Maize Small Leaf Spot Classification Based on Improved Deep Convolutional Neural Networks with a Multi-Scale Attention Mechanism
AGRONOMY-BASEL(2022)
摘要
Maize small leaf spot (Bipolaris maydis) is one of the most important diseases of maize. The severity of the disease cannot be accurately identified, the cost of pesticide application increases every year, and the agricultural ecological environment is polluted. Therefore, in order to solve this problem, this study proposes a novel deep learning network DISE-Net. We designed a dilated-inception module instead of the traditional inception module for strengthening the performance of multi-scale feature extraction, then embedded the attention module to learn the importance of interchannel relationships for input features. In addition, a dense connection strategy is used in model building to strengthen channel feature propagation. In this paper, we constructed a data set of maize small leaf spot, including 1268 images of four disease grades and healthy leaves. Comparative experiments show that DISE-Net with a test accuracy of 97.12% outperforms the classical VGG16 (91.11%), ResNet50 (89.77%), InceptionV3 (90.97%), MobileNetvl (92.51%), MobileNetv2 (92.17%) and DenseNet121 (94.25%). In addition, Grad-Cam network visualization also shows that DISE-Net is able to pay more attention to the key areas in making the decision. The results showed that the DISE-Net was suitable for the classification of maize small leaf spot in the field.
更多查看译文
关键词
small spot disease, disease grading, dilated inception, multi-scale feature extraction, deep learning
AI 理解论文
溯源树
样例
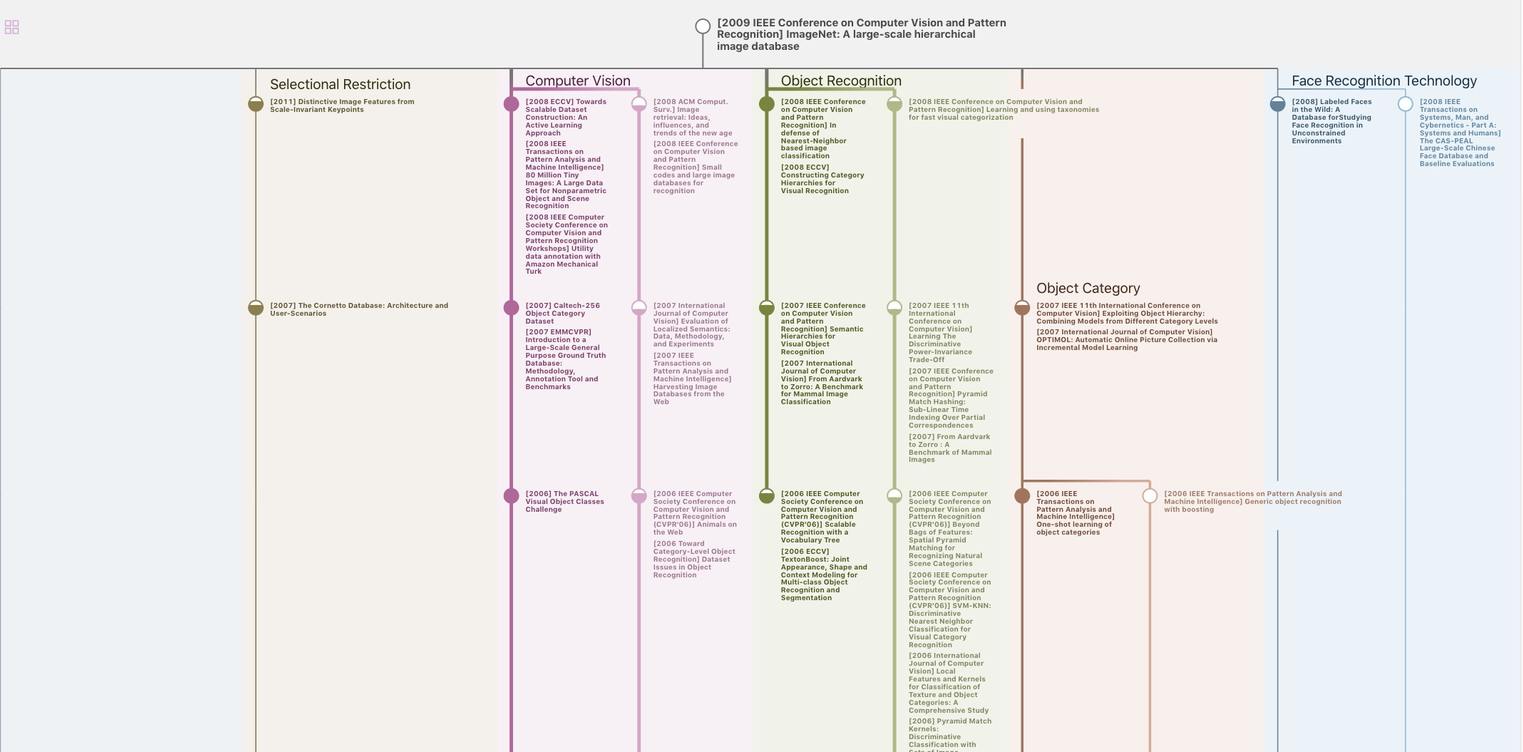
生成溯源树,研究论文发展脉络
Chat Paper
正在生成论文摘要