A new class of self-normalising LMS algorithms
ELECTRONICS LETTERS(2022)
摘要
Many researchers and practitioners make heavy use of the least mean squares (LMS) algorithm as an efficient adaptive filter suitable for a multitude of problems. Despite being versatile and efficient, a drawback of this algorithm is that the adaptation rate, i.e. step-size, has to be chosen very carefully in order to get the desired result (optimum compromise between fast adaptation and low steady state error). This choice was simplified by the invention of the normalised LMS, which bounds the step-size and guarantees convergence. However, the optimum choice of the normalisation becomes non-trivial if the system to be approximated is part of a bigger, non-trivial model, e.g. cascaded filters or linear paths followed by nonlinearities. Such cases usually require approximations or worst-case estimates in order to yield a normalised update algorithm, which might result in sub-optimal performance. To counteract this problem, a new class of LMS algorithms which automatically choose their own normalisation terms, the so-called self normalising LMS, is introduced. The simulations show that this new algorithm not only outperforms state-of-the-art solutions in terms of steady state performance in a cascaded filter scenario but also converges just as fast as all other considered algorithms.
更多查看译文
AI 理解论文
溯源树
样例
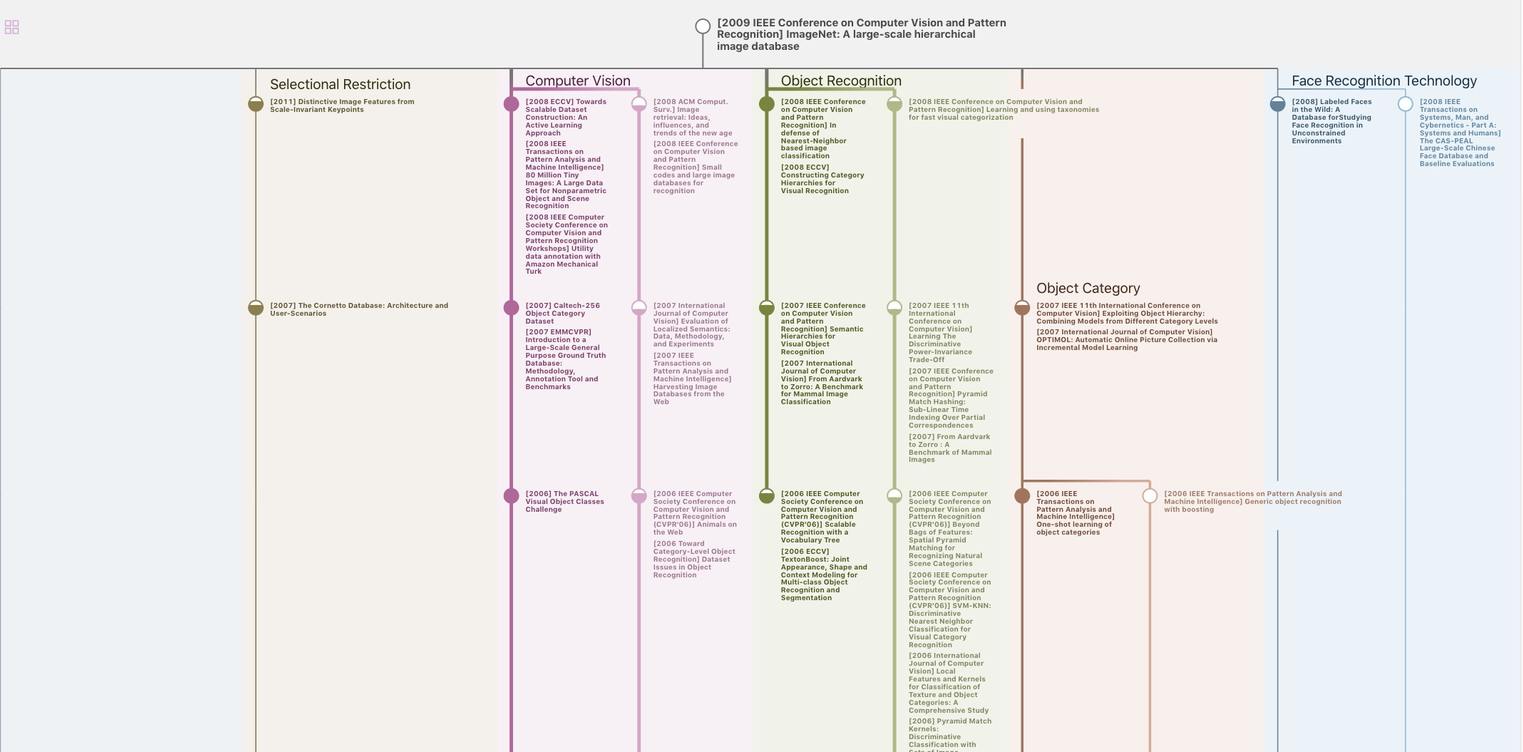
生成溯源树,研究论文发展脉络
Chat Paper
正在生成论文摘要