InterpoNet, A brain inspired neural network for optical flow dense interpolation
30TH IEEE CONFERENCE ON COMPUTER VISION AND PATTERN RECOGNITION (CVPR 2017)(2016)
摘要
Sparse-to-dense interpolation for optical flow is a fundamental phase in the pipeline of most of the leading optical flow estimation algorithms. The current state-of-the-art method for interpolation, EpicFlow, is a local average method based on an edge aware geodesic distance. We propose a new data-driven sparse-to-dense interpolation algorithm based on a fully convolutional network. We draw inspiration from the filling-in process in the visual cortex and introduce lateral dependencies between neurons and multi-layer supervision into our learning process. We also show the importance of the image contour to the learning process. Our method is robust and outperforms EpicFlow on competitive optical flow benchmarks with several underlying matching algorithms. This leads to state-of-the-art performance on the Sintel and KITTI 2012 benchmarks.
更多查看译文
关键词
edge aware geodesic distance,sparse-to-dense interpolation algorithm,filling-in process,learning process,EpicFlow,competitive optical flow benchmarks,state-of-the-art performance,brain inspired neural network,optical flow dense interpolation,local average method,convolutional network,matching algorithms,optical flow estimation algorithms,visual cortex,multilayer supervision,image contour,KITTI 2012 benchmarks,Sintel benchmarks
AI 理解论文
溯源树
样例
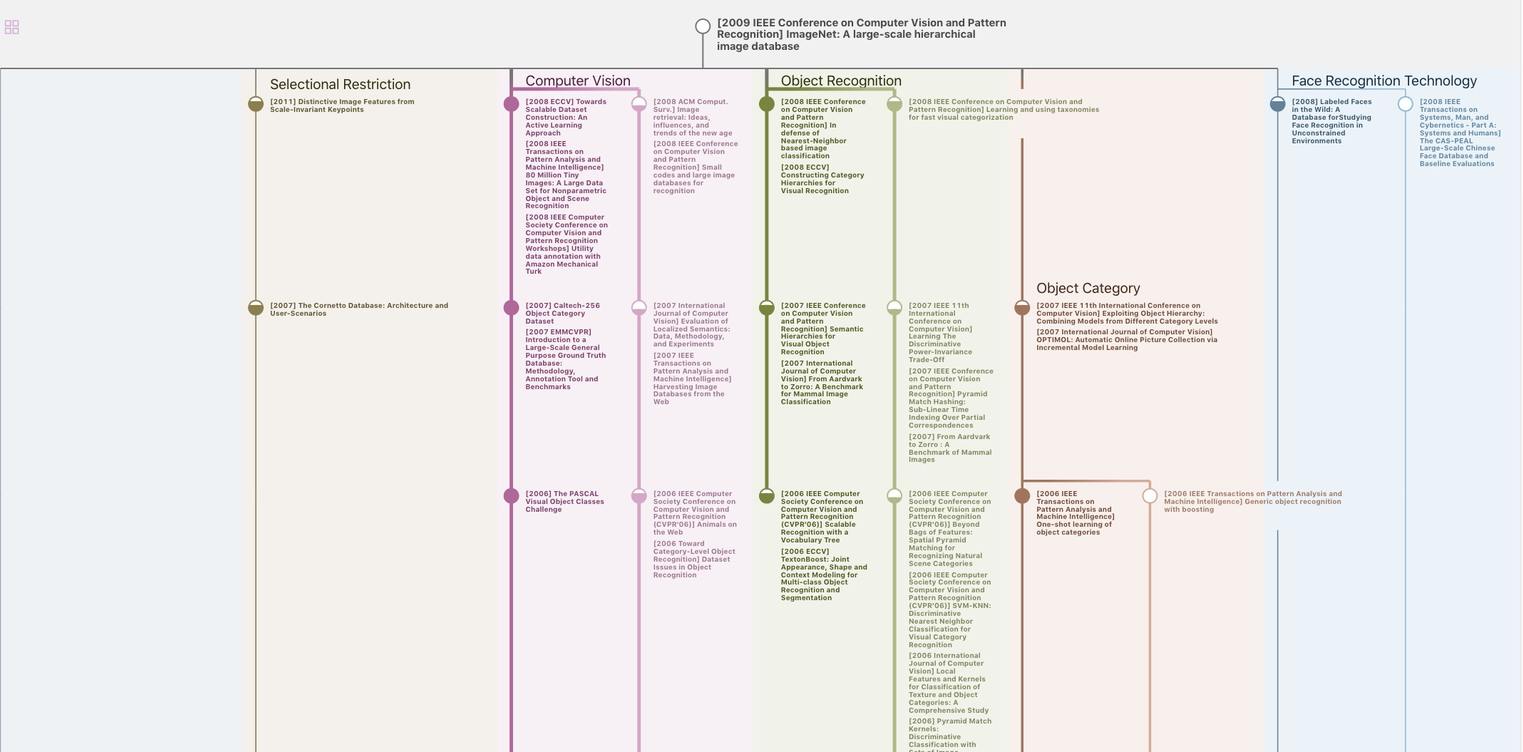
生成溯源树,研究论文发展脉络
Chat Paper
正在生成论文摘要