Leveraging Expert Knowledge for Label Noise Mitigation in Machine Learning
APPLIED SCIENCES-BASEL(2021)
摘要
In training-based Machine Learning applications, the training data are frequently labeled by non-experts and expose substantial label noise which greatly alters the training models. In this work, a novel method for reducing the effect of label noise is introduced. The rules are created from expert knowledge to identify the incorrect non-expert training data. Using the gradient descent algorithm, the violating data samples are weighted less to mitigate their effects during model training. The proposed method is applied to the image classification problem using Manga109 and CIFAR-10 dataset. The experiments show that when the noise level is up to 50% our proposed method significantly increases the accuracy of the model compared to conventional learning methods.
更多查看译文
关键词
prior knowledge, noise datasets, label noise, weighting training data
AI 理解论文
溯源树
样例
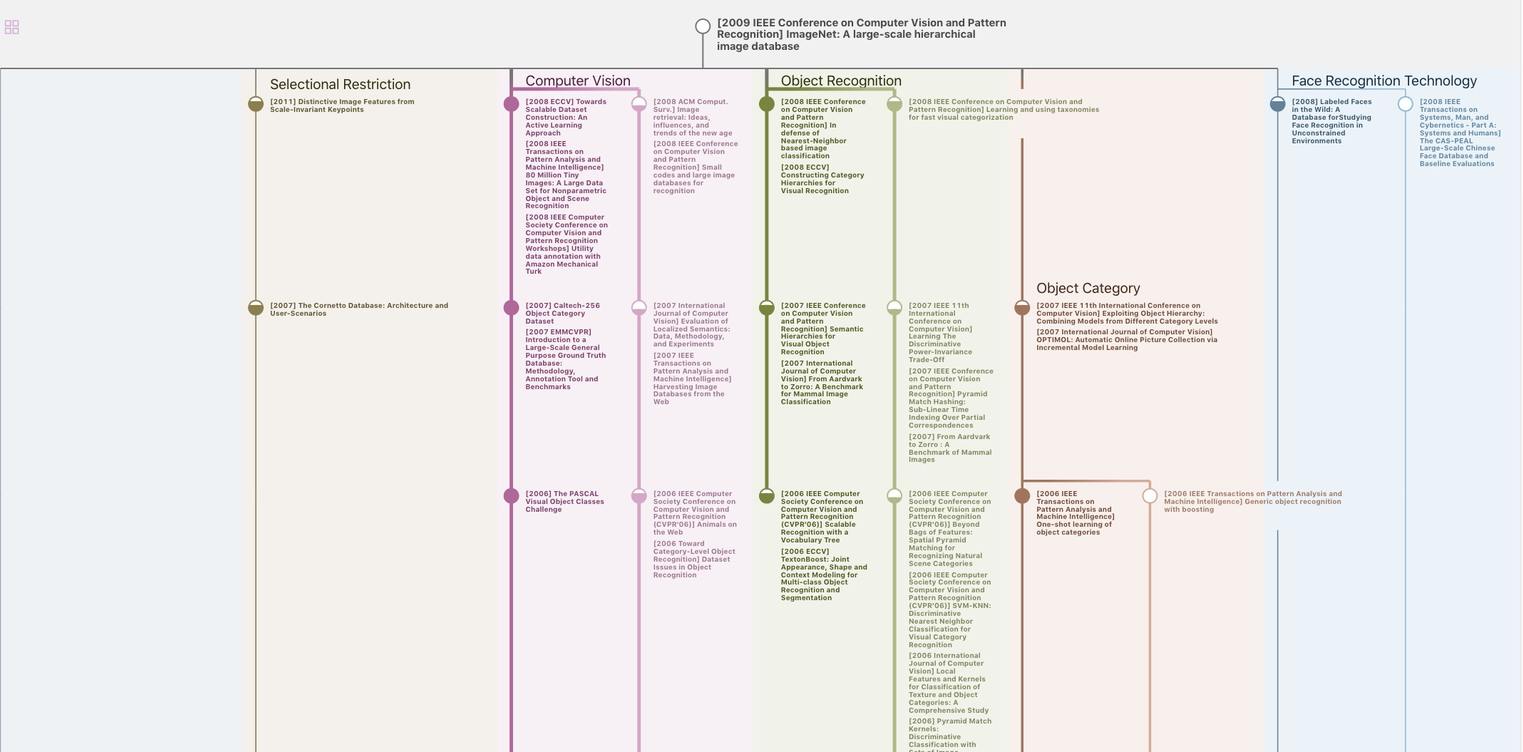
生成溯源树,研究论文发展脉络
Chat Paper
正在生成论文摘要