A Mixed Ensemble Learning and Time-Series Methodology for Category-Specific Vehicular Energy and Emissions Modeling
SUSTAINABILITY(2022)
摘要
The serially-correlated nature of engine operation is overlooked in the vehicular fuel and emission modeling literature. Furthermore, enabling the calibration and use of time-series models for instrument-independent eco-driving applications requires reliable forecast aggregation procedures. To this end, an ensemble time-series machine-learning methodology is developed using data collected through extensive field experiments on a fleet of 35 vehicles. Among other results, it is found that Long Short-Term Memory (LSTM) architecture is the best fit for capturing the dynamic and lagged effects of speed, acceleration, and grade on fuel and emission rates. The developed vehicle-specific ensembles outperformed state-of-the-practice benchmark models by a significant margin and the category-specific models outscored the vehicle-specific sub-models by an average margin of 6%. The results qualify the developed ensembles to work as representatives for vehicle categories and allows them to be utilized in both eco-driving services as well as environmental assessment modules.
更多查看译文
关键词
vehicular emissions, eco-driving, recurrent neural networks, ensemble learning
AI 理解论文
溯源树
样例
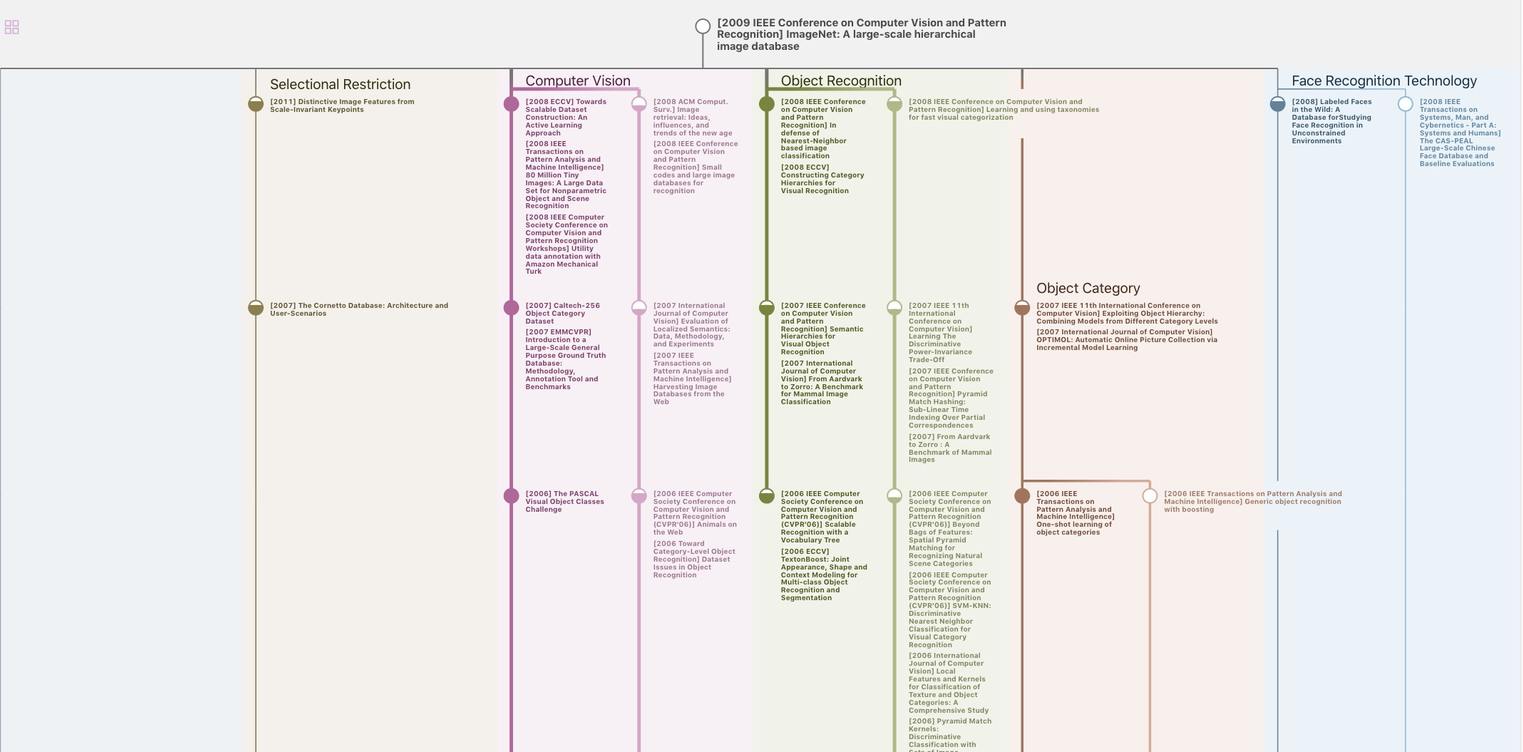
生成溯源树,研究论文发展脉络
Chat Paper
正在生成论文摘要