identification is still a challenge. Most existing methods capture visible human parts based on external cues, such as human pose and semantic mask. In this paper, we propose a double granularity relation network with self-criticism to locate visible human parts. We learn the region-wise relation between part and whole and pixel-wise relation between pixel and whole. The relations find non-occluded human body parts and exclude noisy information. To guide the relation learning, we introduce two relation critic losses, which score the parts and maximize the performance by imposing higher weights on large parts and lower ones on small parts. We design the double branch model based on the proposed critic loss and evaluate it on the popular benchmarks. The experimental results show the superiority of our method, which achieves mAP of 51.0% and 75.4% respectively on Occluded-DukeMTMC and P-DukeMTMC-reID. Our codes are available at DRNC.
MoreTranslated text
Key words
Occluded person re-identification, Relationships learning, Relation critic loss
AI Read Science
Must-Reading Tree
Example
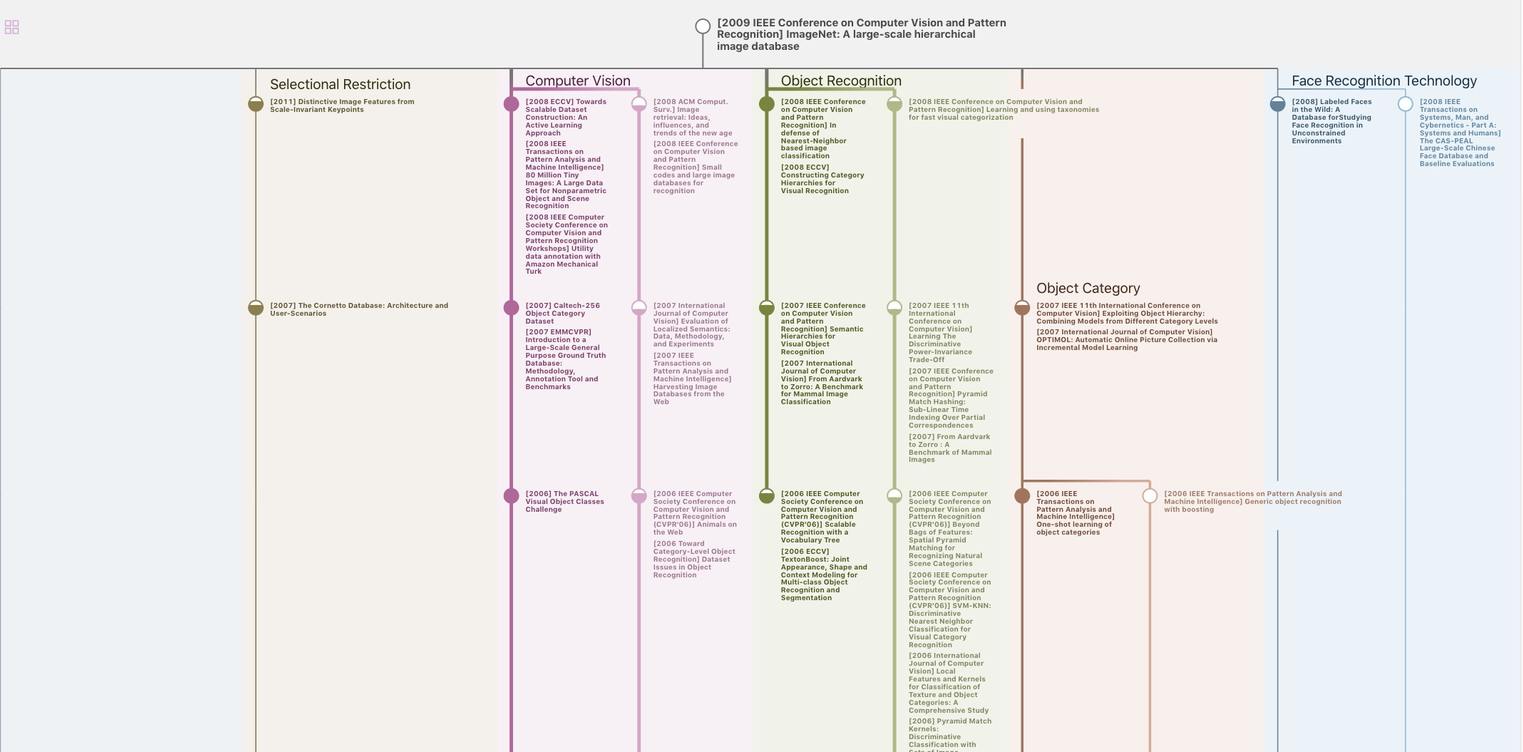
Generate MRT to find the research sequence of this paper
Chat Paper
Summary is being generated by the instructions you defined