Therapeutic response in the HAWK and HARRIER trials using deep learning in retinal fluid volume and compartment analysis
EYE(2022)
摘要
Objectives To assess the therapeutic response to brolucizumab and aflibercept by deep learning/OCT-based analysis of macular fluid volumes in neovascular age-related macular degeneration. Methods In this post-hoc analysis of two phase III, randomised, multi-centre studies (HAWK/HARRIER), 1078 and 739 treatment-naive eyes receiving brolucizumab or aflibercept according to protocol-specified criteria in HAWK and HARRIER, respectively, were included. Macular fluid on 41,840 OCT scans was localised and quantified using a validated deep learning-based algorithm. Volumes of intraretinal fluid (IRF), subretinal fluid (SRF), pigment epithelial detachment (PED) for all central macular areas (1, 3 and 6 mm) in nanolitres (nL) and best corrected visual acuity (BCVA) change in ETDRS letters were associated using mixed models for repeated measures. Results Baseline IRF volumes decreased by >92% following the first intravitreal injection and consistently remained low during follow-up. Baseline SRF volumes decreased by >74% following the first injection, while PED volume resolved by 68–79% of its baseline volume. Resolution of SRF and PED was dependent on the substance and regimen used. Larger residual post-loading IRF, SRF and PED volumes were all independently associated with progressive vision loss during maintenance, where the differences in mean BCVA change between high and low fluid volume subgroups for IRF, SRF and PED were 3.4 letters ( p < 0.0001), 1.7 letters ( p < 0.001) and 2.5 letters ( p < 0.0001), respectively. Conclusions Deep-learning methods allow an accurate assessment of substance and regimen efficacy. Irrespectively, all fluid compartments were found to be important markers of disease activity and were relevant for visual outcomes.
更多查看译文
关键词
Macular degeneration,Retinal diseases,Medicine/Public Health,general,Ophthalmology,Laboratory Medicine,Surgery,Surgical Oncology,Pharmaceutical Sciences/Technology
AI 理解论文
溯源树
样例
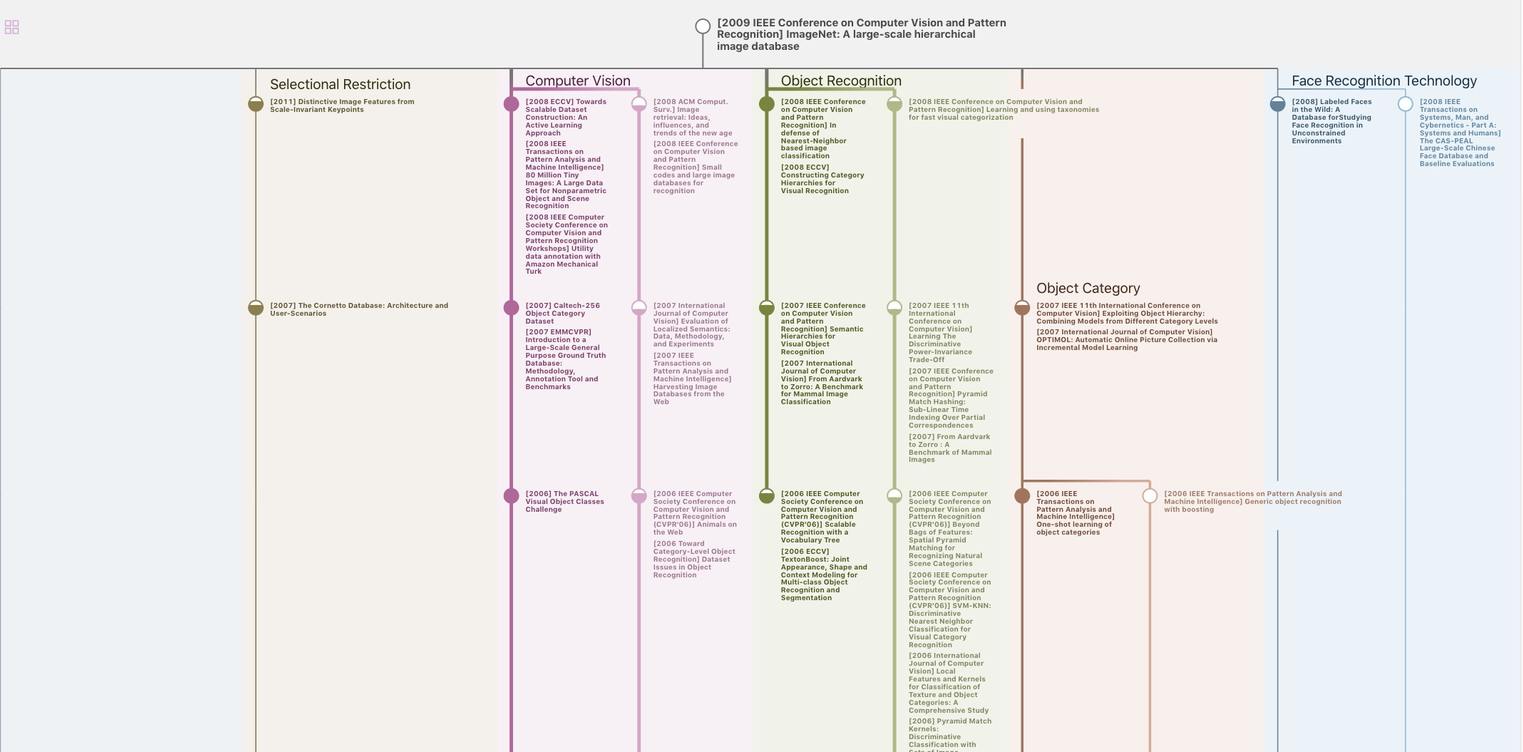
生成溯源树,研究论文发展脉络
Chat Paper
正在生成论文摘要