Latent Multi-view Subspace Clustering Based on Schatten-P Norm
PARALLEL AND DISTRIBUTED COMPUTING, APPLICATIONS AND TECHNOLOGIES, PDCAT 2021(2022)
摘要
In this paper, we aim at the research of rank minimization to find more accurate low-dimensional representations for multi-view subspace learning. The Schatten-p norm is utilized as the rank relaxation function for subspace learning to enhance its ability to recover the low rank matrices, and a multi-view subspace clustering algorithm via maximizing the original feature information is proposed under the assumption that each view is derived from a latent representation. With the Schatten-p norm, the proposed algorithm can improve the quality and robustness of the latent representations. The effectiveness of our method is validated through experiments on several benchmark datasets.
更多查看译文
关键词
Latent multi-view subspace clustering, Rank function, Schatten-p norm
AI 理解论文
溯源树
样例
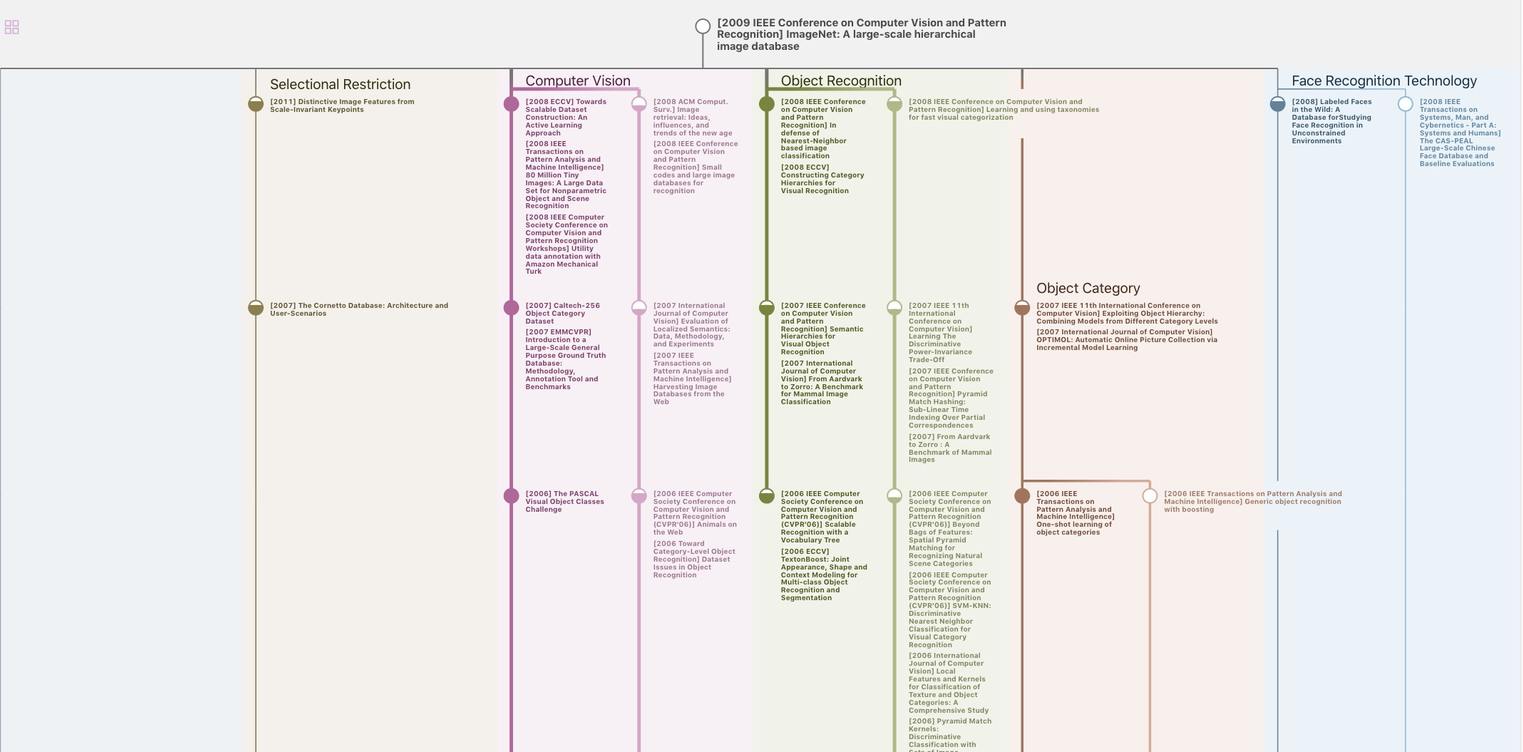
生成溯源树,研究论文发展脉络
Chat Paper
正在生成论文摘要