Turning traffic volume imputation for persistent missing patterns with GNNs
Applied Intelligence(2022)
摘要
Traffic volume data at fixed detectors are of great importance to track the time-varying states of urban traffic, and the volume of each turning movement (i.e., continuing straight, turning left, turning right) in an intersection plays a significant role in traffic control. It is a difficult issue to determine the complete road network volume due to the limited coverage of detector deployment. Existing traffic volume imputation methods seem to be hardly tractable to address this persistent missing data problem of fixed detectors because they mainly function by utilizing spatiotemporal patterns of historical data to address the problem of intermittent missing traffic data collected from probe vehicles. Considering the unattainable temporal information of the target movement, this paper aims to develop an end-to-end graph neural network-based imputation approach to impute traffic volume data with persistent missing patterns. We regard the road network as a directed graph with each turning movement as a node and employ a meta-learning-based graph attention mechanism with graph embedding in multiple spatial granularities to fully extract the relationship between the target node and its neighbours. In addition, a multitask learning mechanism is designed to improve the accuracy of the imputation results by setting auxiliary tasks according to the volume conservation constraints. The proposed approach has been validated with extensive simulation experiments and applied to field tests for Shenzhen and Shanghai, China, with considerable accuracy and reliability, satisfying the demand for traffic control. To the best of our knowledge, this is the first time that traffic volume has been imputed for persistent missing patterns with a graph neural network-based method.
更多查看译文
关键词
Traffic volume imputation,Volume conservation,Graph neural network
AI 理解论文
溯源树
样例
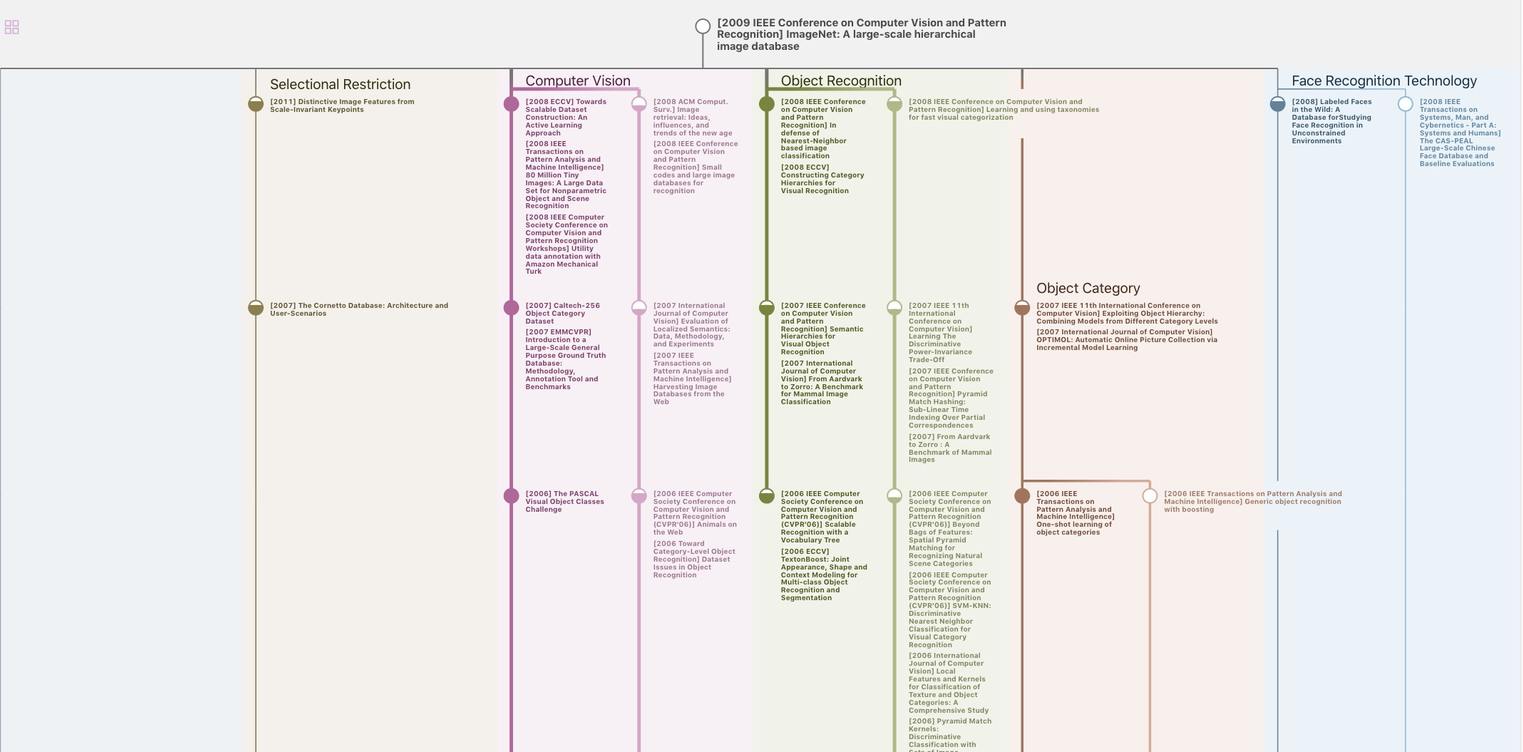
生成溯源树,研究论文发展脉络
Chat Paper
正在生成论文摘要