MF-GAN: Multi-conditional Fusion Generative Adversarial Network for Text-to-Image Synthesis
MULTIMEDIA MODELING (MMM 2022), PT I(2022)
摘要
The performance of text-to-image synthesis has been significantly boosted accompanied by the development of generative adversarial network (GAN) techniques. The current GAN-based methods for text-to-image generation mainly adopt multiple generator-discriminator pairs to explore the coarse/fine-grained textual content (e.g., words and sentences); however, they only consider the semantic consistency between the text-image pair. One drawback of such a multi-stream structure is that it results in many heavyweight models. In comparison, the single-stream counterpart bears the weakness of insufficient use of texts. To alleviate the above problems, we propose a Multi-conditional Fusion GAN (MF-GAN) to reap the benefits of both the multi-stream and the single-stream methods. MF-GAN is a single-stream model but achieves the utilization of both coarse and fine-grained textual information with the use of conditional residual block and dual attention block. More specifically, the sentence and word features are repeatedly inputted into different model stages for textual information enhancement. Furthermore, we introduce a triple loss to close the visual gap between the synthesized image and its positive image and enlarge the gap to its negative image. To thoroughly verify our method, we conduct extensive experiments on two benchmarked CUB and COCO datasets. Experimental results show that the proposed MF-GAN outperforms the state-of-the-art methods.
更多查看译文
关键词
Text-to-Image, GAN, Triplet loss
AI 理解论文
溯源树
样例
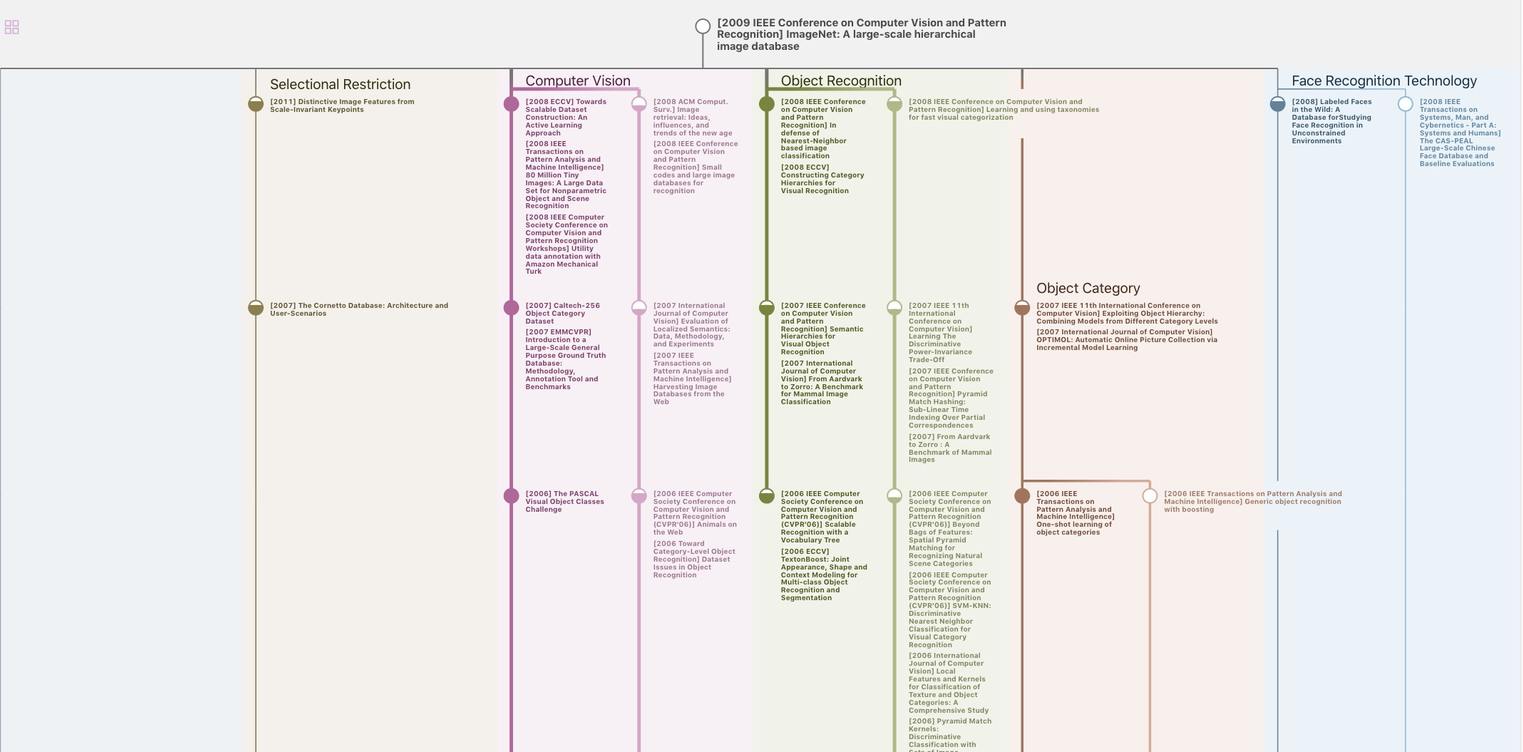
生成溯源树,研究论文发展脉络
Chat Paper
正在生成论文摘要