Supervised Attention Network for Arbitrary-Shaped Text Detection in Edge-Fainted Noisy Scene Images
IEEE TRANSACTIONS ON COMPUTATIONAL SOCIAL SYSTEMS(2023)
摘要
Text mining in noisy situations, like poor contrast and fainted edges, is one of the challenging areas of research in the domain of social networks and computer vision. Scene text detection is a complicated task as text regionhaving varying span in term of size, orientation, aspect ratio, color, font, and script. Furthermore, the contrast of a scene image varies drastically in noisy situations due to poor illumination and image filtering. This faints the text edges and make the task of detection more challenging. In this article, we bring forward a semantic edge supervised spatial-channel attention network, known as SESANet, for detecting arbitrary-shaped text instances in noisy scene images with faint text edges. Our network learns multiscale (MS) supervised edge semantic, pixel-wise spatial structure information, and interchannel dependencies for precisely localizing the text masks in scene images with poor contrast and illumination. Our network is efficient, precise, and fast in nature. SESANet captures rich, dense, discriminative, and MS semantic information. The experimental results show the success of the proposed network. It shows a superior performance with regard to recall on the publicly available benchmark datasets. A new dataset scene images, named as Edge-fainted Noisy Arbitrary-shaped Scene Text (EFNAST) dataset, having varying noise density, poor contrast, low illumination, and faint edges is created.
更多查看译文
关键词
Image edge detection, Semantics, Feature extraction, Noise measurement, Detectors, Task analysis, Lighting, Deep neural networks, fainted edges, multiattention networks, scene text detection
AI 理解论文
溯源树
样例
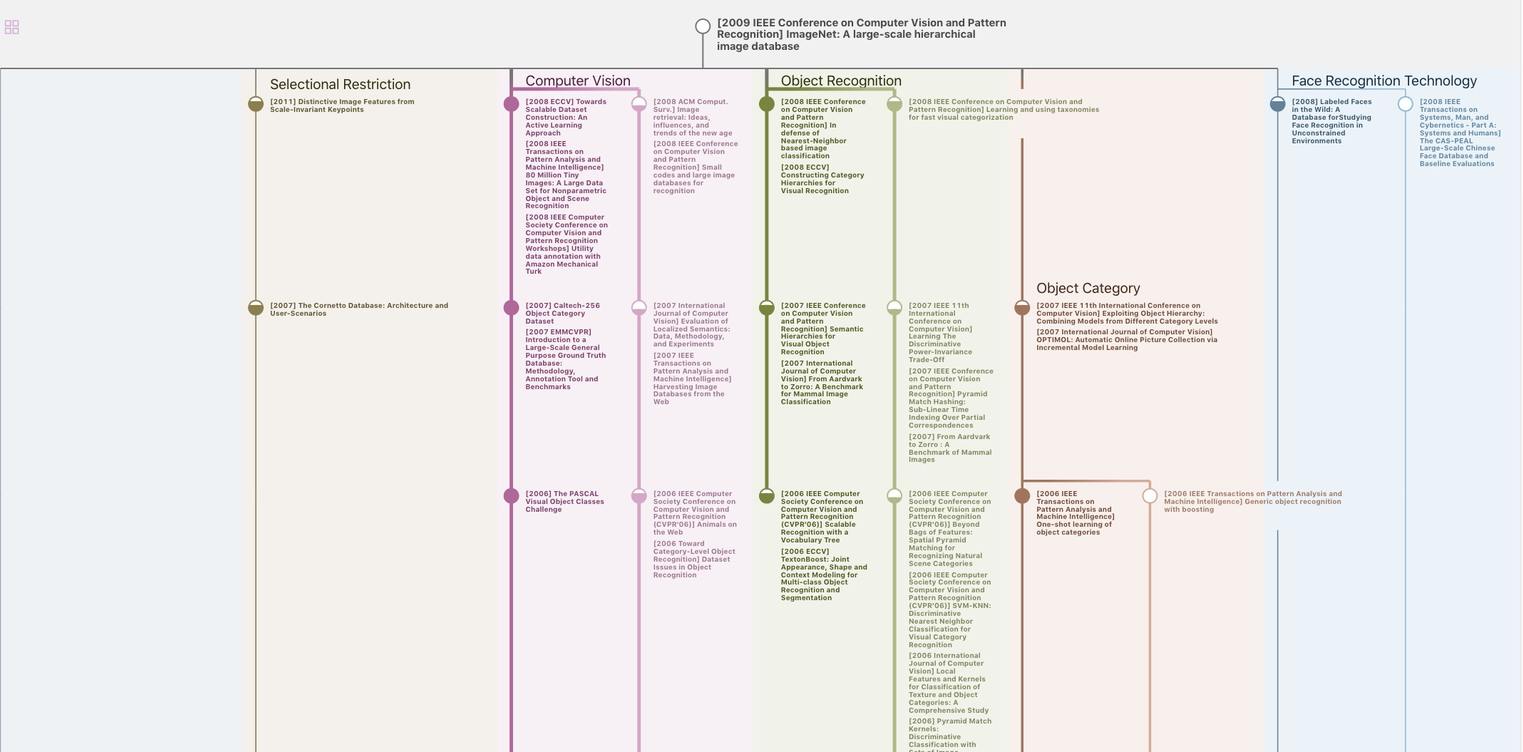
生成溯源树,研究论文发展脉络
Chat Paper
正在生成论文摘要