Structural novelty detection based on sparse autoencoders and control charts
STRUCTURAL ENGINEERING AND MECHANICS(2022)
摘要
The powerful data mapping capability of computational deep learning methods has been recently explored in academic works to develop strategies for structural health monitoring through appropriate characterization of dynamic responses. In many cases, these studies concern laboratory prototypes and finite element models to validate the proposed methodologies. Therefore, the present work aims to investigate the capability of a deep learning algorithm called Sparse Autoencoder (SAE) specifically focused on detecting structural alterations in real-case studies. The idea is to characterize the dynamic responses via SAE models and, subsequently, to detect the onset of abnormal behavior through the Shewhart T control chart, calculated with SAE extracted features. The anomaly detection approach is exemplified using data from the Z24 bridge, a classical benchmark, and data from the continuous monitoring of the San Vittore bell-tower, Italy. In both cases, the influence of temperature is also evaluated. The proposed approach achieved good performance, detecting structural changes even under temperature variations.
更多查看译文
关键词
damage detection, deep learning, machine learning, sparse autoencoder, structural health monitoring
AI 理解论文
溯源树
样例
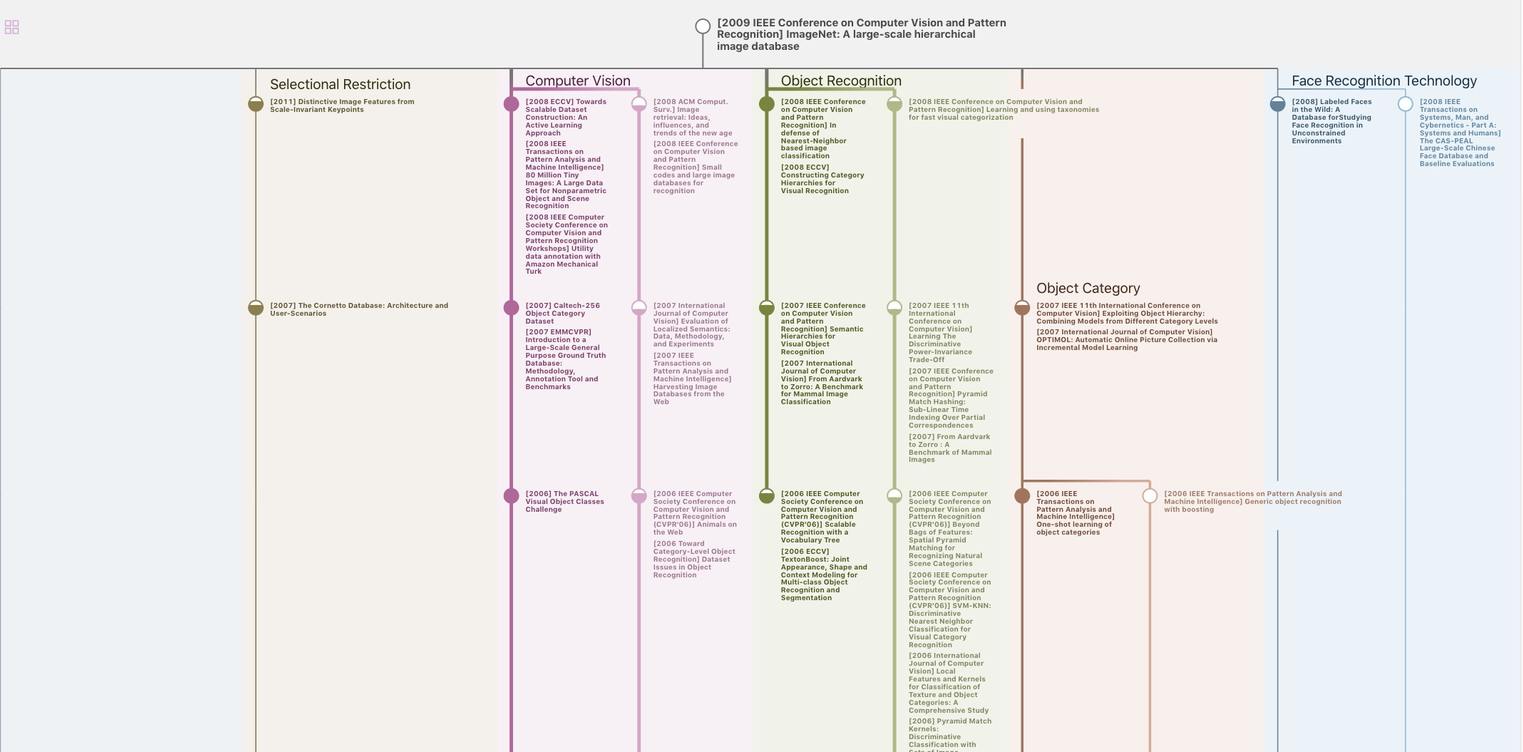
生成溯源树,研究论文发展脉络
Chat Paper
正在生成论文摘要