Hyperspectral Image Abnormal Target Detection Based on End-Member Extraction and Low-Rank and Sparse Matrix Decomposition
LASER & OPTOELECTRONICS PROGRESS(2021)
Abstract
In this study, to suppress the interference of mixed pixels and noise in hyperspectralimages (HSI) on abnormal target detection in a complex background and fully extract and utilize the spectral and spatial features of HSI, a HSI abnormal target detection algorithm based on end-member extraction and low-rank and sparse matrix decomposition is proposed. First, optimal fractional-order Fourier transform is applied to the original HSI. Then, the sequential maximum angle convex cone algorithm is used to extract the endmembers of the transformed HSI; subsequently, the end members and corresponding abundance matrix are obtained. The abundance matrix is decomposed into a low-rank background component and an abnormal component with sparse characteristics using the solution of the low-rank and sparse matrix decomposition method with row constraints. Finally, the background covariance matrix is constructed and abnormal targets are detected using the Mahalanobis distance. Experimental results show that the proposed algorithm exhibits good performance in HSI abnormal target detection.
MoreTranslated text
Key words
remote sensing, hyperspectral images, sequential maximum angle convex cone, optimal fractional-order Fourier transform, low-rank and sparse matrix decomposition, abnormal target detection
AI Read Science
Must-Reading Tree
Example
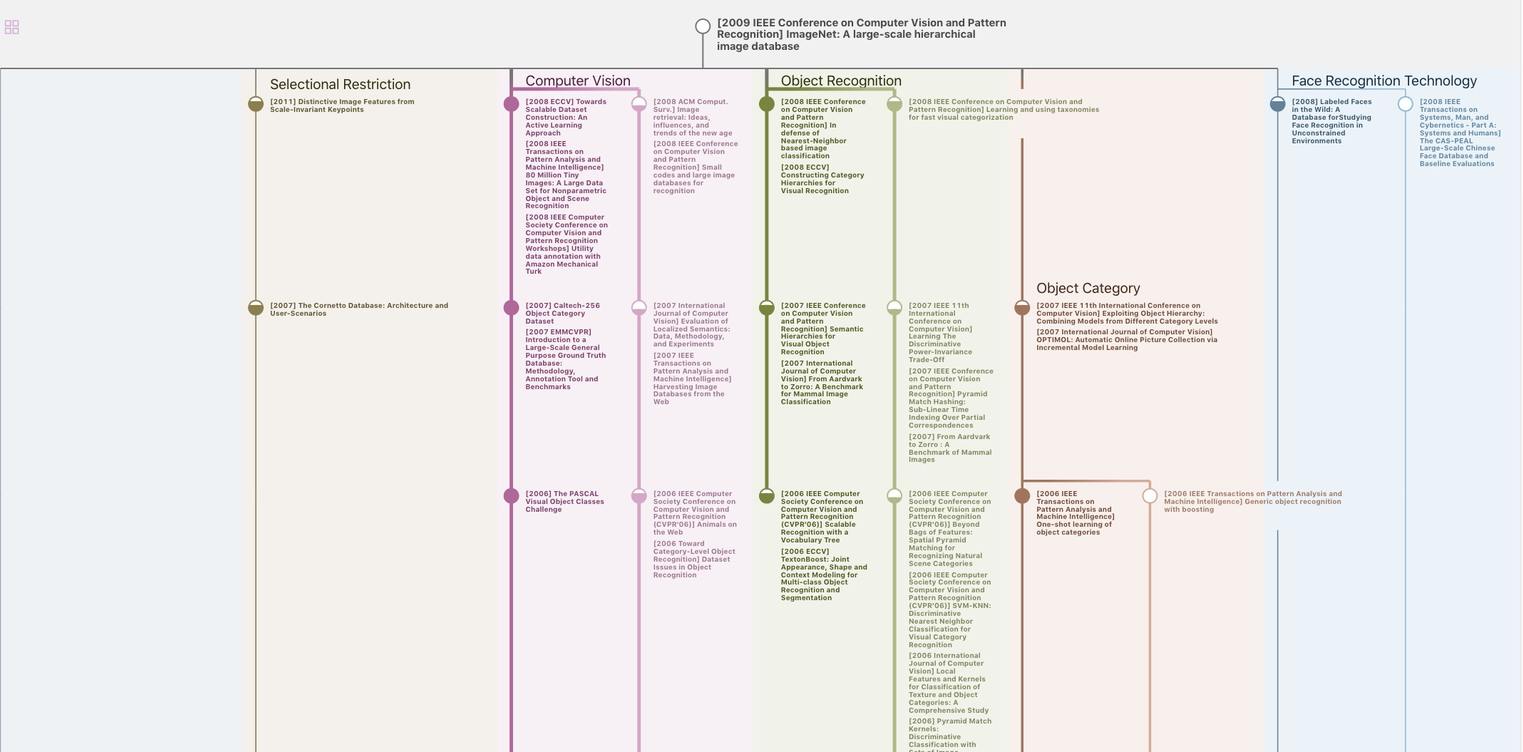
Generate MRT to find the research sequence of this paper
Chat Paper
Summary is being generated by the instructions you defined