Feedback-Guided Attributed Graph Embedding for Relevant Video Recommendation
MACHINE LEARNING AND KNOWLEDGE DISCOVERY IN DATABASES: APPLIED DATA SCIENCE TRACK, ECML PKDD 2020, PT IV(2021)
摘要
Representation learning on graphs, as alternatives to traditional feature engineering, has been exploited in many application domains, ranging from e-commerce to computational biology. However, generating satisfactory video embeddings and putting them into practical use to improve the performance of recommendation tasks remains a challenge. In this paper, we present a video embedding approach named Equuleus, which learns video embeddings from user interaction behaviors. In Equuleus, we carefully incorporate user behavior characteristics into the construction of the video graph and the generation of node sequences. To accurately quantify the contributions of different attributes to embeddings, we propose a particular attributed encoder network, which employs an attention mechanism to aggregate different attributes in a distinguishable way. Moreover, we also leverage the user feedback as a guide to correct the generation of embeddings. Video embeddings generated by Equuleus have been used for relevant recommendation of videos in MX Player. Based on real data from MX Player, extensive offline experiments and online A/B test are conducted. Both experimental results and online CTRs illustrate that Equuleus can generate high-quality video embeddings and it can work effectively in a real-world production environment.
更多查看译文
关键词
Recommender system, Representation learning, Graph embedding, User behavior mining
AI 理解论文
溯源树
样例
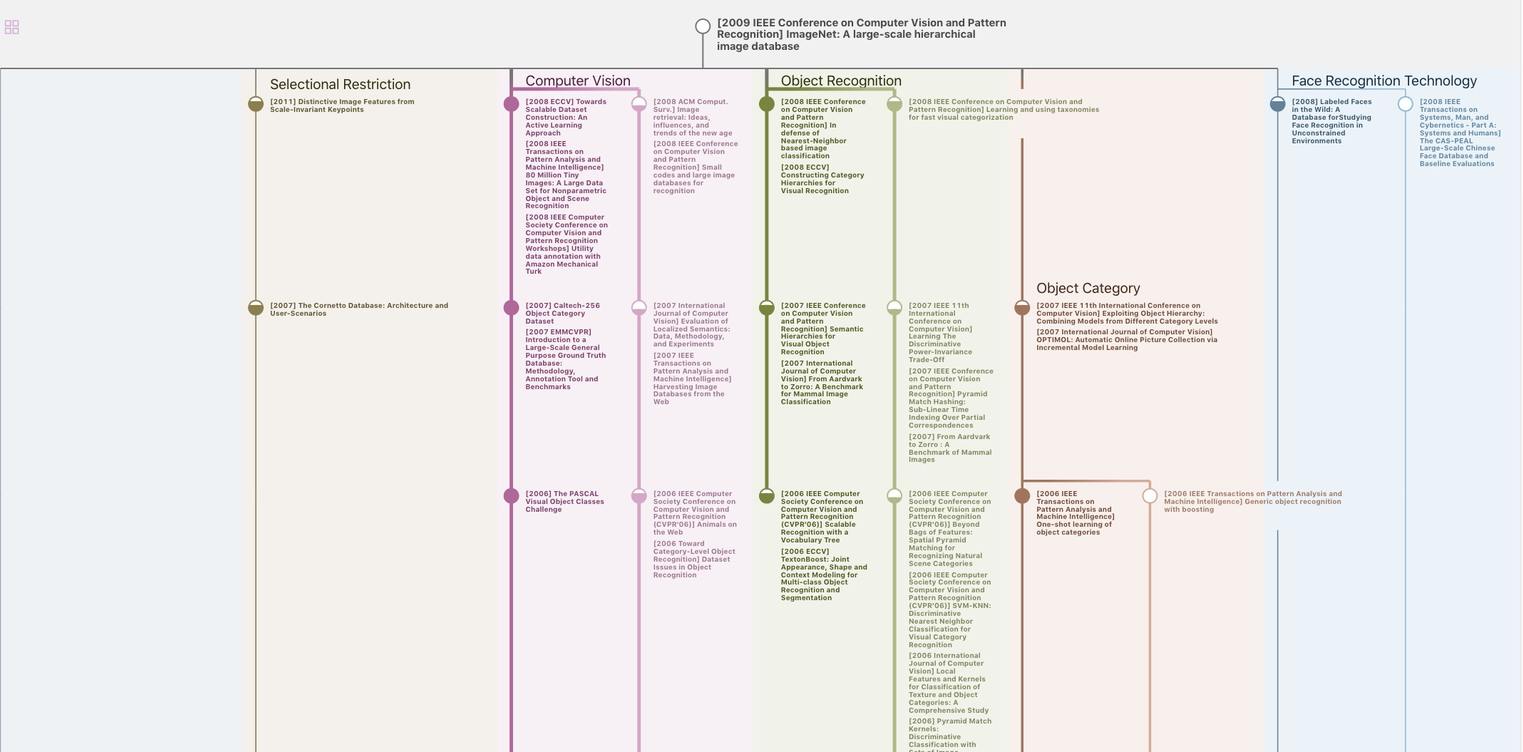
生成溯源树,研究论文发展脉络
Chat Paper
正在生成论文摘要