Large-sample properties of unsupervised estimation of the linear discriminant using projection pursuit
ELECTRONIC JOURNAL OF STATISTICS(2021)
摘要
We study the estimation of the linear discriminant with projection pursuit, a method that is unsupervised in the sense that it does not use the class labels in the estimation. Our viewpoint is asymptotic and, as our main contribution, we derive central limit theorems for estimators based on three different projection indices, skewness, kurtosis, and their convex combination. The results show that in each case the limiting covariance matrix is proportional to that of linear discriminant analysis (LDA), a supervised estimator of the discriminant. An extensive comparative study between the asymptotic variances reveals that projection pursuit gets arbitrarily close in efficiency to LDA when the distance between the groups is large enough and their proportions are reasonably balanced. Additionally, we show that consistent unsupervised estimation of the linear discriminant can be achieved also in high-dimensional regimes where the dimension grows at a suitable rate to the sample size, for example, pn = o(n(1/3)) is sufficient under skewness-based projection pursuit. We conclude with a real data example and a simulation study investigating the validity of the obtained asymptotic formulas for finite samples.
更多查看译文
关键词
Clustering, Kurtosis, Skewness, Linear discriminant analysis, Projection pursuit
AI 理解论文
溯源树
样例
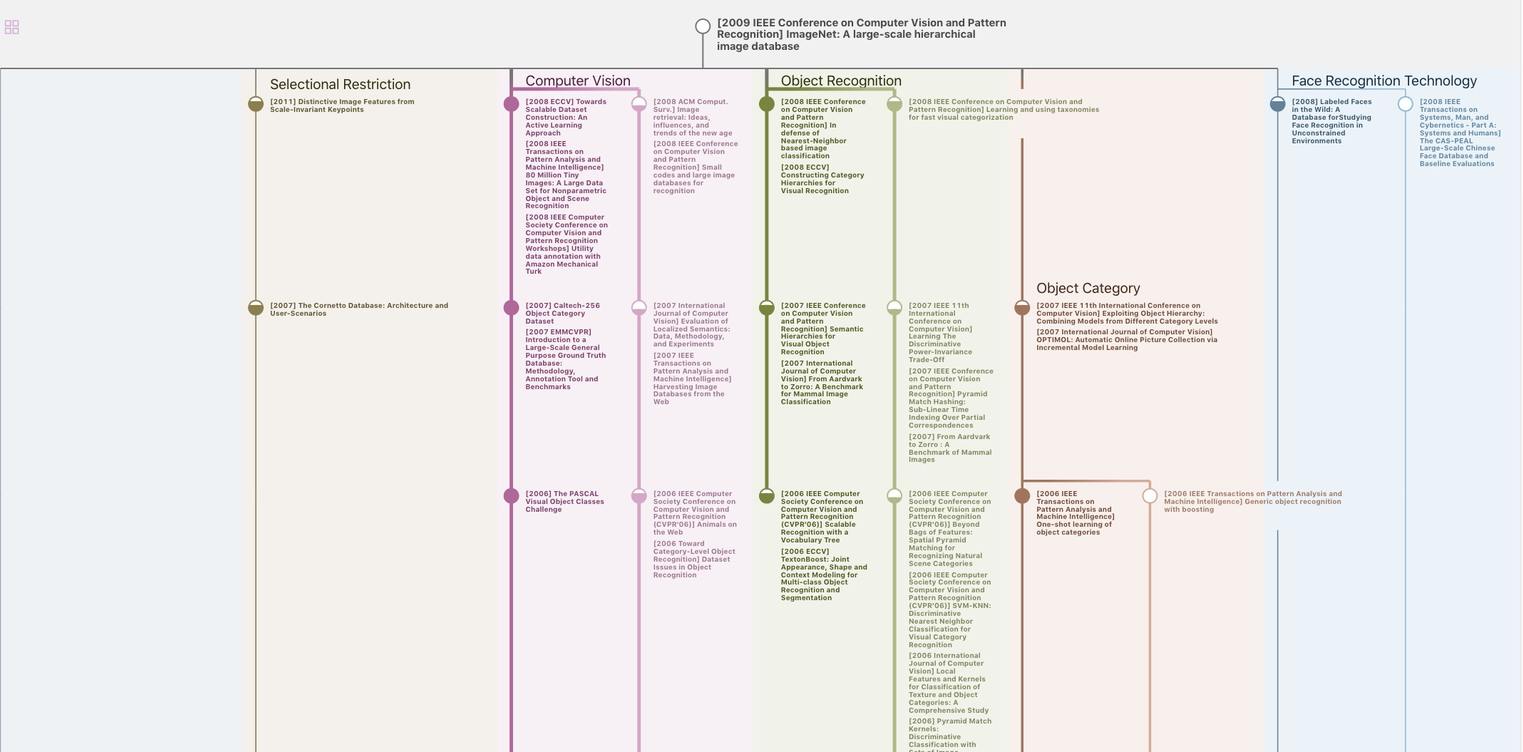
生成溯源树,研究论文发展脉络
Chat Paper
正在生成论文摘要