Biclustering via structured regularized matrix decomposition
Statistics and Computing(2022)
摘要
Biclustering is a machine learning problem that deals with simultaneously clustering of rows and columns of a data matrix. Complex structures of the data matrix such as overlapping biclusters have challenged existing methods. In this paper, we first provide a unified formulation of biclustering that uses structured regularized matrix decomposition, which synthesizes various existing methods, and then develop a new biclustering method called BCEL based on this formulation. The biclustering problem is formulated as a penalized least-squares problem that approximates the data matrix 𝐗 by a multiplicative matrix decomposition 𝐔𝐕^T with sparse columns in both 𝐔 and 𝐕 . The squared ℓ _1,2 -norm penalty, also called the exclusive Lasso penalty, is applied to both 𝐔 and 𝐕 to assist identification of rows and columns included in the biclusters. The penalized least-squares problem is solved by a novel computational algorithm that combines alternating minimization and the proximal gradient method. A subsampling based procedure called stability selection is developed to select the tuning parameters and determine the bicluster membership. BCEL is shown to be competitive to existing methods in simulation studies and an application to a real-world single-cell RNA sequencing dataset.
更多查看译文
关键词
Biclustering,Squared ℓ _1,2 -norm,Structured sparsity,Stability selection
AI 理解论文
溯源树
样例
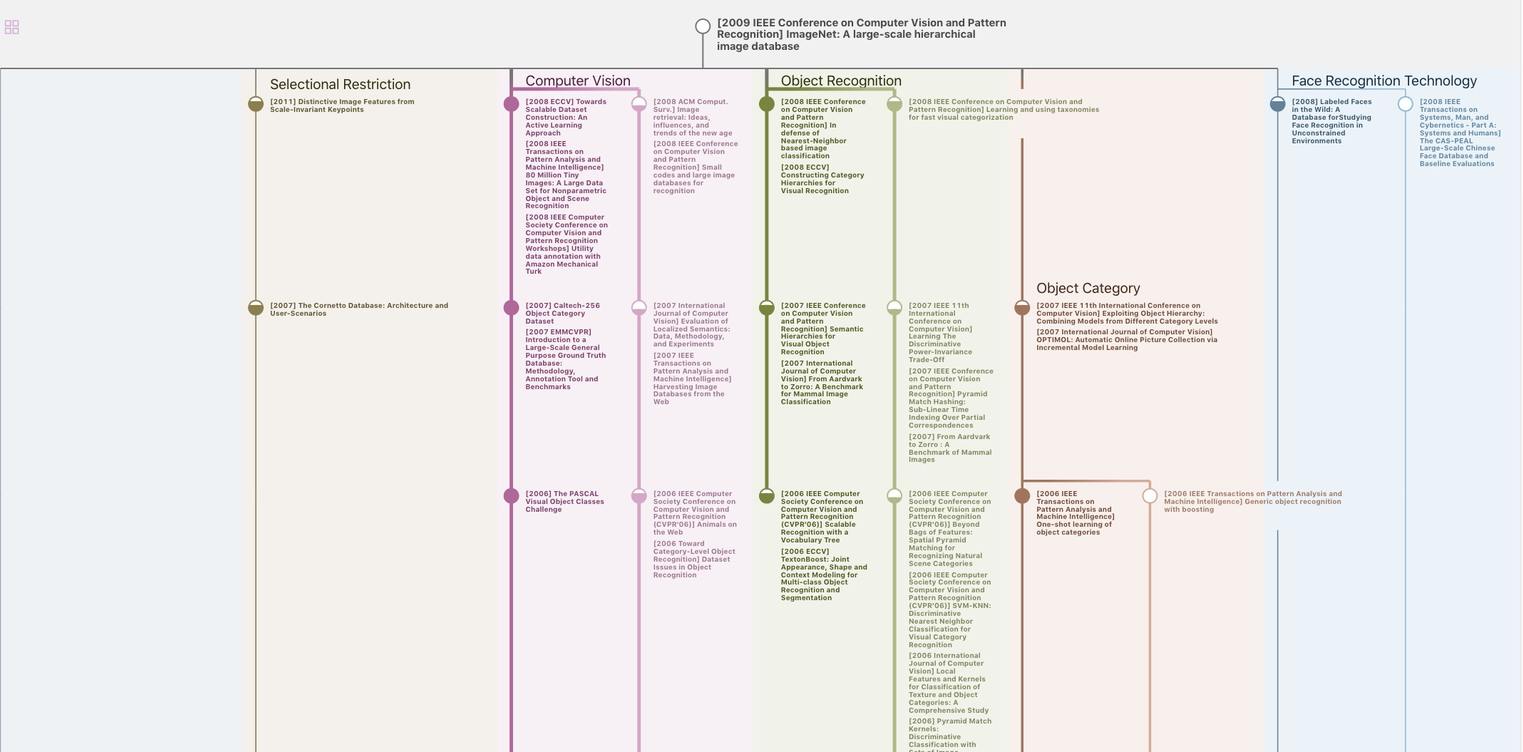
生成溯源树,研究论文发展脉络
Chat Paper
正在生成论文摘要