Multi-Spectral Color Data Dimension Reduction Model Research Based on Sparse Representation
LASER & OPTOELECTRONICS PROGRESS(2021)
Abstract
In order to solve the problem of real color reproduction in real three-dimensional images of multispectral lidar system, a dimensionality reduction method of multi-spectral color data based on sparse signal representation is proposed in this paper. This method utilizes dictionary learning and alternate update of sparse coding to correct spectral errors in an iterative way. Root mean square error of the experimental results show that the proposed method is the principal component analysis is reduced by 35.29%, the average of spectrum fitting coefficient reaches more than 99.8 %, and the average of chromaticity accuracy than principal component analysis on average increases by 70. 23%, under different light source observation conditions still can maintain the stability of color, its reconstruction precision is better than that of the principal component analysis. The sparse representation can recover high-dimensional sparse signals through low-dimensional observation vectors. This method can accurately recover a large number of test samples from a relatively small number of training samples, which improves the cost efficiency of data processing and is of great help to truly reflect the ground object information of remote sensing multi-spectral images.
MoreTranslated text
Key words
spectroscopy,sparse representation,dictionary learning,spectral reflectance,spectral dimension reduction
AI Read Science
Must-Reading Tree
Example
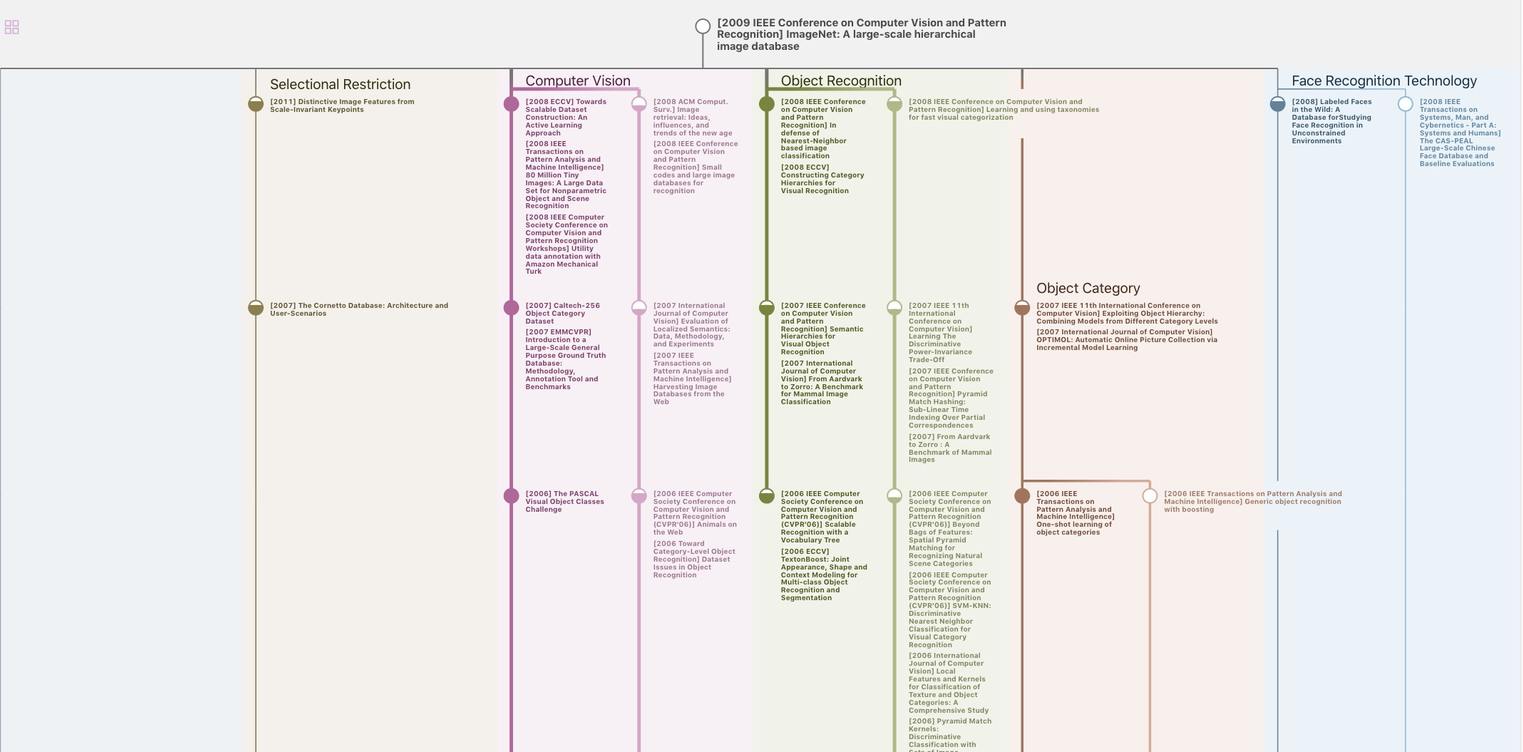
Generate MRT to find the research sequence of this paper
Chat Paper
Summary is being generated by the instructions you defined