Deep learning approaches for imaging fluorescence correlation spectroscopy parameter estimation with limited data sets
BIOPHYSICAL JOURNAL(2022)
摘要
Deep Learning approaches using Convolutional Neural Networks (CNNs) are successfully applied in many disciplines and especially in imaging applications. CNNs provide advantages in terms of data processing time, required data size to reach a particular precision in parameter estimation, as well as the possibility of unsupervised data evaluation. Here we propose two different approaches to estimate diffusion coefficients from Imaging Fluorescence Correlation Spectroscopy (Imaging FCS) experiments and compare their performance with standard non-linear least squares (NLS) fits. The first approach estimates parameters directly from pixel intensity time traces. This has the advantage that it is not limited by the averaging performed by correlation functions, but it requires a large convolutional neural network (CNN). The second approach is applied to the autocorrelation function for each pixel. As a correlation function contains fewer points than the intensity trace, this approach requires smaller CNNs. However, to train a CNN one requires large training data sets with known properties. This can be difficult to acquire as measuring data sets takes a considerable amount of time and there is a lack of standard samples with known diffusion coefficients that span a wide range. We circumvent this problem by simulating training sets. To ensure that the data sets represent similar properties to actual experiments we test several noise models that take into account camera as well as experimental noise. The resulting CNNs for both approaches require up to 20 times less data to reach the same estimated parameter accuracy and precision as NLS fits. They thus not only improve on Imaging FCS data evaluation but also open up the possibility to reach single-digit second resolution on diffusion coefficient maps.
更多查看译文
关键词
fluorescence correlation,spectroscopy,parameter estimation,limited data sets
AI 理解论文
溯源树
样例
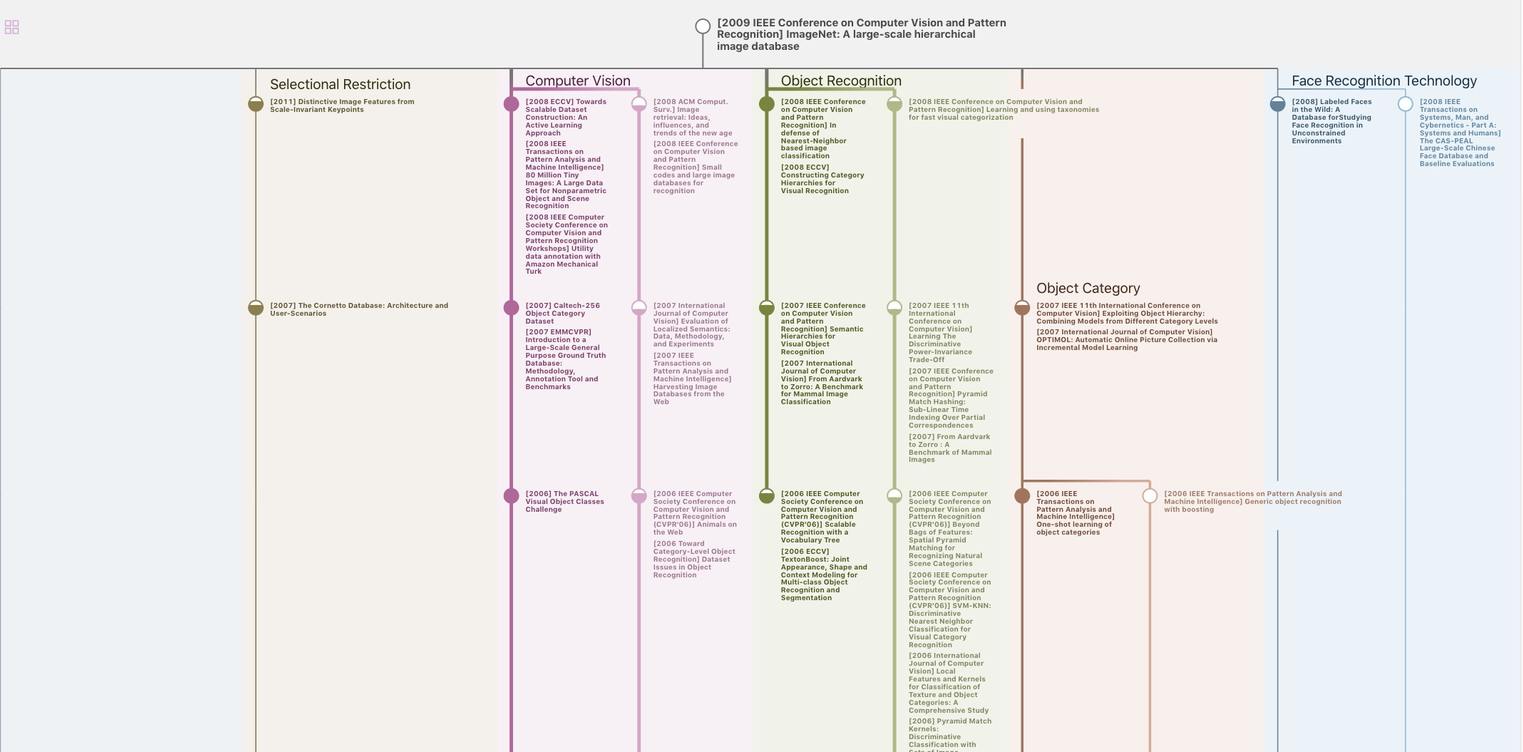
生成溯源树,研究论文发展脉络
Chat Paper
正在生成论文摘要