Multiple attentional path aggregation network for marine object detection
Applied Intelligence(2022)
摘要
Marine target detection is a challenging task because degraded underwater images cause unclear targets. Furthermore, marine targets are small in size and tend to live together. The popular object detection methods perform poorly in marine target detection. Thus, this paper proposes a novel multiple attentional path aggregation network named APAN to improve performance on marine object detection. Firstly, we design a path aggregation network structure which brings features from backbone network to bottom-up path augmentation. Each feature map is enhanced by the lower layer through the bottom-up downsampling pathway and incorporates the features from top-down upsampling layers. Specifically, the last layer fuses feature map from backbone network which enhances the semantic features and improve the ability of feature extraction. Then, a multi-attention which combines coordinate competing attention and spatial supplement attention applies to proposed path aggregation network. Multi-attention can further improve the accuracy of multiple marine object detection. Finally, a double transmission underwater image enhancement algorithm is proposed to enhance the underwater image datasets. The experiments show our method achieves 79.6 % mAP in underwater image datasets and 79.03 % mAP in enhanced underwater image datasets. Meanwhile, our method achieves 81.5 % mAP in PASCAL VOC datasets. In addition, we also applly the method to the underwater robot. The experiments show our method achieves good performance compared with popular object detection methods. The source code is publicly available at https://github.com/yhf2022/APAN .
更多查看译文
关键词
Marine target detection, Path aggregation network, Multi-attention, Underwater image enhancement
AI 理解论文
溯源树
样例
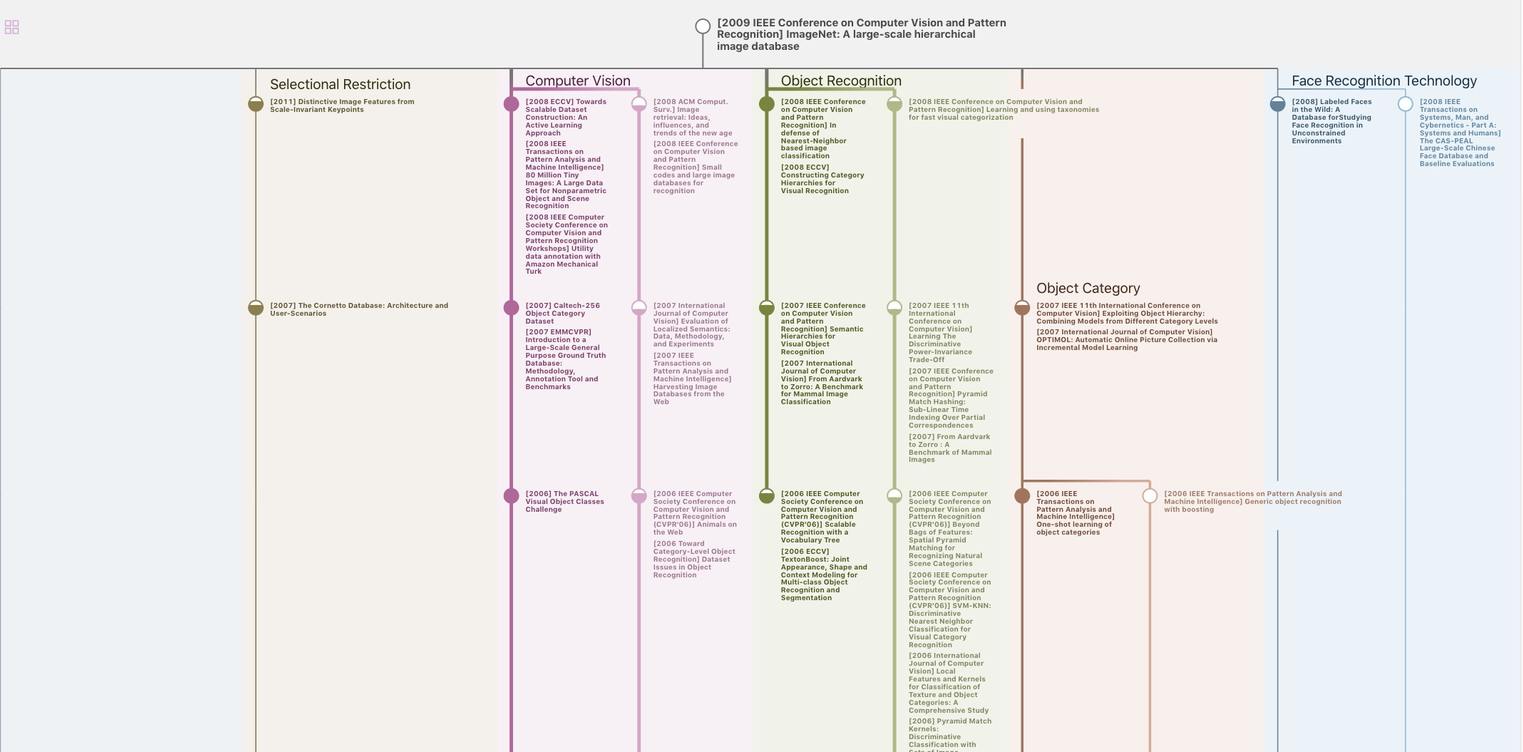
生成溯源树,研究论文发展脉络
Chat Paper
正在生成论文摘要