Improved Stereo Matching Accuracy Based on Selective Backpropagation and Extended Cost Volume
International Journal of Control, Automation and Systems(2022)
摘要
Stereo matching using convolutional neural networks employs three-dimensional (3D) convolutions to improve the matching accuracy. However, such 3D convolutions also increase the stereo matching time. Therefore, some studies have shortened the matching time by reducing the size of the cost volumes used for a 3D convolution. In this paper, we apply a coarse-to-fine approach to reduce the matching time and improve the matching accuracy. This approach controls the size of the cost volume by adjusting the number of disparity planes while maintaining the spatial resolution. However, the inaccuracies obtained in the coarse module lead to an incorrect training of the network. We propose two methods for preventing such incorrect training. The first method is selective backpropagation, which excludes pixels with a large difference between the predicted disparity and the ground-truth disparity from the learning process in the fine module. The second method involves the construction of a new extended cost volume, which uses three different feature maps specialized for each of the three cost volumes: concatenation volume, correlation volume, and absolute difference volume. We conducted various experiments using three standard datasets for two existing networks and showed that the proposed algorithm improves the disparity prediction performance.
更多查看译文
关键词
Coarse-to-fine approach, extended cost volume, selective backpropagation, stereo matching
AI 理解论文
溯源树
样例
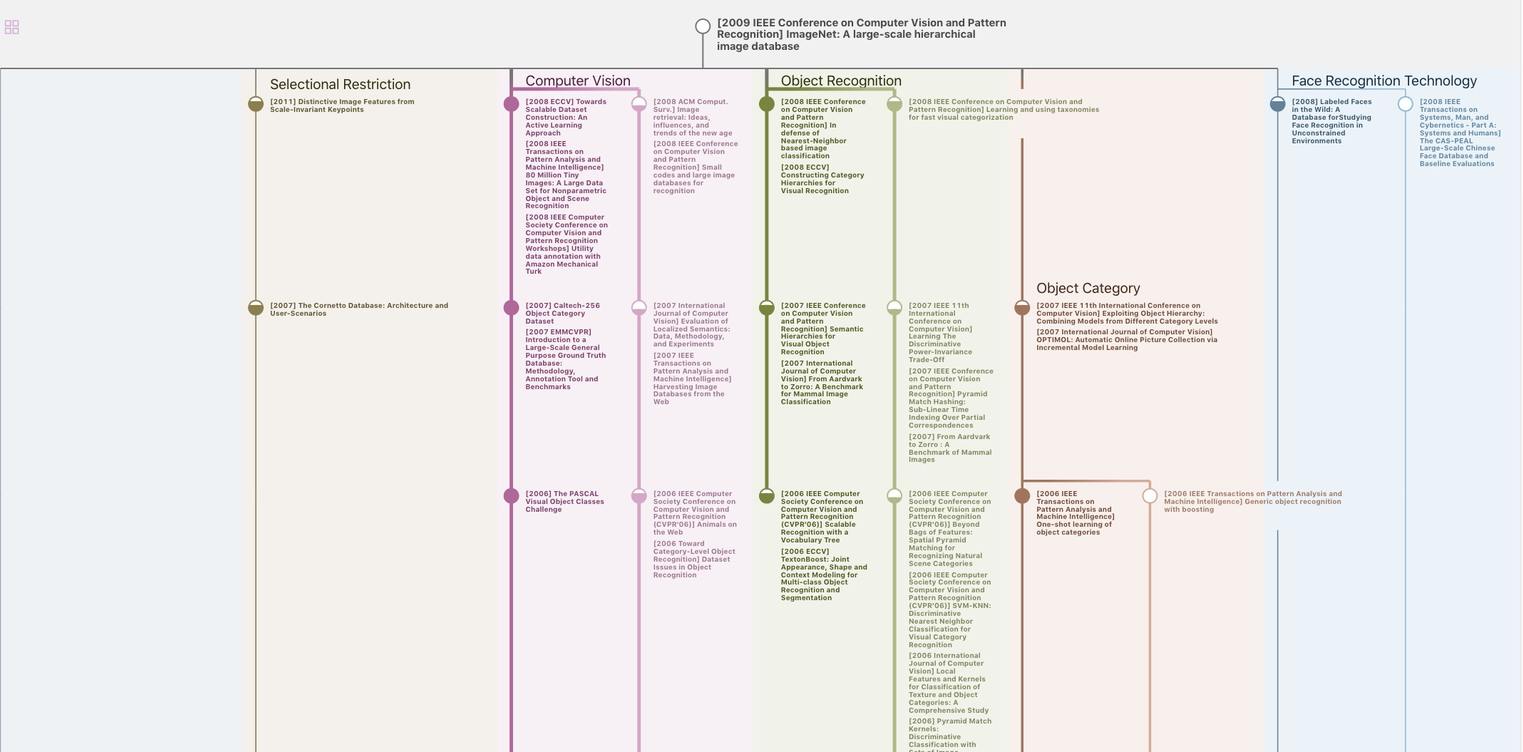
生成溯源树,研究论文发展脉络
Chat Paper
正在生成论文摘要