A Novel High-Order Cluster-GCN-Based Approach for Service Recommendation
WEB SERVICES - ICWS 2021(2022)
摘要
When exploring high-order neighbors for embedding learning, data sparsity problems in service recommendation system can be compensated via Graph Convolutional Network (GCN). However, the performance of GCN will deteriorate when stacking more layers, namely, over-smoothing problem. Though LightGCN and LR-GCN can alleviate over-smoothing and achieve state-of-the-art performance, all users with dissimilar preferences become similar and the services become homogeneous, introducing noise information in exploration high-order graph convolution. Thus, we argue that the loss of uniqueness of all nodes is the cause of over-smoothing problems in high-order graph convolution. To solve the above problems, we propose to use graph clustering algorithm to cluster user-service graph. Moreover, this node enhancement technique in our model can further facilitate systems to learn more information from nearby neighbors. The experimental results confirm that the proposed algorithm outperforms most baseline algorithms, achieving state-of-the-art performance.
更多查看译文
关键词
Service recommendation, Graph convolution networks, Clustering
AI 理解论文
溯源树
样例
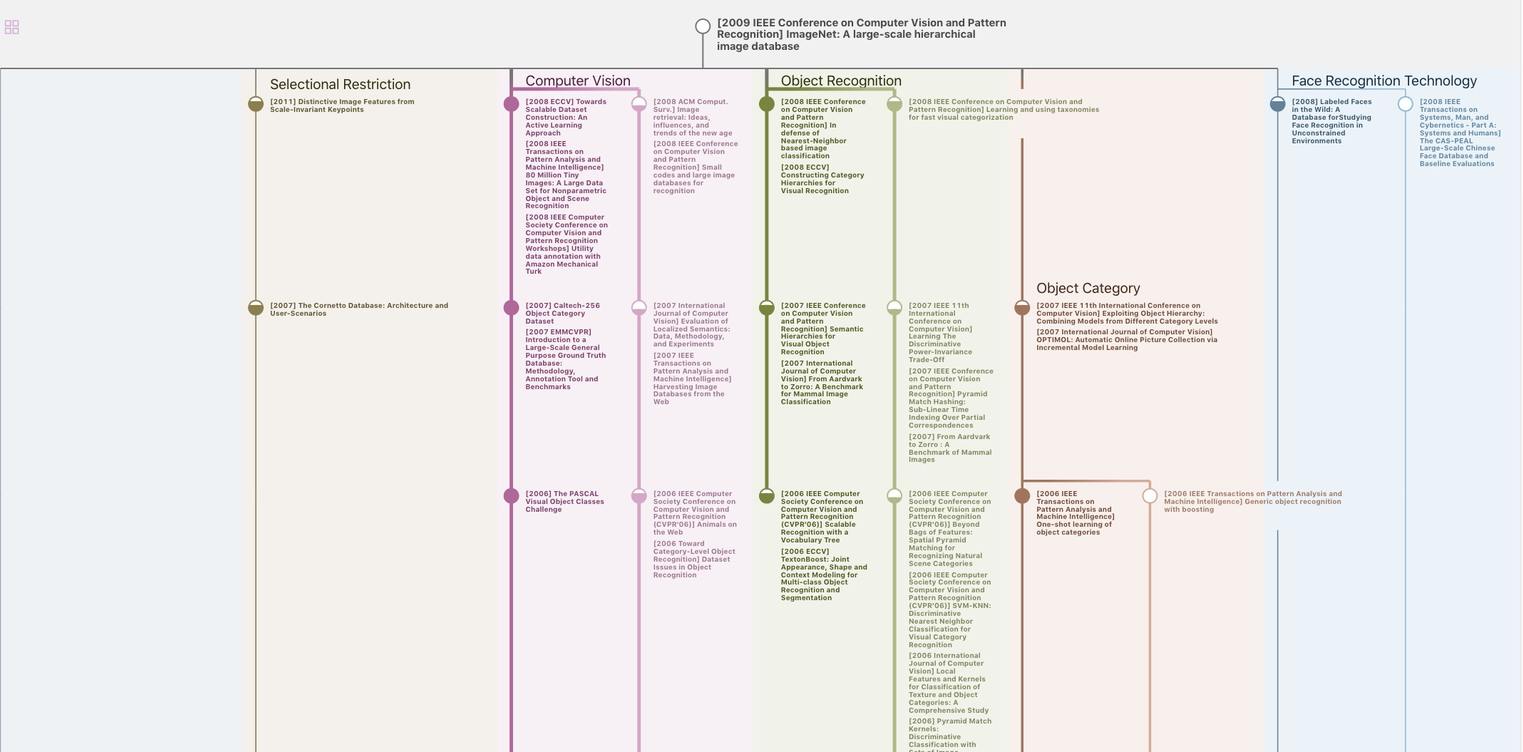
生成溯源树,研究论文发展脉络
Chat Paper
正在生成论文摘要