Fast Path Planning for Long-Range Planetary Roving Based on a Hierarchical Framework and Deep Reinforcement Learning
AEROSPACE(2022)
摘要
The global path planning of planetary surface rovers is crucial for optimizing exploration benefits and system safety. For the cases of long-range roving or obstacle constraints that are time-varied, there is an urgent need to improve the computational efficiency of path planning. This paper proposes a learning-based global path planning method that outperforms conventional searching and sampling-based methods in terms of planning speed. First, a distinguishable feature map is constructed through a traversability analysis of the extraterrestrial digital elevation model. Then, considering planning efficiency and adaptability, a hierarchical framework consisting of step iteration and block iteration is designed. For the planning of each step, an end-to-end step planner named SP-ResNet is proposed that is based on deep reinforcement learning. This step planner employs a double-branch residual network for action value estimation, and is trained over a simulated DEM map collection. Comparative analyses with baselines demonstrate the prominent advantage of our method in terms of planning speed. Finally, the method is verified to be effective on real lunar terrains using CE2TMap2015.
更多查看译文
关键词
planetary rovers, global path planning, deep reinforcement learning, hierarchical framework, computational efficiency
AI 理解论文
溯源树
样例
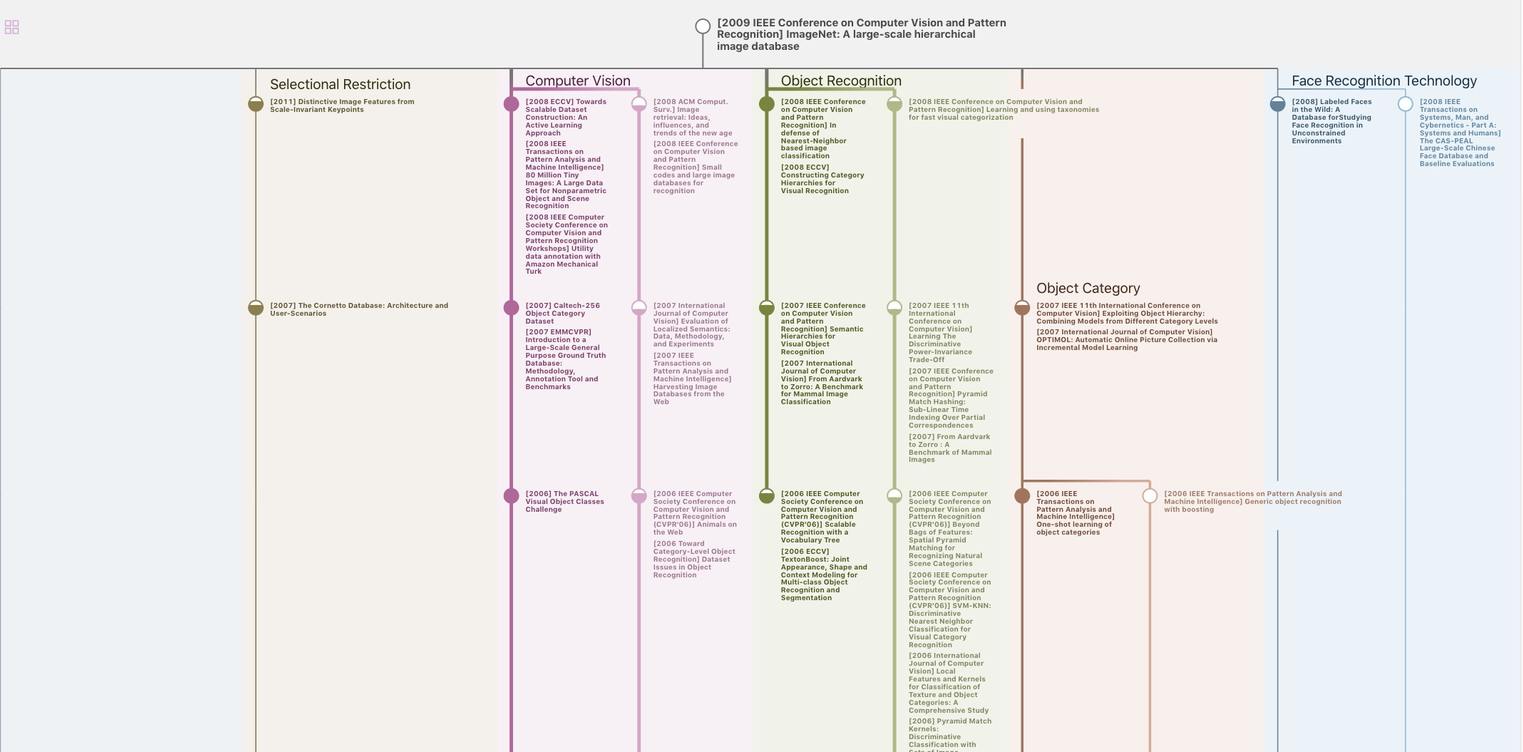
生成溯源树,研究论文发展脉络
Chat Paper
正在生成论文摘要