Parallel DBSCAN-Martingale Estimation of the Number of Concepts for Automatic Satellite Image Clustering
MULTIMEDIA MODELING (MMM 2022), PT I(2022)
摘要
The necessity of organising big streams of Earth Observation (EO) data induces the efficient clustering of image patches, deriving from satellite imagery, into groups. Since the different concepts of the satellite image patches are not known a priori, DBSCAN-Martingale can be applied to estimate the number of the desired clusters. In this paper we provide a parallel version of the DBSCAN-Martingale algorithm and a framework for clustering EO data in an unsupervised way. The approach is evaluated on a benchmark dataset of Sentinel-2 images with ground-truth annotation and is also implemented on High Performance Computing (HPC) infrastructure to demonstrate its scalability. Finally, a cost-benefit analysis is conducted to find the optimal selection of reserved nodes for running the proposed algorithm, in relation to execution time and cost.
更多查看译文
关键词
Density-based clustering, Image clustering, High Performance Computing
AI 理解论文
溯源树
样例
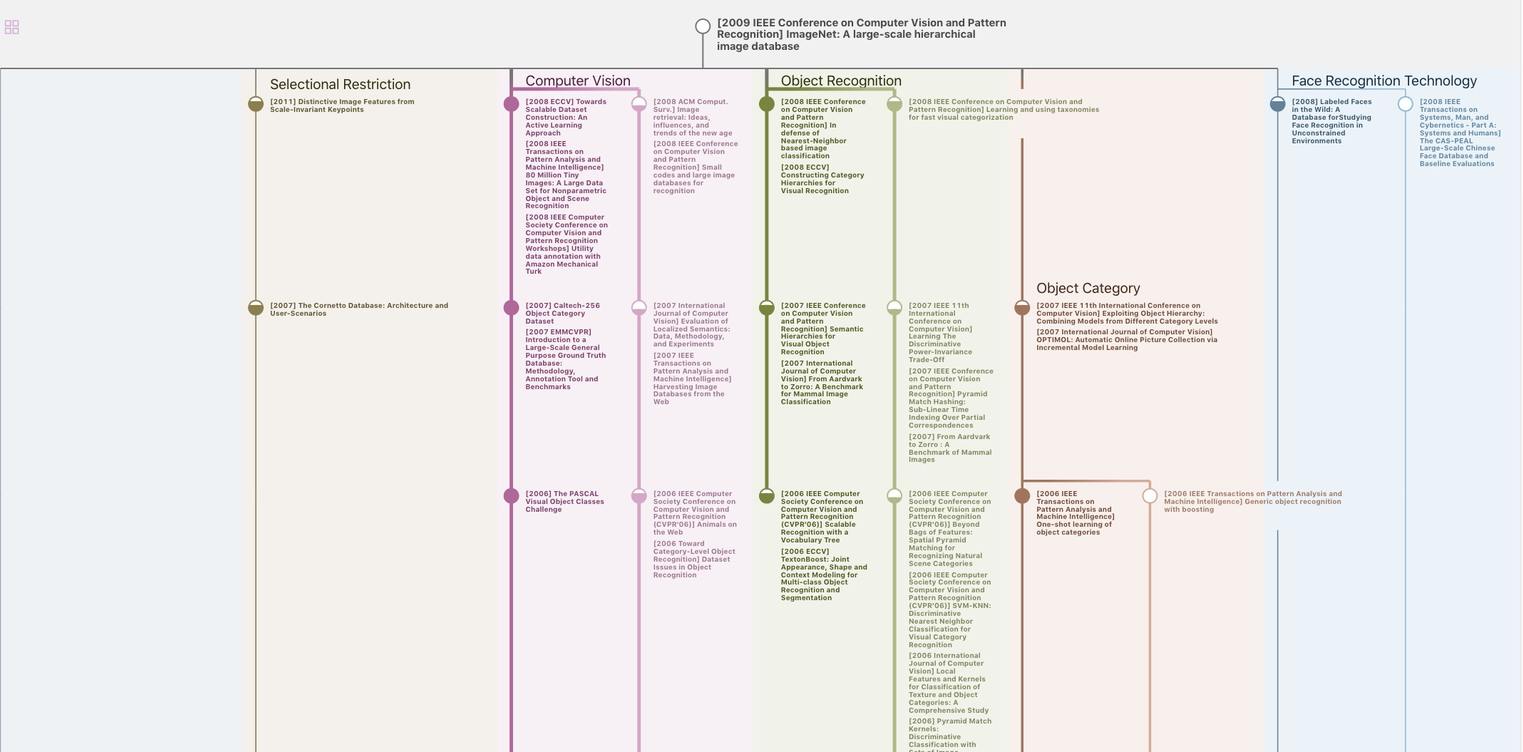
生成溯源树,研究论文发展脉络
Chat Paper
正在生成论文摘要