Lightweight edge-attention network for surface-defect detection of rubber seal rings
MEASUREMENT SCIENCE AND TECHNOLOGY(2022)
摘要
Detection of surface defects is an essential step to ensure the quality and reliability of rubber seal-ring products. Machine vision inspection methods have received attention from engineers because they are beneficial in terms of the detection speed or detection accuracy. It is important to simultaneously guarantee high speed and high accuracy of defect detection in practice production. Considering this problem, in this paper, we propose a YOLO-based (You Only Look Once) lightweight neural network with edge-attention fusion for defect detection of rubber rings. First, an edge-attention block is integrated into the network to improve the detection accuracy. The edge images are weighted with attention weights and summarized with feature maps in the block. Second, an involution-based feature pyramid structure is proposed to reduce the network size and computational cost by using a new involution operator, instead of a convolution operator, at the bottleneck of the feature pyramid. Comparison of the proposed network with popular YOLO networks was performed based on a rubber-ring-image dataset with 138 images. The network achieved the highest detection accuracy and fastest speed among selected lightweight networks, including YOLOv3-tiny, YOLOv5-s, and YOLOX-s. Moreover, compared with the large and highly accurate YOLOv3-spp network, the network size decreased by 93.45% and the computational cost decreased by 91.46%, while average precision only decreased by 3.41%. It can be concluded that the YOLO-based lightweight neural network reaches a good balance between detection speed and detection accuracy.
更多查看译文
关键词
edge-attention block, neural network, YOLO, defect detection, rubber seal ring
AI 理解论文
溯源树
样例
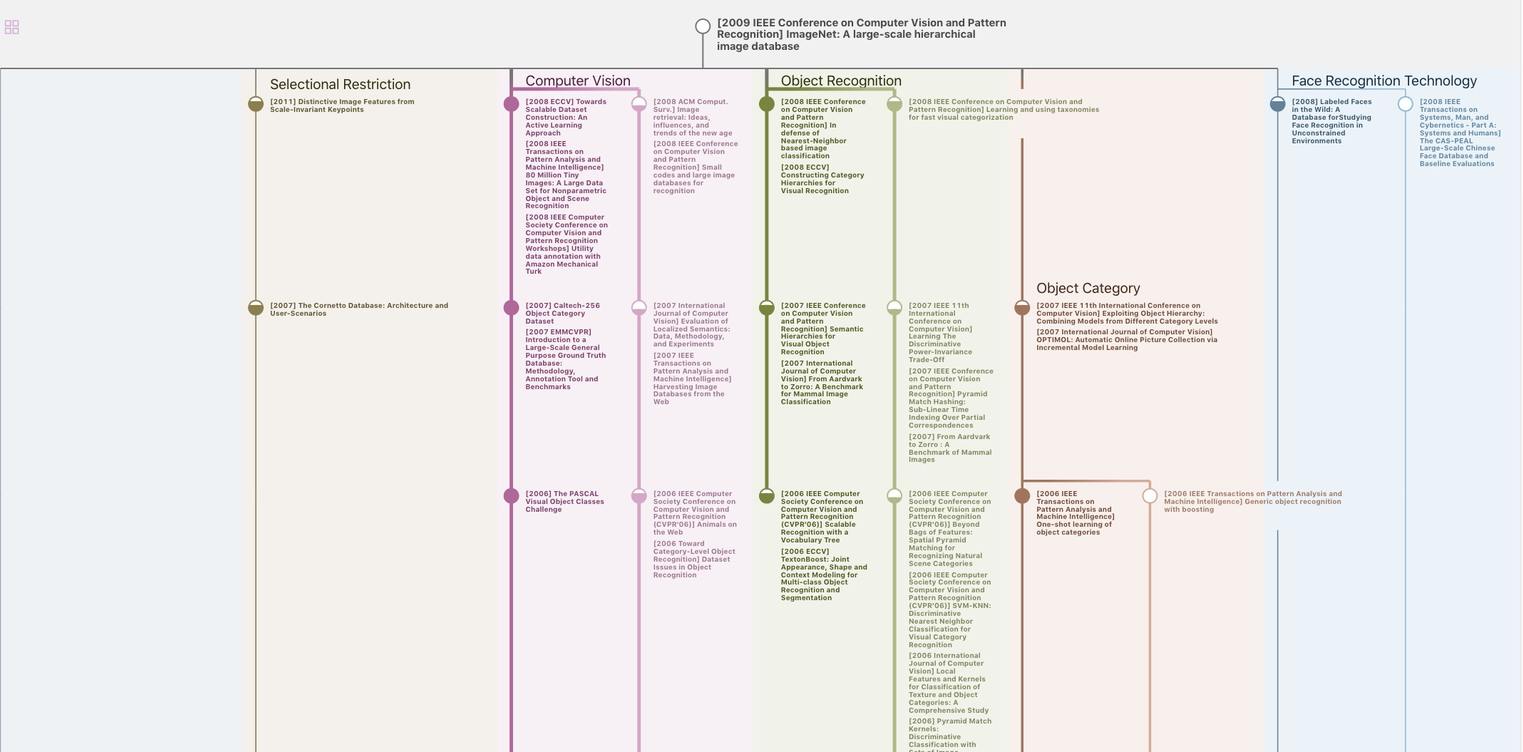
生成溯源树,研究论文发展脉络
Chat Paper
正在生成论文摘要