Prediction of shield jamming risk for double-shield TBM tunnels based on numerical samples and random forest classifier
ACTA GEOTECHNICA(2022)
摘要
Currently, various machine learning (ML) techniques have been developed to solve geotechnical engineering problems. However, the lack of representative field samples limits the application of ML models. A shield jamming risk prediction method based on numerical samples and random forest (RF) classifier is proposed. The database with samples of different shield jamming risk levels is established by numerical simulation of the TBM construction process. By setting different values and combinations, seven influencing parameters, i.e., advance rate, overcut, elastic modulus, tensile strength, in situ stress, maximum thrust and friction coefficient are considered. The shield jamming risk level is determined according to the ratio of the total friction force to TBM residual thrust. Feature importance analysis indicates that elastic modulus, overcut and in-situ stress are the major influencing factors of shield jamming. Based on the labeled database, the RF model integrating multiple decision trees is established to capture the complex relationship between shield jamming risk and different influencing factors. The trained model has shown a good prediction performance on test set, and the prediction results of six field instances in DXL tunnel are in good agreement with the actual shield jamming situation. Compared with other conventional classifiers, i.e., support vector machine (SVM), k-nearest neighbors (KNN), decision tree (DT) and logistic regression (LR), the proposed FR classifier has stronger prediction accuracy and generalization ability. Additionally, the influence of sample numbers and sample imbalance is discussed.
更多查看译文
关键词
Double-shield TBM, Machine learning, Numerical simulation, Random forest classifier, Shield jamming risk
AI 理解论文
溯源树
样例
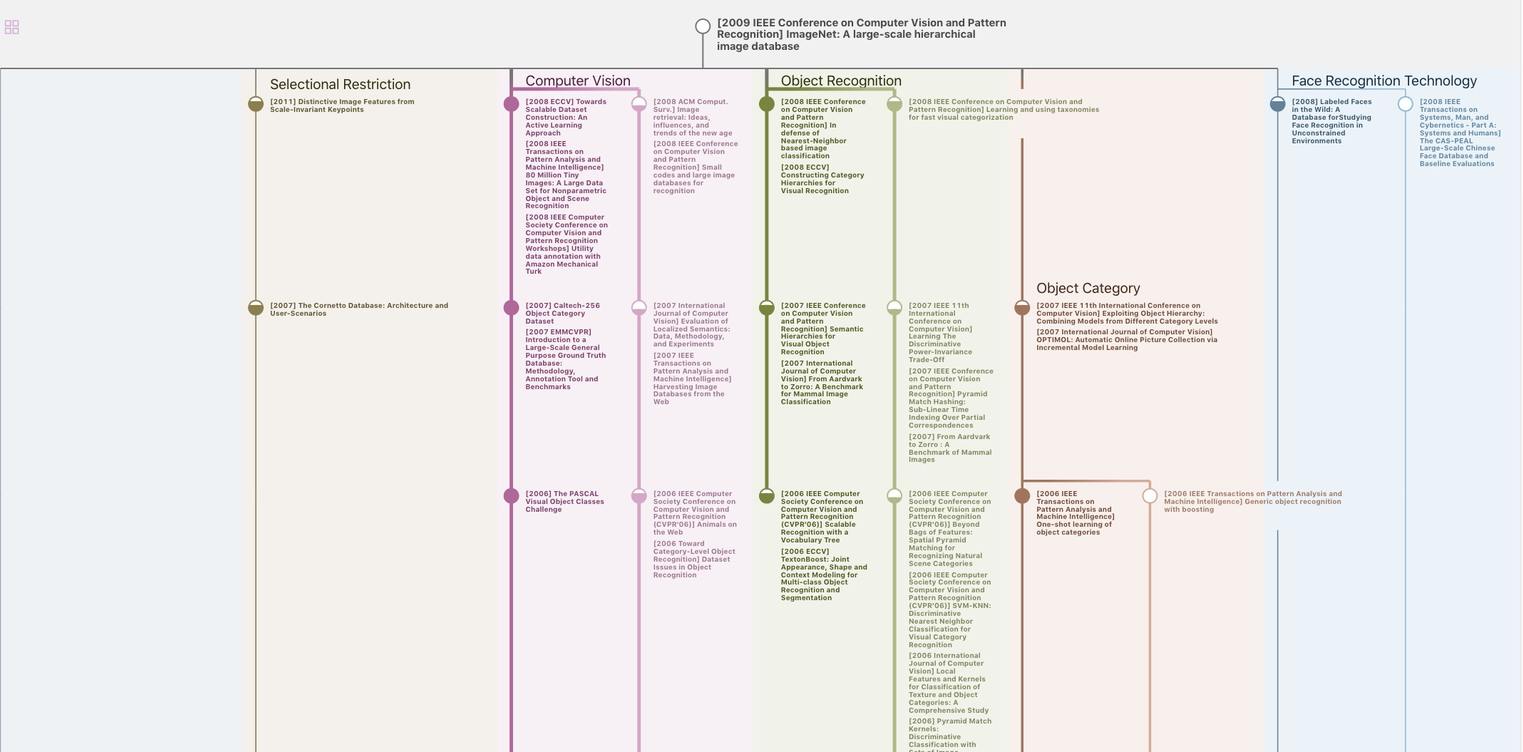
生成溯源树,研究论文发展脉络
Chat Paper
正在生成论文摘要